Can Pretrained Language Models Derive Correct Semantics from Corrupt Subwords under Noise?
CoRR(2023)
摘要
For Pretrained Language Models (PLMs), their susceptibility to noise has recently been linked to subword segmentation. However, it is unclear which aspects of segmentation affect their understanding. This study assesses the robustness of PLMs against various disrupted segmentation caused by noise. An evaluation framework for subword segmentation, named Contrastive Lexical Semantic (CoLeS) probe, is proposed. It provides a systematic categorization of segmentation corruption under noise and evaluation protocols by generating contrastive datasets with canonical-noisy word pairs. Experimental results indicate that PLMs are unable to accurately compute word meanings if the noise introduces completely different subwords, small subword fragments, or a large number of additional subwords, particularly when they are inserted within other subwords.
更多查看译文
关键词
subwords,language,noise
AI 理解论文
溯源树
样例
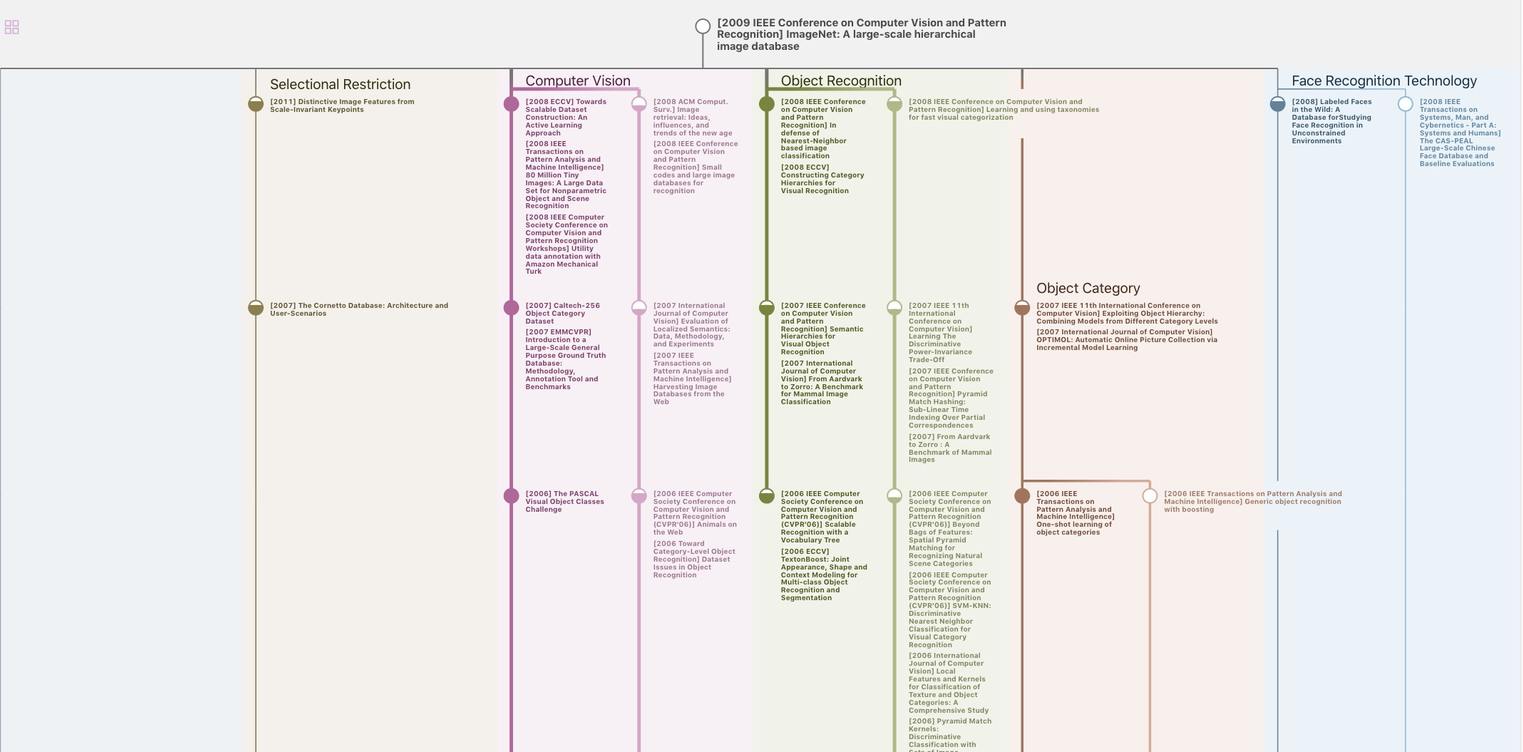
生成溯源树,研究论文发展脉络
Chat Paper
正在生成论文摘要