Radiomics-based machine learning model for predicting Overall and Progression-Free Survival in rare cancer: a case study for Primary CNS Lymphoma patients
Research Square (Research Square)(2022)
摘要
Abstract In this study, we investigated whether radiomics features can improve outcome prediction in patients with Primary Central Nervous System Lymphoma (PCNSL). 80 patients diagnosed with PCNSL were enrolled. Of these, 56 patients with complete Magnetic Resonance Imaging (MRI) series (including T1-weighted, T2-weighted, 3D-T1 with gadolinium, and FLAIR) were selected for the stratification analysis. Following radiomic feature extraction and selection, different Machine Learning (ML) models were tested for Overall Survival (OS) and Progression-free Survival (PFS) prediction, and accuracy was computed in terms of F1-score and area under ROC curve (AUC). To assess the stability of the selected features, images from 23 patients scanned at three different time points were used to compute the Interclass Correlation Coefficient (ICC) and evaluate the reproducibility of each feature for both original and normalized images. Features extracted from Z-score normalized images resulted significantly more stable (ICC=0.80) than those extracted from non-normalized images (ICC=0.58), p-value<10 −12. For the best classifier, F1-score resulted 0.83/0.77-0.86 (median/interquartile range), and 0.80/0.67-0.88, for OS and PFS respectively. On average, AUC showed that radiomics based prediction (AUC=0.86 for OS, AUC=0.84 for PFS) overcomes prediction based on current clinical prognostic factors (AUC=0.70 for OS, AUC=0.56 for PFS). These results indicate that radiomics features extracted from normalized MR images can improve prognosis stratification of PCNSL patients and pave the way for further study on its potential role to drive treatment choise.
更多查看译文
关键词
rare cancer,lymphoma,machine learning model,radiomics-based,progression-free
AI 理解论文
溯源树
样例
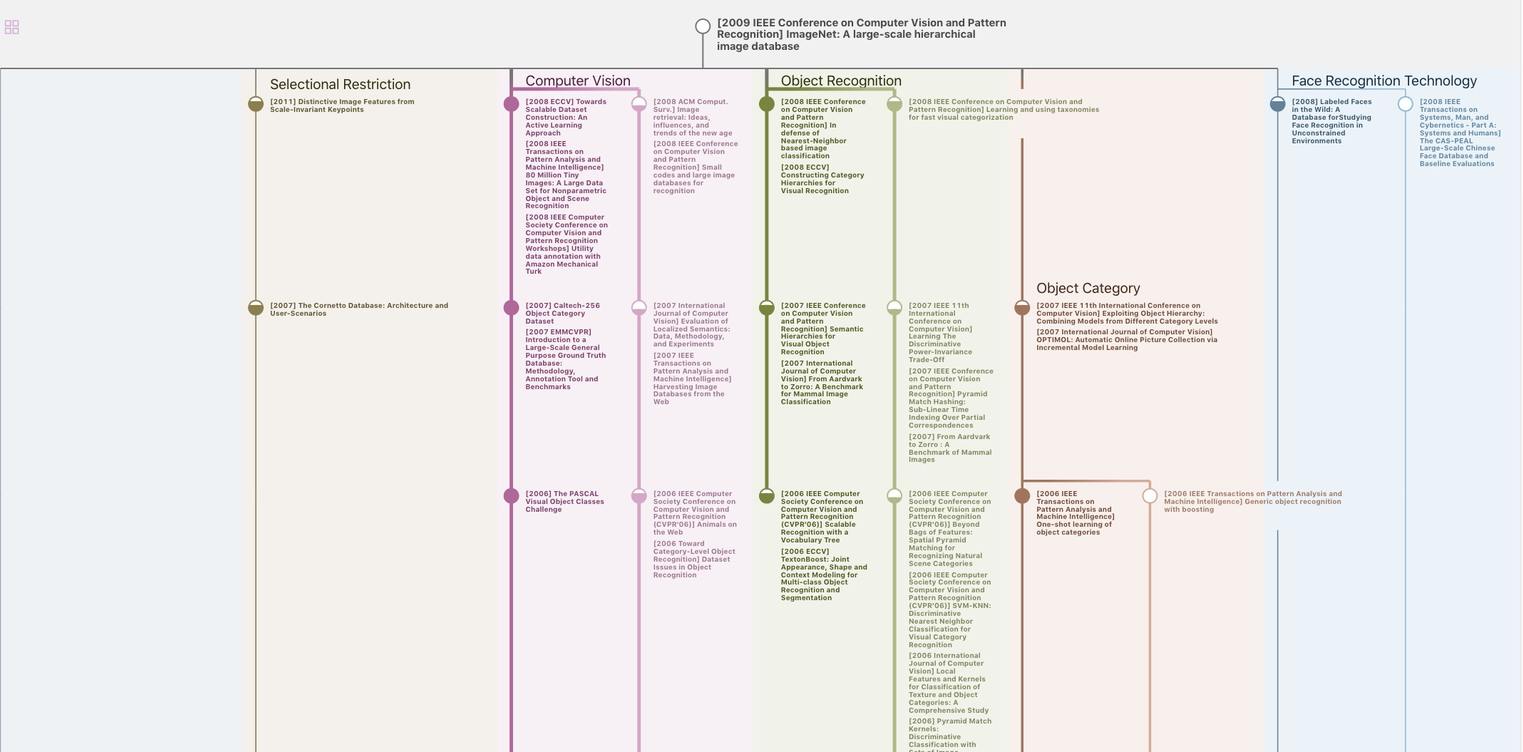
生成溯源树,研究论文发展脉络
Chat Paper
正在生成论文摘要