The Segment Anything Model (SAM) for remote sensing applications: From zero to one shot
CoRR(2023)
摘要
Segmentation is an essential step for remote sensing image processing. This study aims to advance the application of the Segment Anything Model (SAM), an innovative image segmentation model by Meta AI, in the field of remote sensing image analysis. SAM is known for its exceptional generalization capabilities and zero-shot learning, making it a promising approach to processing aerial and orbital images from diverse geographical contexts. Our exploration involved testing SAM across multi-scale datasets using various input prompts, such as bounding boxes, individual points, and text descriptors. To enhance the model's performance, we implemented a novel automated technique that combines a text-prompt-derived general example with one-shot training. This adjustment resulted in an improvement in accuracy, underscoring SAM's potential for deployment in remote sensing imagery and reducing the need for manual annotation. Despite the limitations, encountered with lower spatial resolution images, SAM exhibits promising adaptability to remote sensing data analysis. We recommend future research to enhance the model's proficiency through integration with supplementary fine-tuning techniques and other networks. Furthermore, we provide the open-source code of our modifications on online repositories, encouraging further and broader adaptations of SAM to the remote sensing domain.
更多查看译文
关键词
Artificial intelligence,Image segmentation,Multi-scale datasets,Text-prompt technique
AI 理解论文
溯源树
样例
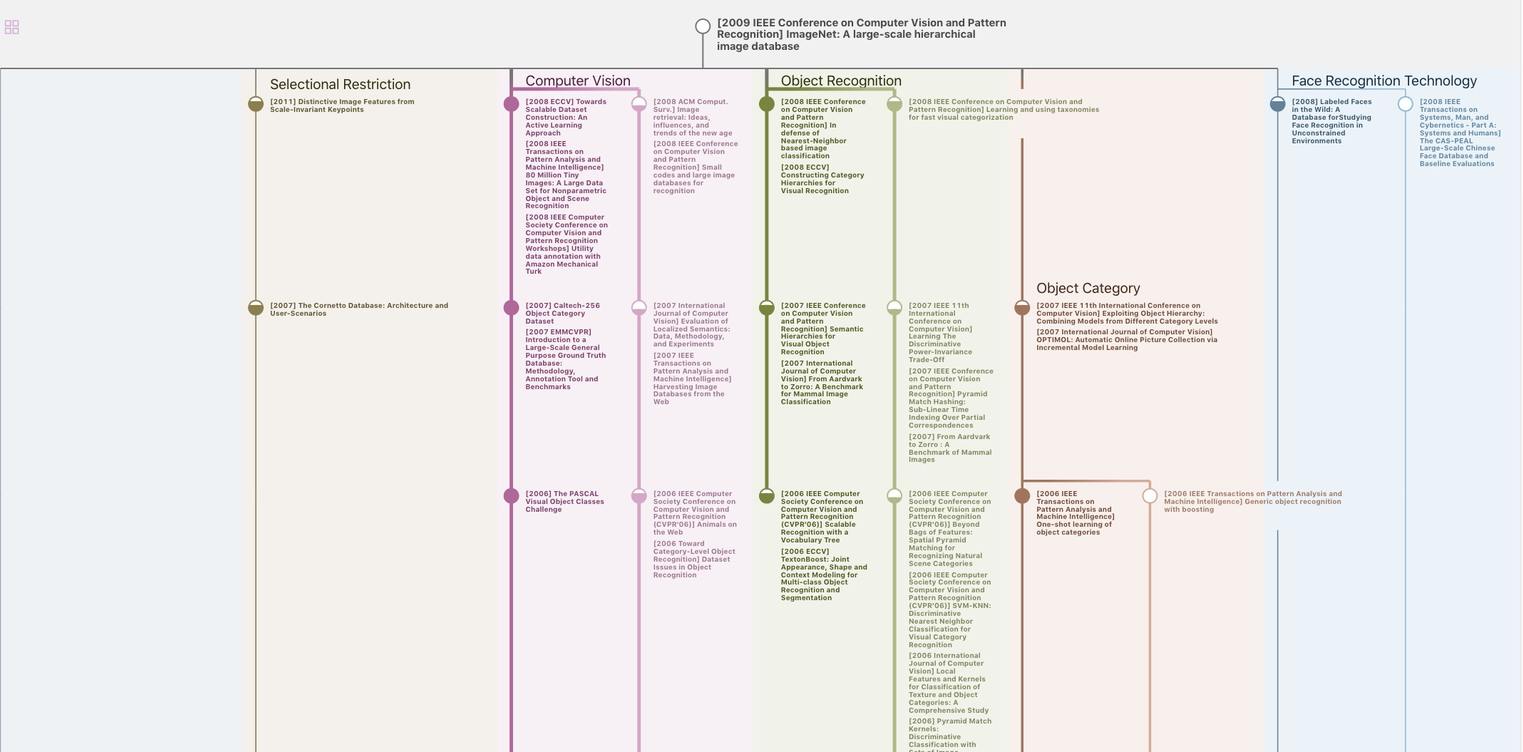
生成溯源树,研究论文发展脉络
Chat Paper
正在生成论文摘要