The Expressive Leaky Memory Neuron: an Efficient and Expressive Phenomenological Neuron Model Can Solve Long-Horizon Tasks
arXiv (Cornell University)(2023)
摘要
Biological cortical neurons are remarkably sophisticated computational
devices, temporally integrating their vast synaptic input over an intricate
dendritic tree, subject to complex, nonlinearly interacting internal biological
processes. A recent study proposed to characterize this complexity by fitting
accurate surrogate models to replicate the input-output relationship of a
detailed biophysical cortical pyramidal neuron model and discovered it needed
temporal convolutional networks (TCN) with millions of parameters. Requiring
these many parameters, however, could stem from a misalignment between the
inductive biases of the TCN and cortical neuron's computations. In light of
this, and to explore the computational implications of leaky memory units and
nonlinear dendritic processing, we introduce the Expressive Leaky Memory (ELM)
neuron model, a biologically inspired phenomenological model of a cortical
neuron. Remarkably, by exploiting such slowly decaying memory-like hidden
states and two-layered nonlinear integration of synaptic input, our ELM neuron
can accurately match the aforementioned input-output relationship with under
ten thousand trainable parameters. To further assess the computational
ramifications of our neuron design, we evaluate it on various tasks with
demanding temporal structures, including the Long Range Arena (LRA) datasets,
as well as a novel neuromorphic dataset based on the Spiking Heidelberg Digits
dataset (SHD-Adding). Leveraging a larger number of memory units with
sufficiently long timescales, and correspondingly sophisticated synaptic
integration, the ELM neuron displays substantial long-range processing
capabilities, reliably outperforming the classic Transformer or Chrono-LSTM
architectures on LRA, and even solving the Pathfinder-X task with over 70
accuracy (16k context length).
更多查看译文
关键词
Computational Neuroscience,Phenomenological Neuron Modeling,Cortical Neurons,Recurrent Neural Networks,Machine Learning,Biologically Inspired Modelling
AI 理解论文
溯源树
样例
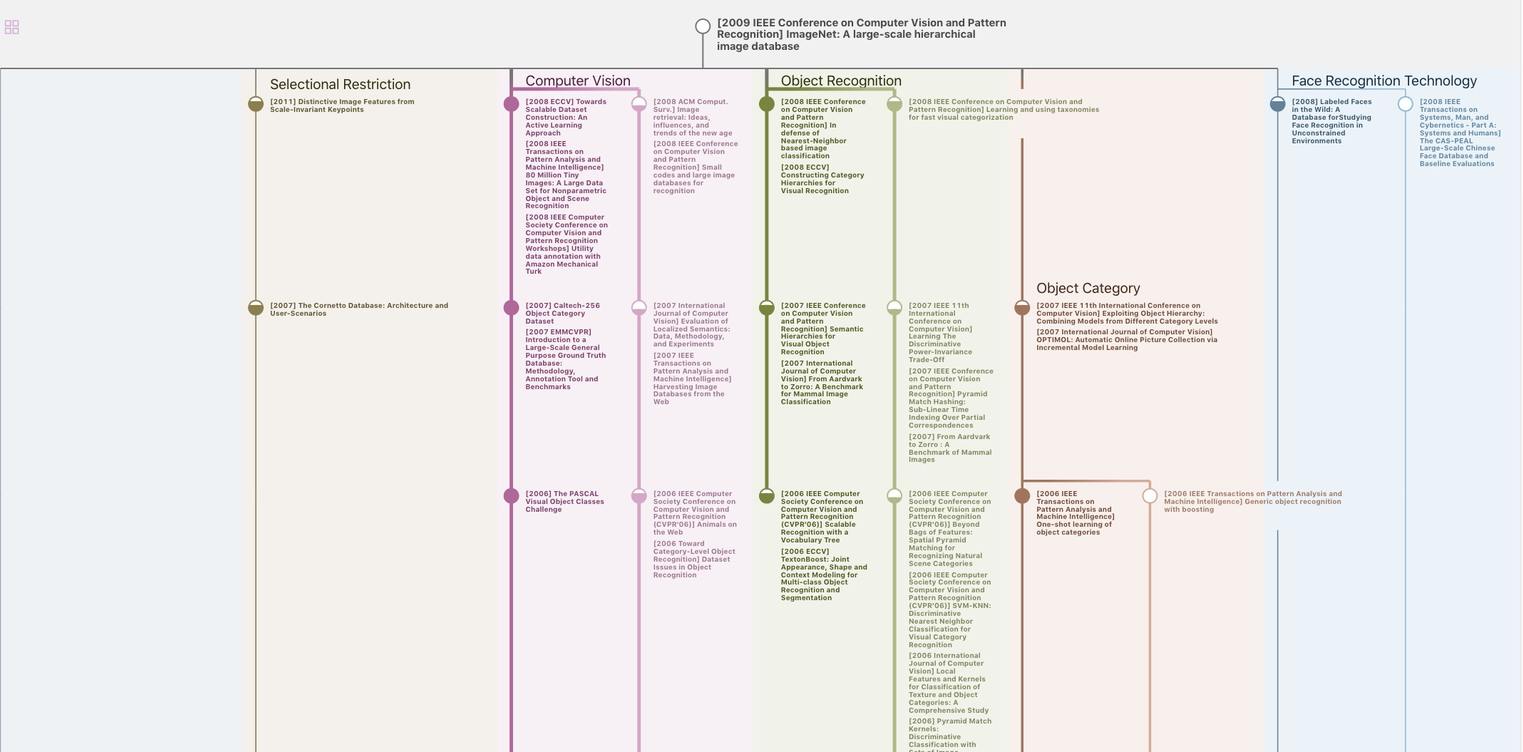
生成溯源树,研究论文发展脉络
Chat Paper
正在生成论文摘要