Using group level factor models to resolve high dimensionality in model-based sampling
crossref(2022)
摘要
Joint modelling of behaviour and neural activation poses the potential to provide significant advances in linking brain and behaviour. However, methods of joint modelling have been limited by difficulties in estimation, often due to high dimensionality and simultaneous estimation challenges. In the current article, we propose a method of model estimation which draws on current state-of-the-art Bayesian hierarchical modelling techniques and uses factor analysis as a means of dimensionality reduction to provide further information on which to make inference. The method uses a particle metropolis within Gibbs sampler (PMwG; Gunawan, Hawkins, Tran, Kohn, & Brown, 2020), where the factor structure is estimated within the Gibbs step for the group level. We show the significant dimensionality reduction gained by factor analysis in the Gibbs step of the PMwG, evidence for parameter recovery, a variety of factor loading constraints which can be used for different purposes and research questions, as well as two applications of the method to previously analysed data. This method represents a flexible and usable approach with interpretable outcomes, which relies on data driven analysis as opposed to hypothesis driven methods often used in joint modelling. Although we focus on joint modelling methods, this model based estimation approach could be used for any high dimensional modelling problem. We provide open source code and accompanying tutorial documentation to make the method accessible to any researchers.
更多查看译文
AI 理解论文
溯源树
样例
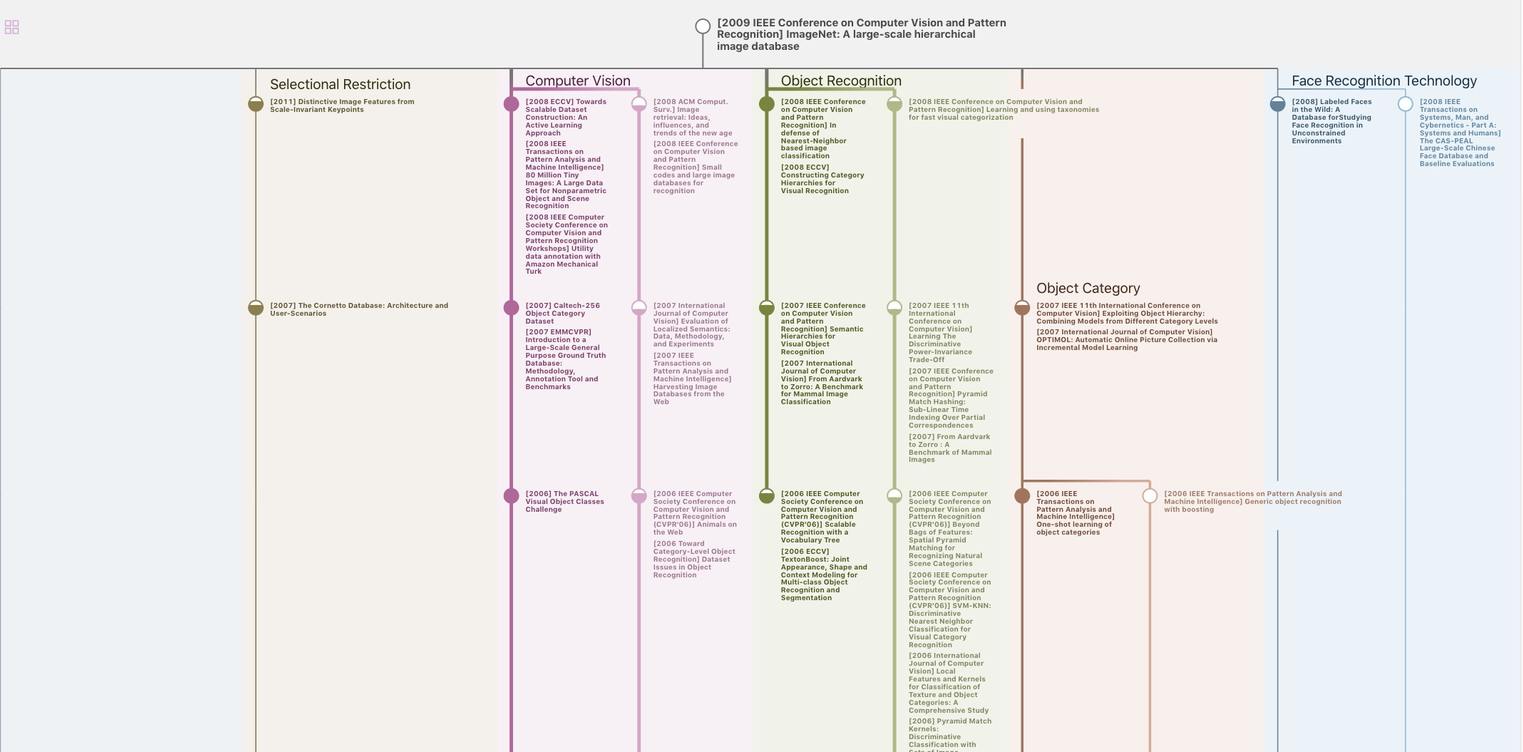
生成溯源树,研究论文发展脉络
Chat Paper
正在生成论文摘要