Integrating Randomized Placebo-Controlled Trial Data with External Controls: A Semiparametric Approach with Selective Borrowing
arXiv (Cornell University)(2023)
摘要
In recent years, real-world external controls (ECs) have grown in popularity as a tool to empower randomized placebo-controlled trials (RPCTs), particularly in rare diseases or cases where balanced randomization is unethical or impractical. However, as ECs are not always comparable to the RPCTs, direct borrowing ECs without scrutiny may heavily bias the treatment effect estimator. Our paper proposes a data-adaptive integrative framework capable of preventing unknown biases of ECs. The adaptive nature is achieved by dynamically sorting out a set of comparable ECs via bias penalization. Our proposed method can simultaneously achieve (a) the semiparametric efficiency bound when the ECs are comparable and (b) selective borrowing that mitigates the impact of the existence of incomparable ECs. Furthermore, we establish statistical guarantees, including consistency, asymptotic distribution, and inference, providing type-I error control and good power. Extensive simulations and two real-data applications show that the proposed method leads to improved performance over the RPCT-only estimator across various bias-generating scenarios.
更多查看译文
关键词
selective borrowing,placebo-controlled
AI 理解论文
溯源树
样例
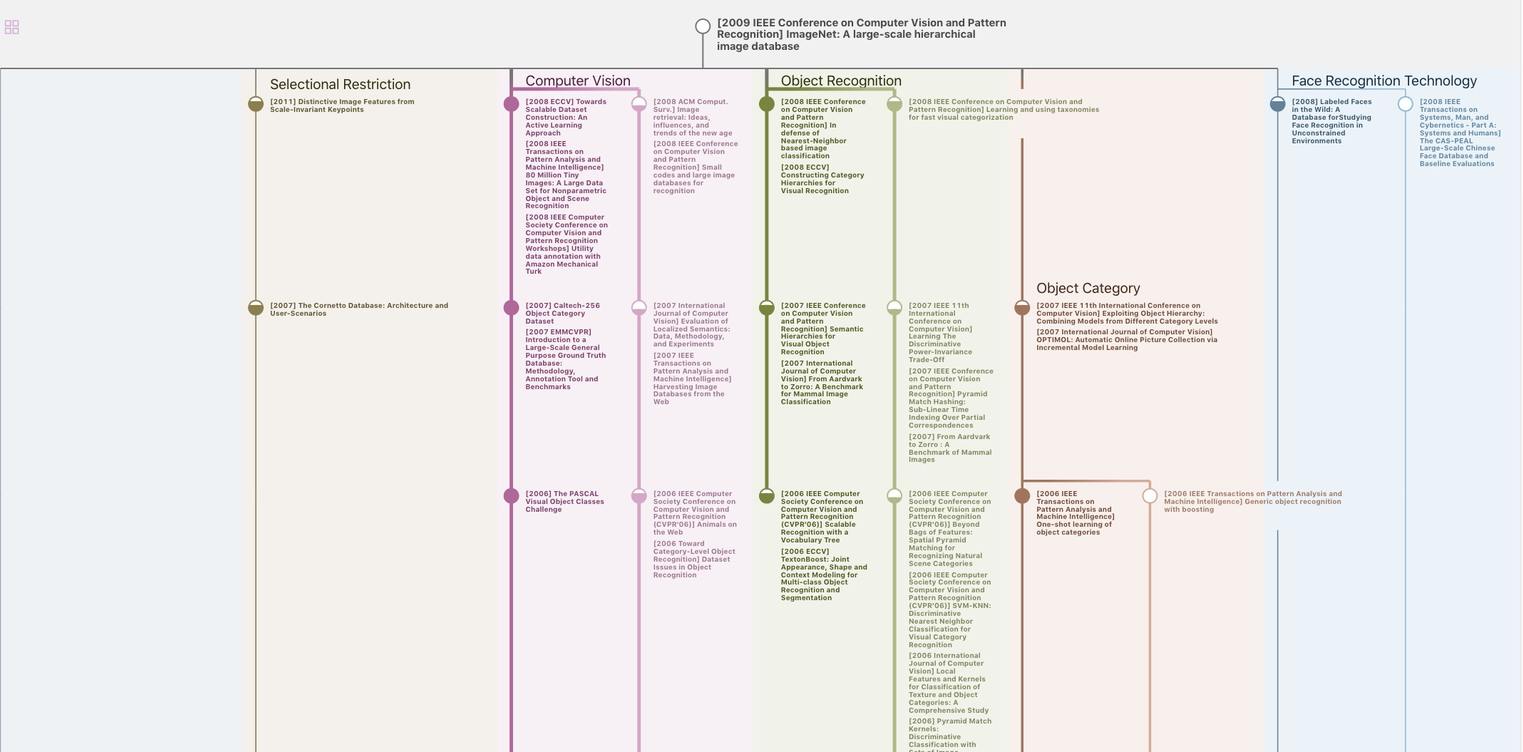
生成溯源树,研究论文发展脉络
Chat Paper
正在生成论文摘要