Model-level attention and batch-instance style normalization for federated learning on medical image segmentation
INFORMATION FUSION(2024)
摘要
Federated learning (FL) offers an effective privacy protection mechanism for cross -center medical collaboration and data sharing. In multi -site medical image segmentation, FL allows each medical site to act as a client, forming its own data domain. FL has the potential to enhance the performance of models on known domains. However, practical deployment faces the challenge of domain generalization (DG) due to the non -identical and non -independent (non-IID) nature of data from different domains. This results in decreased model performance in unseen domains. Current DG solutions are overly complex in addressing style differences and lack focus on inter -domain image features causing model differences. Furthermore, these solutions are not suitable for the FL paradigm that requires data storage separation. Hence, the lightweight model -level attention and batch -instance style normalization (MLA-BIN) is proposed to solve the DG of FL in this study. The MLA module represents the unseen domain as a linear combination of seen domain models. It does not require access to raw data but learns from the sufficient exploration of data features in known domains, thereby identifying differences in interdomain data features and enabling the global model to generalize from seen to unseen domains. In the BIN block, batch normalization (BN) and instance normalization (IN) are combined to perform the shallow layers of the segmentation network for style normalization. By integrating the segmentation backbone network with the BIN block (BIN-Net), it ensures effective learning of intra-domain features and addresses the impact of interdomain image style differences on domain generalization without accessing data from other centers. Extensive experimental results demonstrate that the proposed method achieved a Dice similarity coefficient of 88.27, 88.25 and 64.94 on the prostate, the optic disc and cup, and the COVID-19 lesion segmentation dataset, respectively, outperforming the state-of-the-art methods.
更多查看译文
关键词
Federated learning,Domain generalization,Medical image segmentation,Attention,Style normalization
AI 理解论文
溯源树
样例
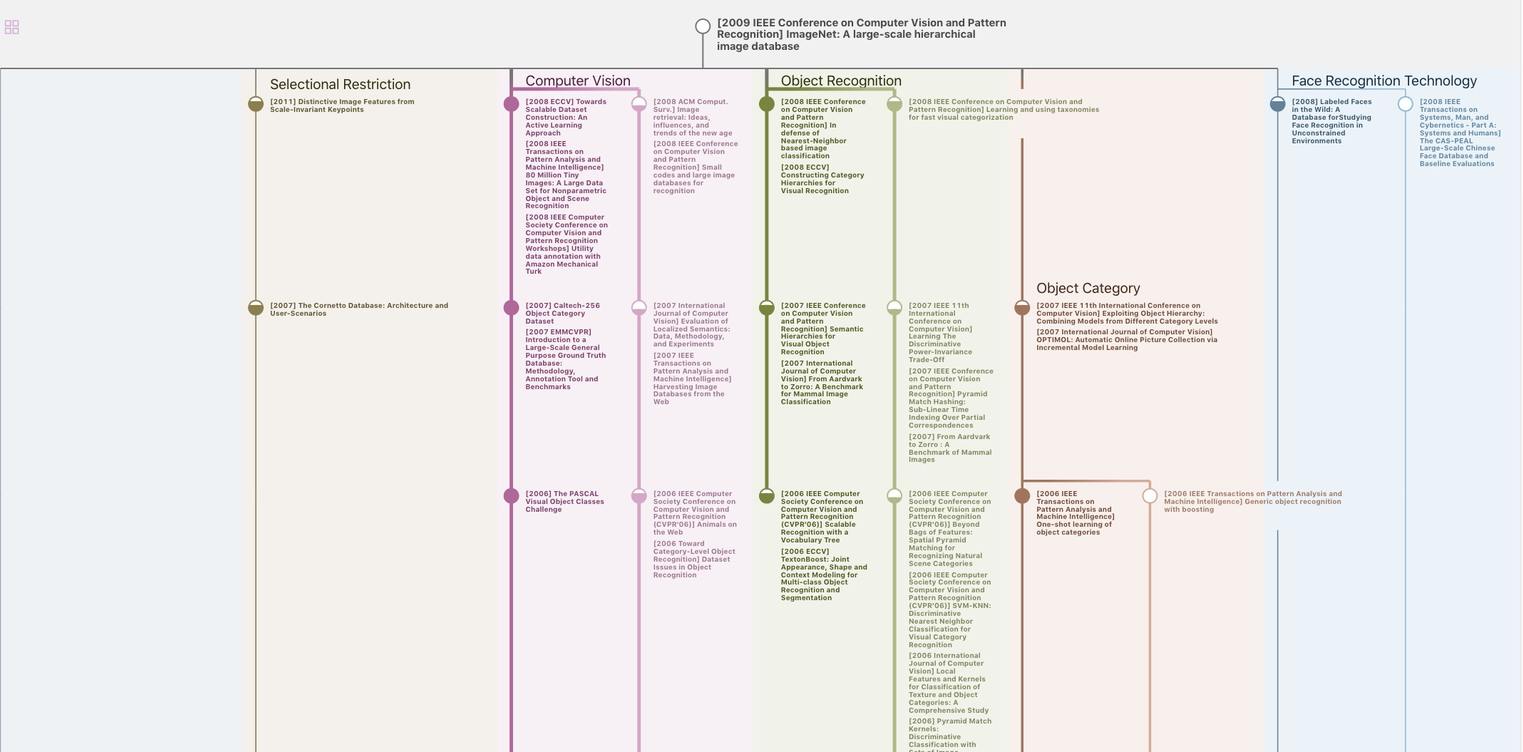
生成溯源树,研究论文发展脉络
Chat Paper
正在生成论文摘要