TG-GNN: Transformer based Geometric enhancement graph neural network for molecular property prediction
Research Square (Research Square)(2022)
摘要
Abstract Molecular properties play a crucial role in material discovery, protein interaction and drug development. The appearance of Graph Neural Network(GNN) significantly improved the performance of molecular property prediction. However, nodes in GNN only update the features of neighbor nodes, resulting in insufficient ability to encode global feature information. The self attention mechanism in transformer encodes the global information of molecules, while its spatial information is insufficient. Since molecules are three-dimensional spatial structures, spatial geometry information is an important attribute of molecules. Therefore, a transformer based geometric enhanced graph neural network (TG-GNN) is proposed to combine global and local molecule information with three-dimensional spatial structures to predict molecular properties. In this network, Graph neural network Geometric Feature Fusion Module (GGFF) and Transformer Geometric Feature Enhancement Module (TGF) are proposed to enhance the spatial geometry learning ability of the network. The GGFF module constructs a parallel graph neural network using bond and bond angle. The introduction of bond angle effectively complements the spatial information of the network. The TGF module introduces the coordinates and centrality degree features into the transformer to enhance the geometric expression ability of the module. GGFF module and TGF module encode local information and global information of molecules at the same time on the QM9 and OMDB dataset.
更多查看译文
关键词
geometric enhancement graph,molecular,neural network,tg-gnn
AI 理解论文
溯源树
样例
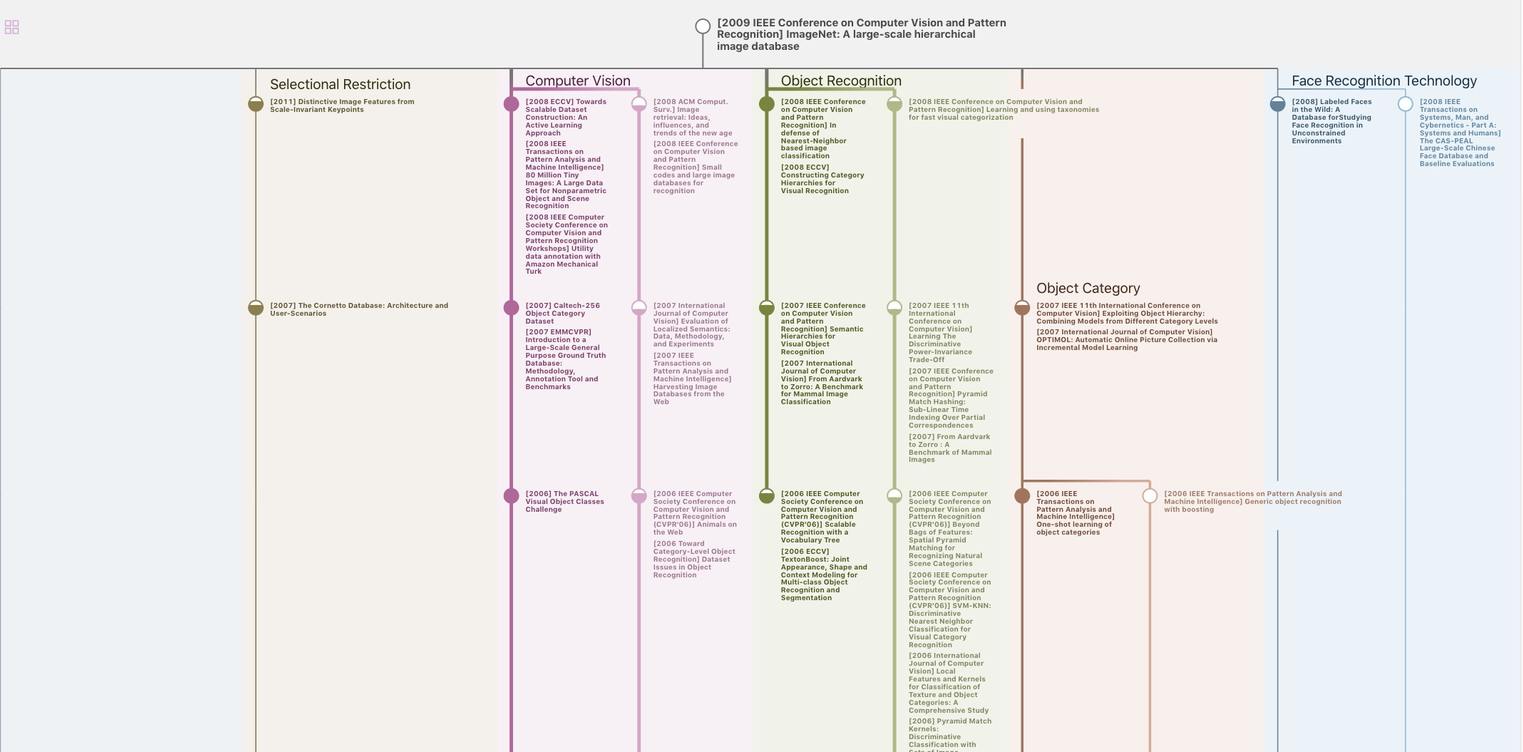
生成溯源树,研究论文发展脉络
Chat Paper
正在生成论文摘要