Bending the Automation Bias Curve: A Study of Human and AI-based Decision Making in National Security Contexts
CoRR(2023)
摘要
Uses of artificial intelligence (AI), especially those powered by machine learning approaches, are growing in sectors and societies around the world. How will AI adoption proceed, especially in the international security realm? Research on automation bias suggests that humans can often be overconfident in AI, whereas research on algorithm aversion shows that, as the stakes of a decision rise, humans become more cautious about trusting algorithms. We theorize about the relationship between background knowledge about AI, trust in AI, and how these interact with other factors to influence the probability of automation bias in the international security context. We test these in a preregistered task identification experiment across a representative sample of 9000 adults in 9 countries with varying levels of AI industries. The results strongly support the theory, especially concerning AI background knowledge. A version of the Dunning Kruger effect appears to be at play, whereby those with the lowest level of experience with AI are slightly more likely to be algorithm-averse, then automation bias occurs at lower levels of knowledge before leveling off as a respondent's AI background reaches the highest levels. Additional results show effects from the task's difficulty, overall AI trust, and whether a human or AI decision aid is described as highly competent or less competent.
更多查看译文
关键词
national security contexts,automation bias curve,ai-based
AI 理解论文
溯源树
样例
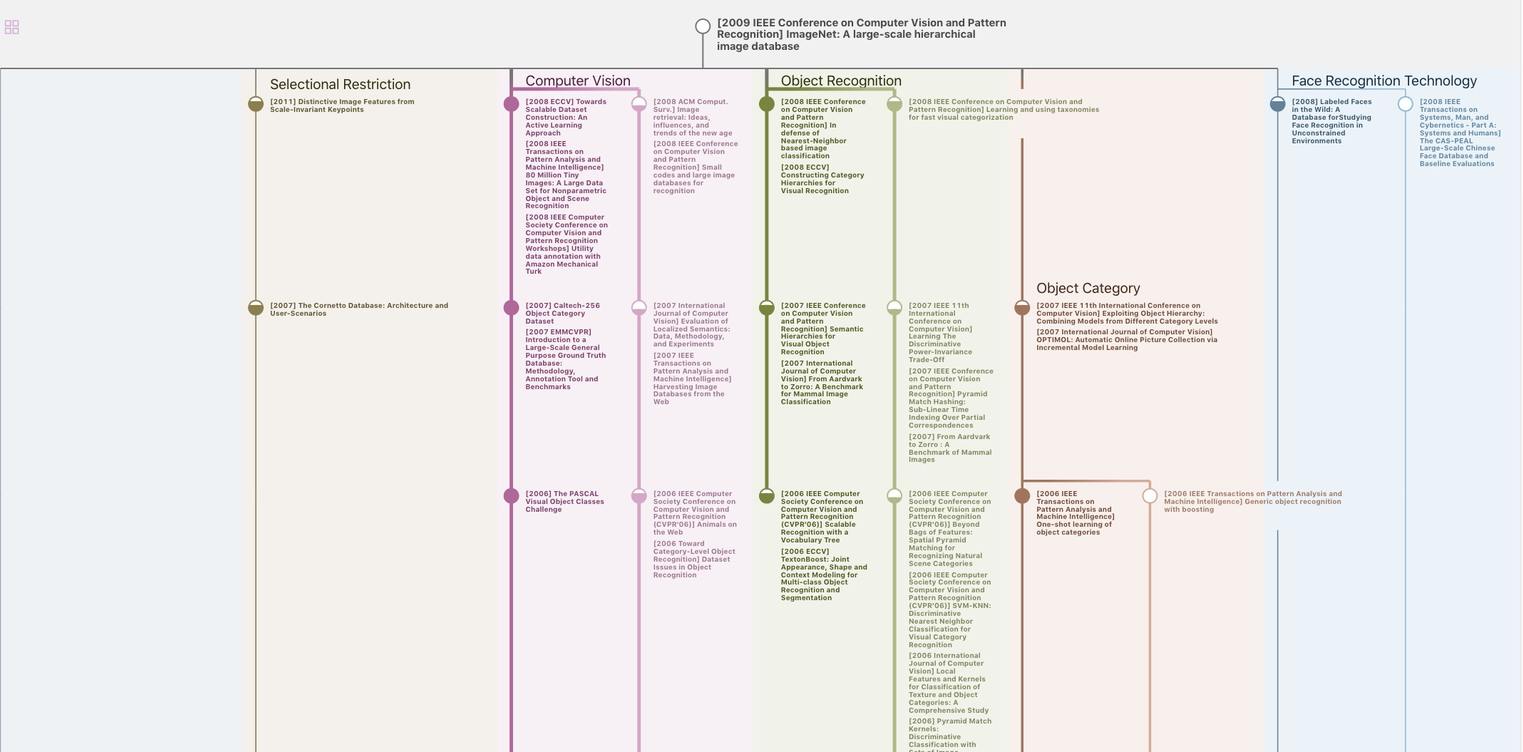
生成溯源树,研究论文发展脉络
Chat Paper
正在生成论文摘要