Unmasking the Twitter Discourses on Masks during the COVID-19 Pandemic: A User Cluster-based BERT Topic Modeling Approach (Preprint)
crossref(2022)
摘要
UNSTRUCTURED The COVID-19 pandemic has spotlighted the intertwining of politics and public health. So too, a public health tool, surveillance, can also be used to expose the political context of an event and to guide better public health interventions. In its current form, infoveillance tends to neglect identity and interest-based users. Adopting an algorithmic tool to appropriately classify short social media texts might remedy that gap. Our study advances a computational framework to cluster Twitter users based on their identities and interests as expressed through Twitter bio pages. The framework then uses BERT topic modeling to identify topics by the user clusters. By analyzing the Twitter discourse on masks and mask-wearing during COVID-19 in the English-speaking world, we show how online discourse shifted over time and varied by four user clusters: Conservative political, Progressive political, General Public, and Public Health Professionals. The political groups and general public focused on the science of mask-wearing and the partisan politics of mask policies. A populist discourse that pits citizens against elites and institutions was identified in some tweets. Politicians (such as Donald Trump) and geopolitical tensions (with regards to China) were found to drive the discourse. Our data show limited participation of public health professionals compared to other users. We conclude by discussing the importance of a priori user classification in analyzing online discourse and illustrating the fit of BERT topic modeling in identifying contextualized topics in short social media texts.
更多查看译文
AI 理解论文
溯源树
样例
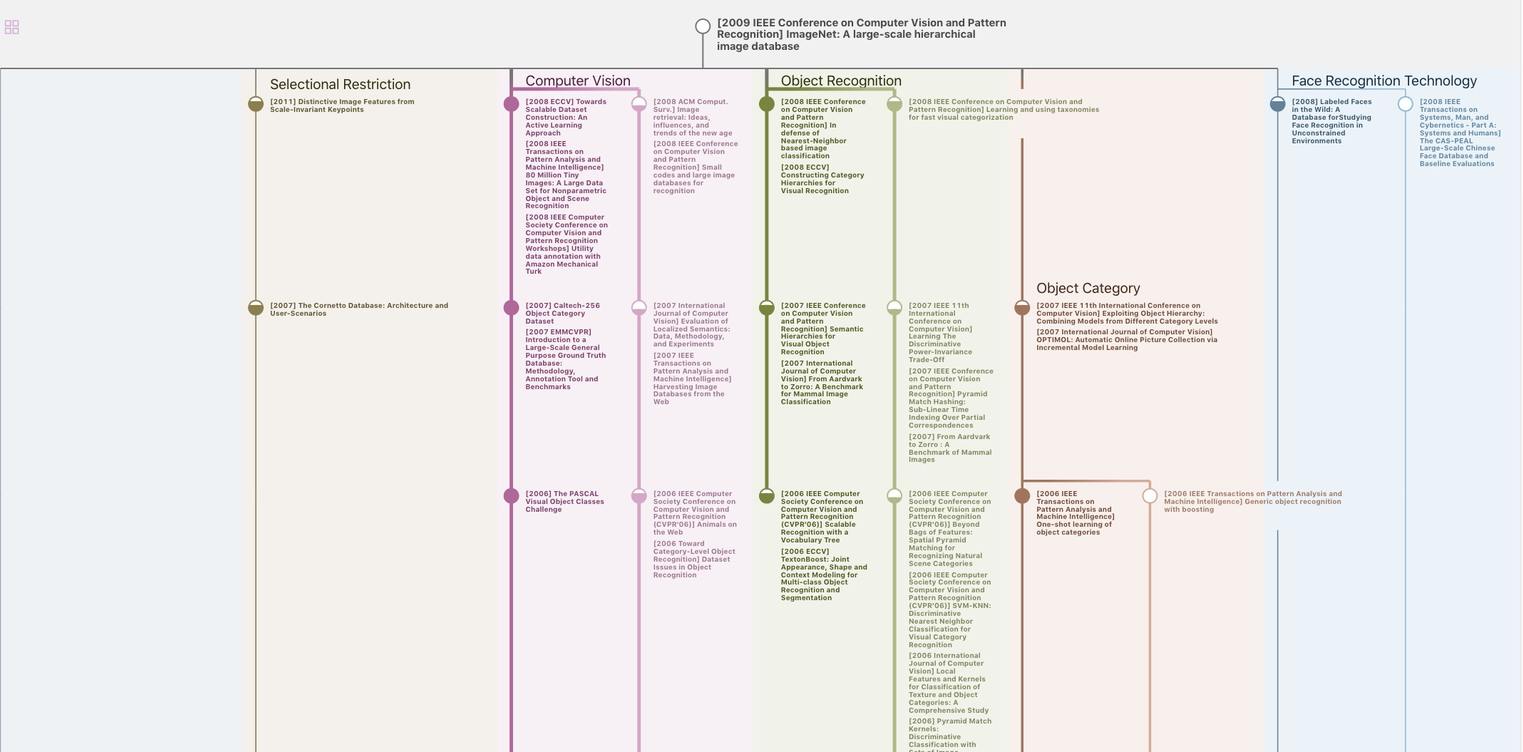
生成溯源树,研究论文发展脉络
Chat Paper
正在生成论文摘要