Energy Modelling and Forecasting for an Underground Agricultural Farm Using a Higher Order Dynamic Mode Decomposition Approach
Building Simulation Conference proceedings(2023)
摘要
This paper presents an approach based on higher order dynamic mode decomposition (HODMD) to model, analyse, and forecast energy behaviour in an urban agriculture farm situated in a retrofitted London underground tunnel, where observed measurements are influenced by noisy and occasionally transient conditions. HODMD is a data-driven reduced order modelling method typically used to analyse and predict highly noisy and complex flows in fluid dynamics or any type of complex data from dynamical systems. HODMD is a recent extension of the classical dynamic mode decomposition method (DMD), customised to handle scenarios where the spectral complexity underlying the measurement data is higher than its spatial complexity, such as is the environmental behaviour of the farm. HODMD decomposes temporal data as a linear expansion of physically-meaningful DMD-modes in a semi-automatic approach, using a time-delay embedded approach. We apply HODMD to three seasonal scenarios using real data measured by sensors located at at the cross-sectional centre of the the underground farm. Through the study we revealed three physically-interpretable mode pairs that govern the environmental behaviour at the centre of the farm, consistently across environmental scenarios. Subsequently, we demonstrate how we can reconstruct the fundamental structure of the observed time-series using only these modes, and forecast for three days ahead, as one, compact and interpretable reduced-order model. We find HODMD to serve as a robust, semi-automatic modelling alternative for predictive modelling in Digital Twins.
更多查看译文
关键词
Load Forecasting,Condition Monitoring,Short-Term Forecasting,Electricity Price Forecasting,Empirical Mode Decomposition
AI 理解论文
溯源树
样例
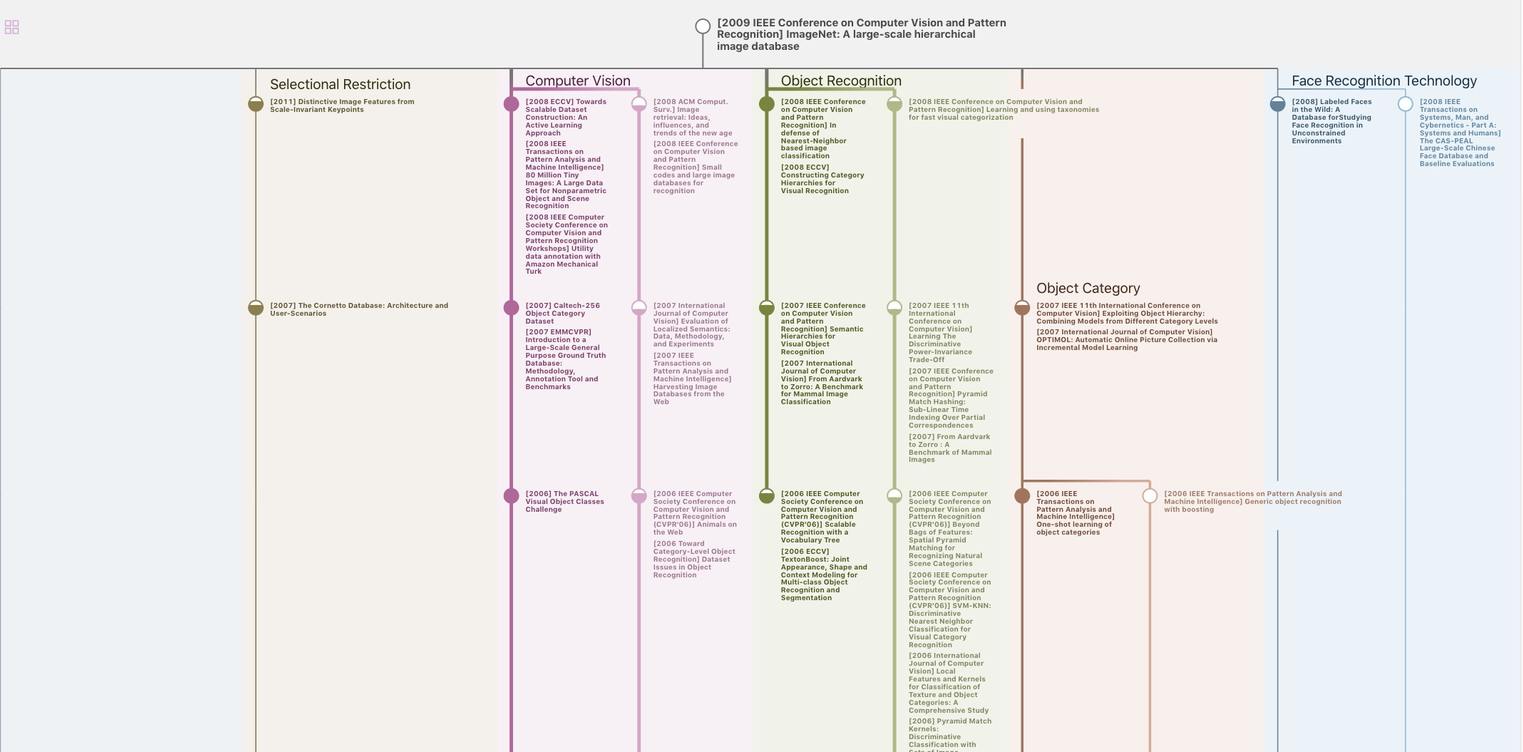
生成溯源树,研究论文发展脉络
Chat Paper
正在生成论文摘要