Deep Denoising Prior-Based Spectral Estimation for Phaseless Synthetic Aperture Radar
arXiv (Cornell University)(2023)
摘要
Incoherent processing for synthetic aperture radar (SAR) is a promising approach that enables low implementation costs, simplified hardware designs and operations in high frequency spectrum compared to the conventional imaging methods using coherent processing. Existing non-convex phaseless imaging algorithms offer recovery guarantees over limited range of forward models. In recent years, several deep learning (DL) based techniques have been introduced with the goal of extending applicability of phaseless imaging techniques to wave-based imaging modalities by addressing fundamental challenges, such as, lack of redundancy, non-uniqueness issues encountered commonly with inverse scattering models. In this paper, we introduce a DL-based phaseless SAR imaging approach that is designed under the premise that the spectral estimation technique, widely used for initializing non-convex phase retrieval algorithms, has significance far beyond generating good initial points. We extend the iterative power method for spectral estimation by using deep denoisers at each iteration, and subsequently design a deep imaging network within the plug-and-play framework. Finally, we verify the feasibility of our approach using synthetic SAR measurements.
更多查看译文
关键词
spectral estimation,radar,phaseless synthetic
AI 理解论文
溯源树
样例
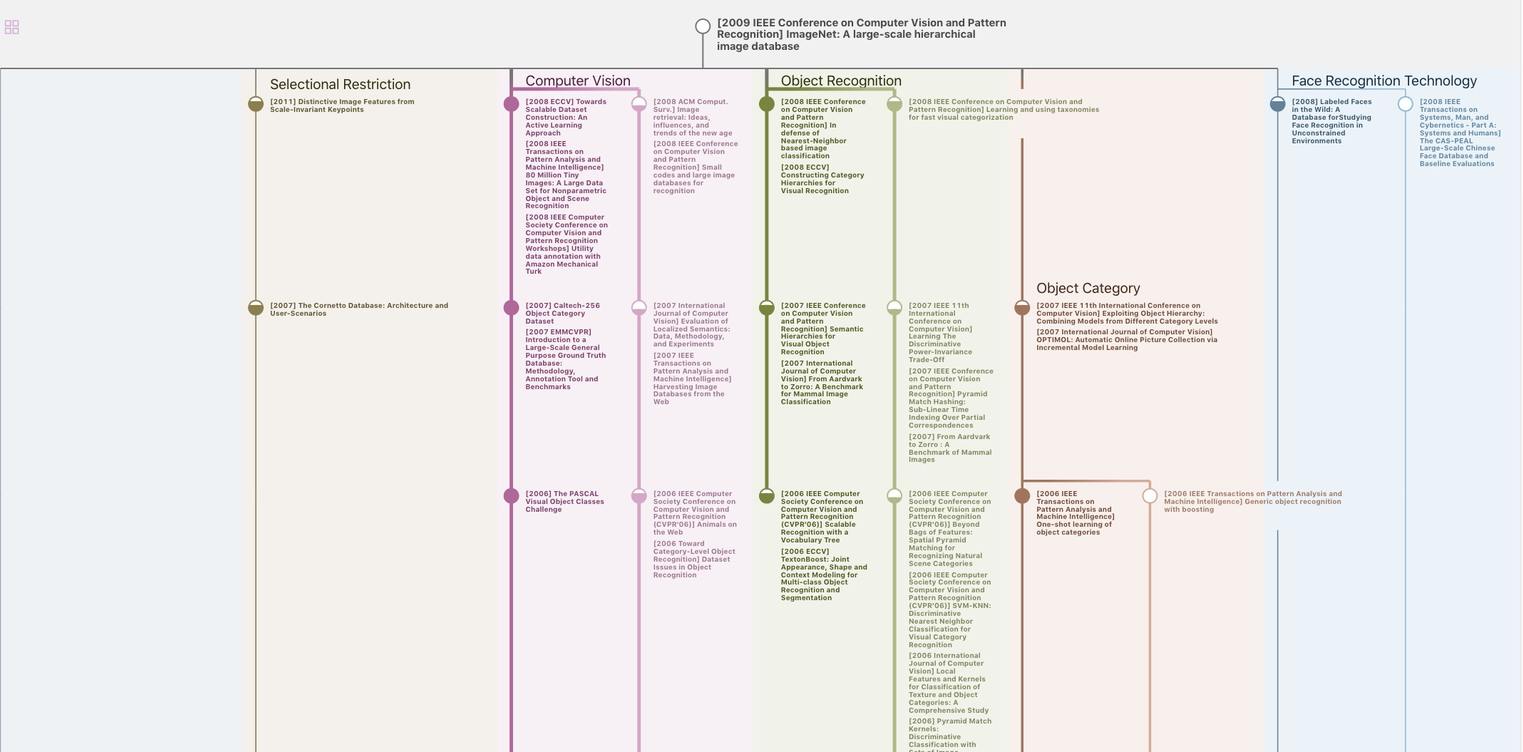
生成溯源树,研究论文发展脉络
Chat Paper
正在生成论文摘要