Surface defect detection and classification of steel using an efficient Swin Transformer
Advanced Engineering Informatics(2023)
摘要
Detecting steel-surface defects is a crucial phase in steel manufacturing; however, accurately completing the detection task is challenging. The Swin Transformer, a self-attention-based model, has shown strong performance in the field of computer vision to enhance the adaptability of the Swin Transformer to the task of steel-surface defect detection, and a new network architecture called the LSwin Transformer is proposed in this study. First, in the downsampling process, we propose a convolutional embedding module and an attention patch merging module, which simultaneously strengthen the connections between the feature map channels, reduce the resolution, and increase image information retention. Second, we propose an effective window shift strategy and a convenient computation approach to make a complete defect between patches have more opportunity to obtain interactive computing. Finally, to combine the feature extraction capability of convolutional neural networks with the global dependency building capability of the Swin Transformer, we propose a depth multilayer perceptron module. Numerous experiments were conducted on a steel-surface defect dataset. The results demonstrated that the detection effect of our model outperformed competing methods, with a mean average precision of 81.2 %. In the ablation study, we verified the effectiveness of each module and initialized the parameters of the model through transfer learning to accelerate the convergence of the model. Therefore, the proposed LSwin Transformer has significant potential for detecting steel-surface defects.
更多查看译文
关键词
Deep learning, Steel defect detection, Improved Swin Transformer, Transfer learning
AI 理解论文
溯源树
样例
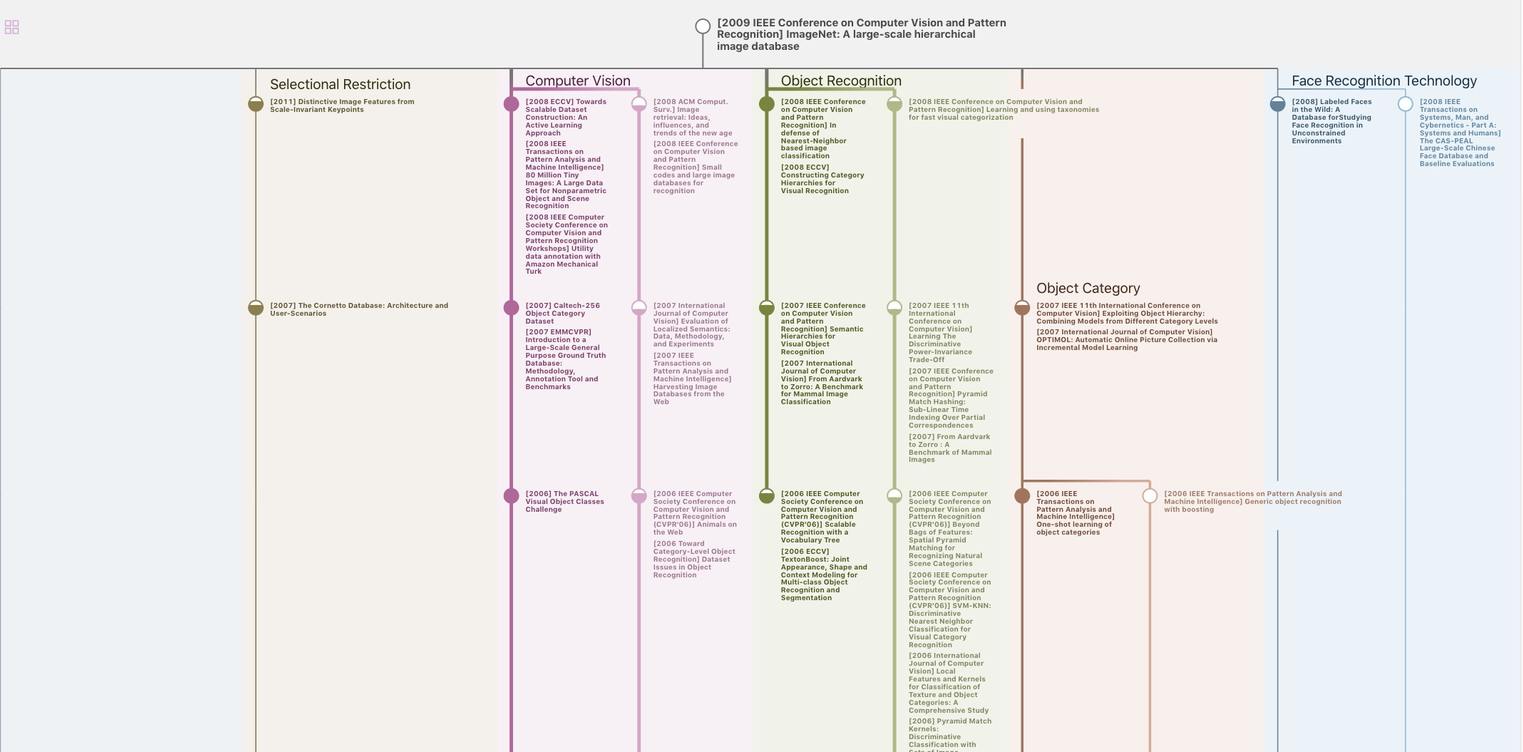
生成溯源树,研究论文发展脉络
Chat Paper
正在生成论文摘要