Super-resolution dual-layer CBCT imaging with model-guided deep learning
PHYSICS IN MEDICINE AND BIOLOGY(2024)
摘要
Objective. This study aims at investigating a novel super resolution CBCT imaging approach with a dual-layer flat panel detector (DL-FPD). Approach. With DL-FPD, the low-energy and high-energy projections acquired from the top and bottom detector layers contain over-sampled spatial information, from which super-resolution CT images can be reconstructed. A simple mathematical model is proposed to explain the signal formation procedure in DL-FPD, and a dedicated recurrent neural network, named suRi-Net, is developed based upon the above imaging model to nonlinearly retrieve the high-resolution dual-energy information. Physical benchtop experiments are conducted to validate the performance of this newly developed super-resolution CBCT imaging method. Main Results. The results demonstrate that the proposed suRi-Net can accurately retrieve high spatial resolution information from the low-energy and high-energy projections of low spatial resolution. Quantitatively, the spatial resolution of the reconstructed CBCT images from the top and bottom detector layers is increased by about 45% and 54%, respectively. Significance. In the future, suRi-Net will provide a new approach to perform high spatial resolution dual-energy imaging in DL-FPD-based CBCT systems.
更多查看译文
关键词
imaging model,dual-energy imaging,high-resolution imaging,dual-layer flat panel detector
AI 理解论文
溯源树
样例
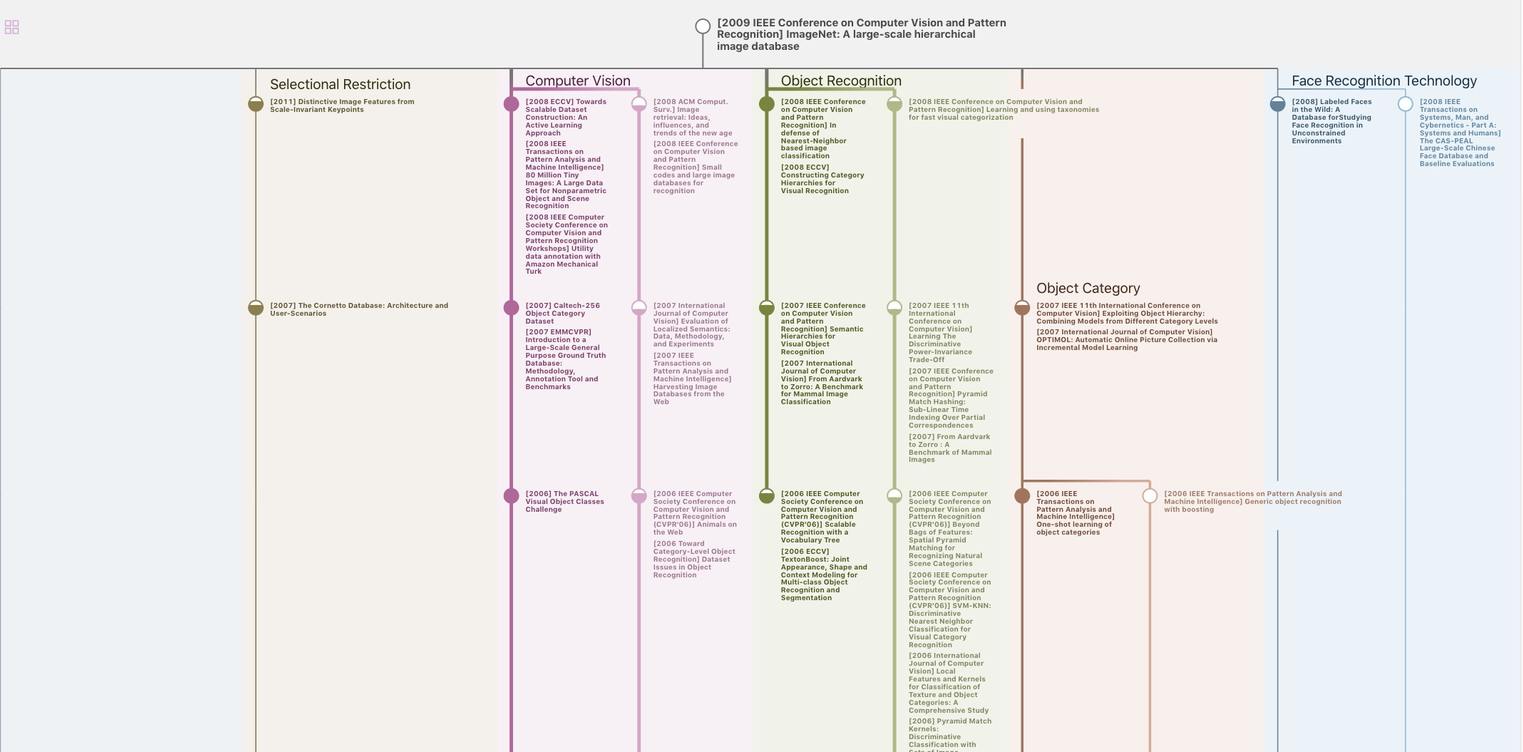
生成溯源树,研究论文发展脉络
Chat Paper
正在生成论文摘要