Fast and Automatic 3D Modeling of Antenna Structure Using CNN-LSTM Network for Efficient Data Generation
CoRR(2023)
摘要
Deep learning-assisted antenna design methods such as surrogate models have gained significant popularity in recent years due to their potential to greatly increase design efficiencies by replacing the time-consuming full-wave electromagnetic (EM) simulations. However, a large number of training data with sufficiently diverse and representative samples (antenna structure parameters, scattering properties, etc.) is mandatory for these methods to ensure good performance. Traditional antenna modeling methods relying on manual model construction and modification are time-consuming and cannot meet the requirement of efficient training data acquisition. In this study, we proposed a deep learning-assisted and image-based intelligent modeling approach for accelerating the data acquisition of antenna samples with different physical structures. Specifically, our method only needs an image of the antenna structure, usually available in scientific publications, as the input while the corresponding modeling codes (VBA language) are generated automatically. The proposed model mainly consists of two parts: Convolutional Neural Network (CNN) and Long Short Term Memory (LSTM) networks. The former is used for capturing features of antenna structure images and the latter is employed to generate the modeling codes. Through training, the proposed model can achieve fast and automatic data acquisition of antenna physical structures based on antenna images. Experiment results show that the proposed method achieves a significant speed enhancement than the manual modeling approach. This approach lays the foundation for efficient data acquisition needed to build robust surrogate models in the future.
更多查看译文
AI 理解论文
溯源树
样例
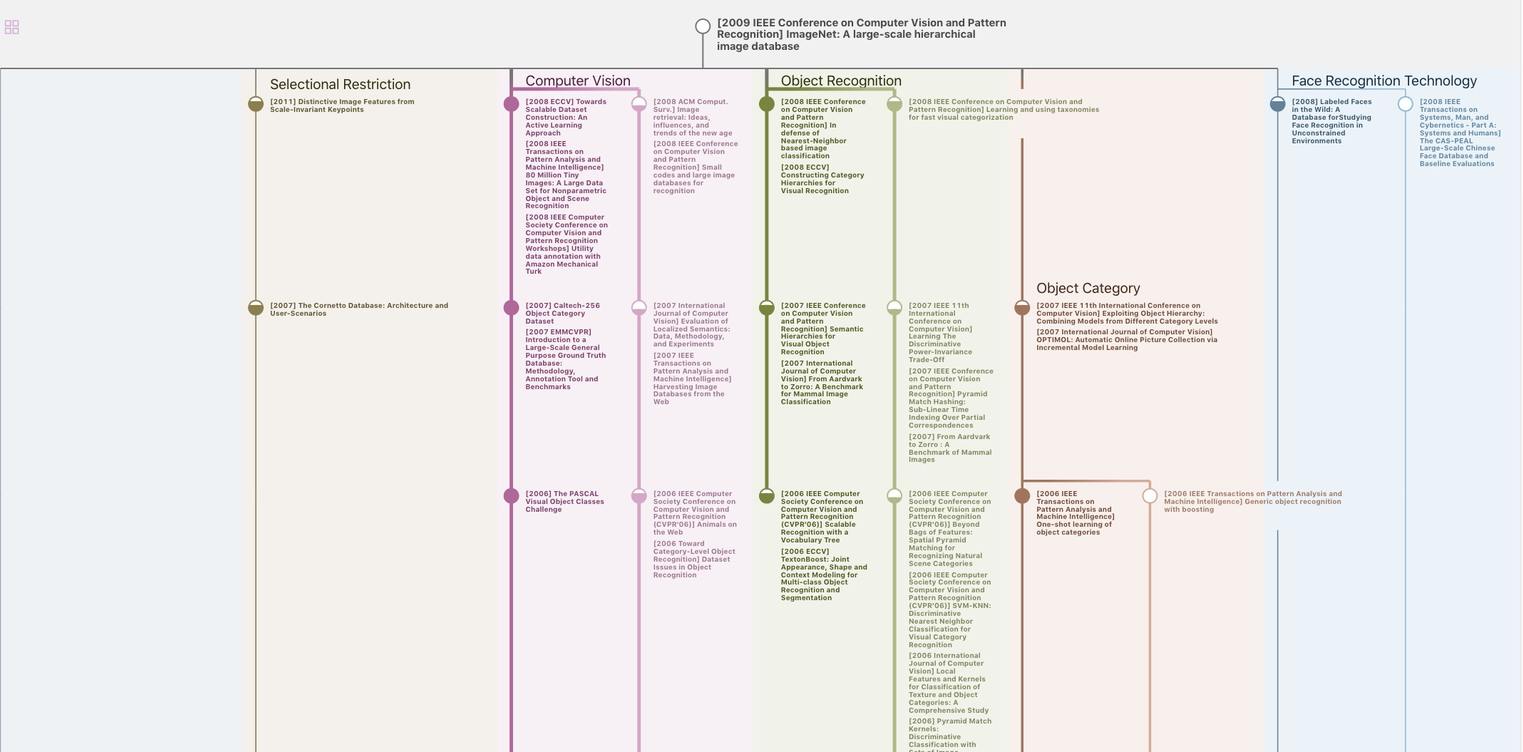
生成溯源树,研究论文发展脉络
Chat Paper
正在生成论文摘要