Multivariate Time Series Early Classification Across Channel and Time Dimensions
CoRR(2023)
摘要
Nowadays, the deployment of deep learning models on edge devices for addressing real-world classification problems is becoming more prevalent. Moreover, there is a growing popularity in the approach of early classification, a technique that involves classifying the input data after observing only an early portion of it, aiming to achieve reduced communication and computation requirements, which are crucial parameters in edge intelligence environments. While early classification in the field of time series analysis has been broadly researched, existing solutions for multivariate time series problems primarily focus on early classification along the temporal dimension, treating the multiple input channels in a collective manner. In this study, we propose a more flexible early classification pipeline that offers a more granular consideration of input channels and extends the early classification paradigm to the channel dimension. To implement this method, we utilize reinforcement learning techniques and introduce constraints to ensure the feasibility and practicality of our objective. To validate its effectiveness, we conduct experiments using synthetic data and we also evaluate its performance on real datasets. The comprehensive results from our experiments demonstrate that, for multiple datasets, our method can enhance the early classification paradigm by achieving improved accuracy for equal input utilization.
更多查看译文
关键词
classification,channel
AI 理解论文
溯源树
样例
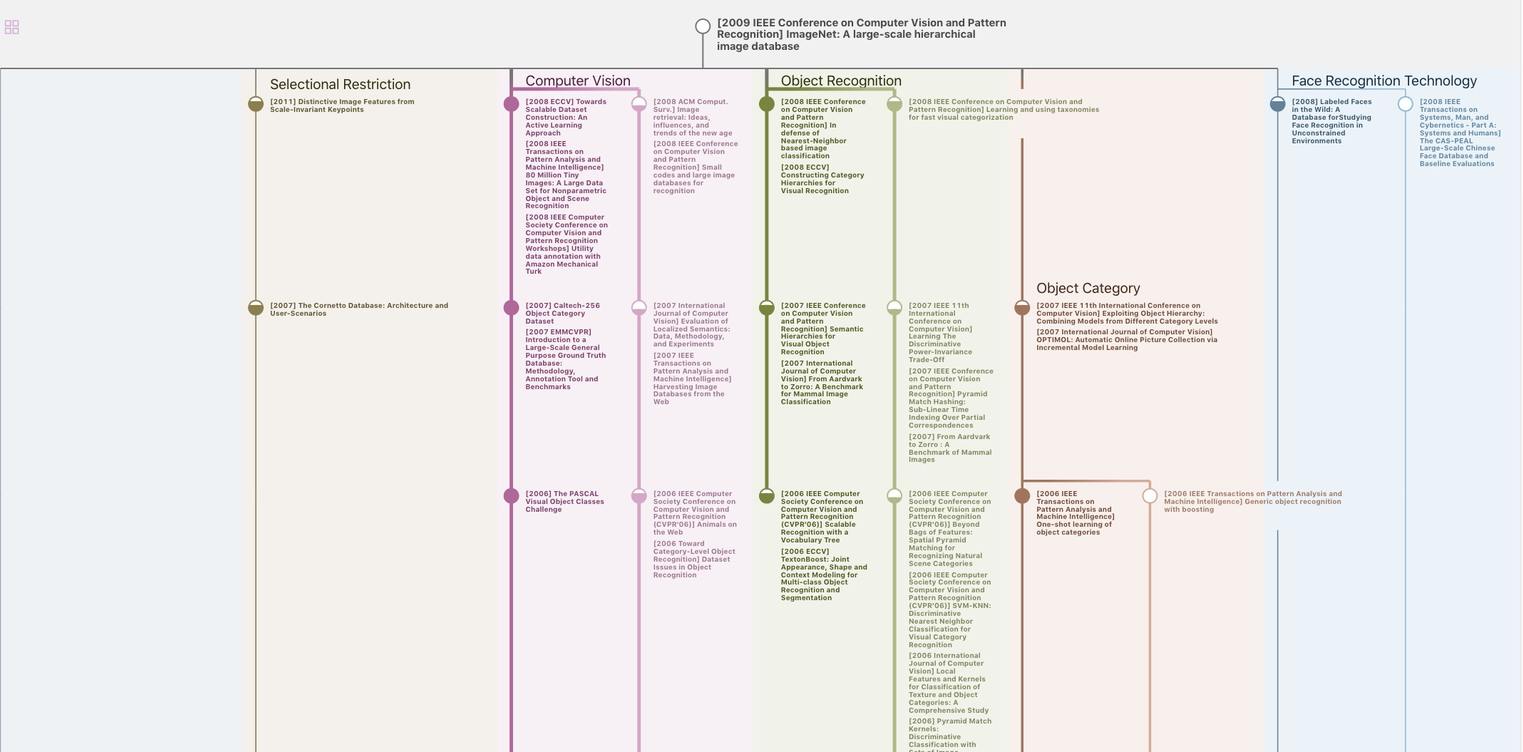
生成溯源树,研究论文发展脉络
Chat Paper
正在生成论文摘要