Assessing Dataset Quality Through Decision Tree Characteristics in Autoencoder-Processed Spaces
CoRR(2023)
摘要
In this paper, we delve into the critical aspect of dataset quality assessment in machine learning classification tasks. Leveraging a variety of nine distinct datasets, each crafted for classification tasks with varying complexity levels, we illustrate the profound impact of dataset quality on model training and performance. We further introduce two additional datasets designed to represent specific data conditions - one maximizing entropy and the other demonstrating high redundancy. Our findings underscore the importance of appropriate feature selection, adequate data volume, and data quality in achieving high-performing machine learning models. To aid researchers and practitioners, we propose a comprehensive framework for dataset quality assessment, which can help evaluate if the dataset at hand is sufficient and of the required quality for specific tasks. This research offers valuable insights into data assessment practices, contributing to the development of more accurate and robust machine learning models.
更多查看译文
关键词
dataset quality,decision tree characteristics,spaces,autoencoder-processed
AI 理解论文
溯源树
样例
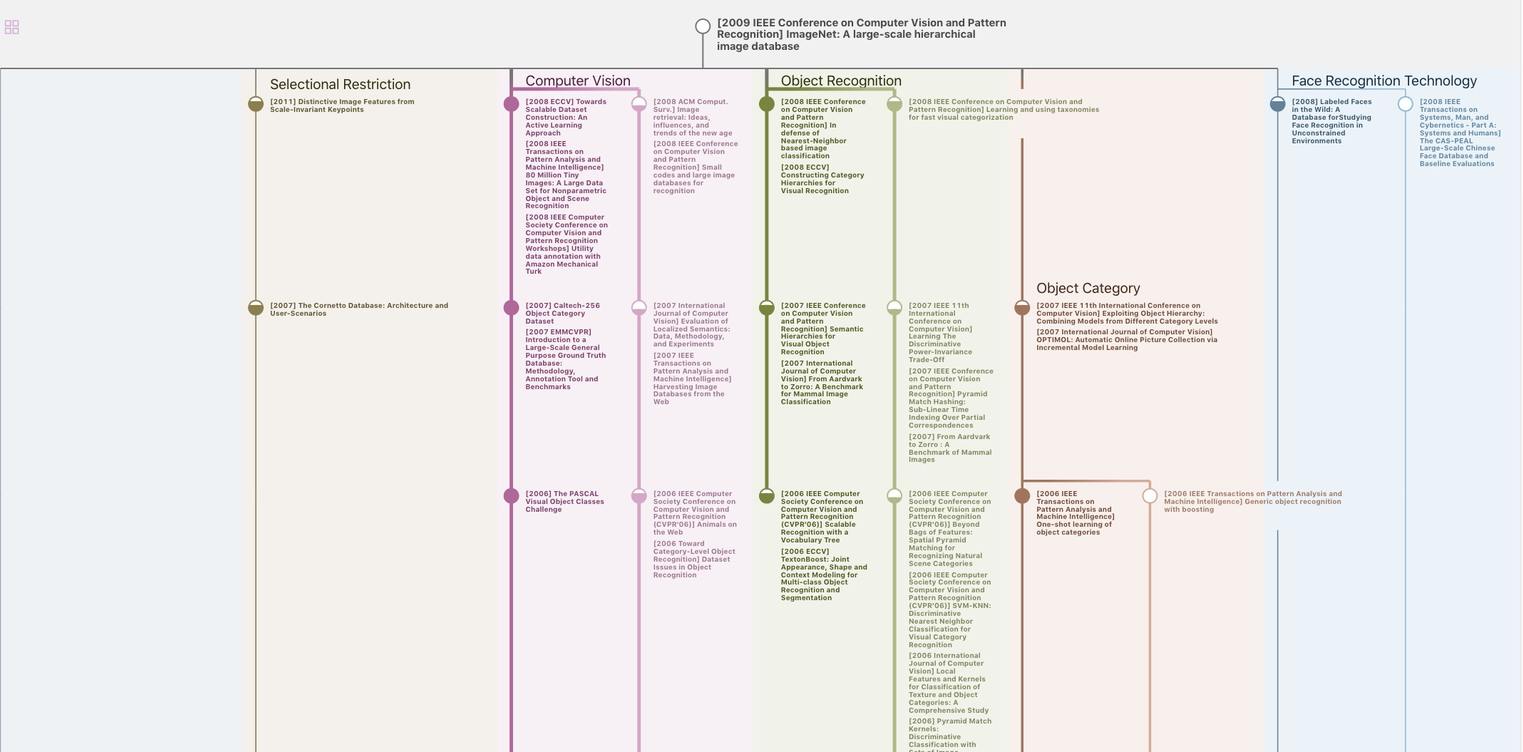
生成溯源树,研究论文发展脉络
Chat Paper
正在生成论文摘要