Graph Diffusion Kernel Maximum Correntropy Criterion Over Sensor Network and Its Performance Analysis
IEEE Sensors Journal(2023)
摘要
In recent years, graph signal processing (GSP) has attracted much attention due to its ability to model irregular and interactive data generated by wireless sensor networks (WSNs). However, there is no practical method to deal with the problem of modeling nonlinear systems in non-Gaussian noise environments. Given that the maximum correntropy criterion (MCC) exhibits robustness to impulsive and non-Gaussian noise, this article introduces it into the graph kernel adaptive filtering (KAF) algorithm and develops a graph diffusion kernel MCC (GDKMCC) algorithm. To suppress the problem of the infinite growth of the filter coefficient vector of the proposed algorithm, a pretrained dictionary (PD) method is used in this article. In addition, the mean-square transient behavior and the convergence condition of the GDKMCC-PD algorithm are also provided under some assumptions. Finally, the simulation results verify the superiority of the proposed algorithm in modeling nonlinear systems under non-Gaussian noise environments and the correctness of the theoretical model.
更多查看译文
关键词
Distributed learning,graph signal processing (GSP),kernel graph filters,maximum correntropy criterion (MCC),transient performance analysis,wireless sensor network (WSN)
AI 理解论文
溯源树
样例
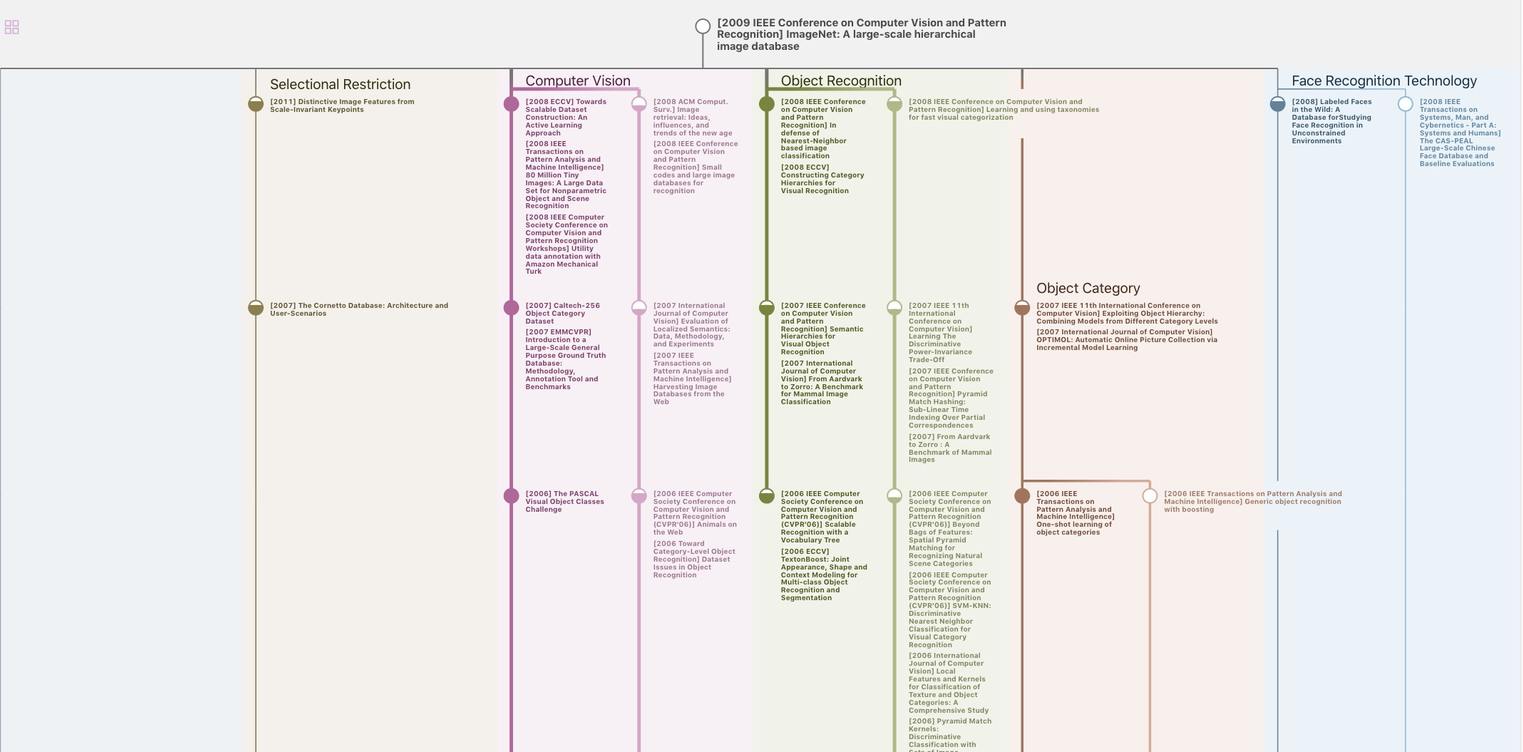
生成溯源树,研究论文发展脉络
Chat Paper
正在生成论文摘要