GWNN-HF: beyond assortativity in graph wavelet neural network
KNOWLEDGE AND INFORMATION SYSTEMS(2023)
摘要
Graph wavelet neural network exerts a powerful learning ability in assortative networks where most of the adjacent nodes have the same label as the target node. However, it does not perform well in disassortative networks where most of the adjacent nodes have different label than the target node. So graph wavelet neural network cannot extract the most useful information based on different types of networks. On the one hand, graph wavelet neural network is not able to extract the similarity information of the same labeled neighbor nodes and the difference information of different labeled neighbor nodes in a flexible way. On the other hand, graph wavelet neural network only aggregates neighbor nodes so that it cannot obtain information of nodes which have similar feature with the target node and are far from the target node. To solve the above problems, we propose the GWNN-HF model, which can effectively adapt to different types of networks and get a better node representation. Specifically speaking, firstly, we design low-pass filter and high-pass filter convolution kernels to get low-pass and high-pass signals and then use the adaptive fusion method to fuse them, which effectively get commonality of same label nodes and difference of different label nodes. Secondly, we use the Relaxed Minimum Spanning Tree algorithm to construct a feature correlation graph and use an attention mechanism to fuse the original graph and feature correlation graph representation. Extensive experiments on benchmark datasets clearly indicate that GWNN-HF has a good performance in different types of network structures.
更多查看译文
关键词
Graph neural network,Graph wavelet neural network,Assortative networks,Disassortative networks
AI 理解论文
溯源树
样例
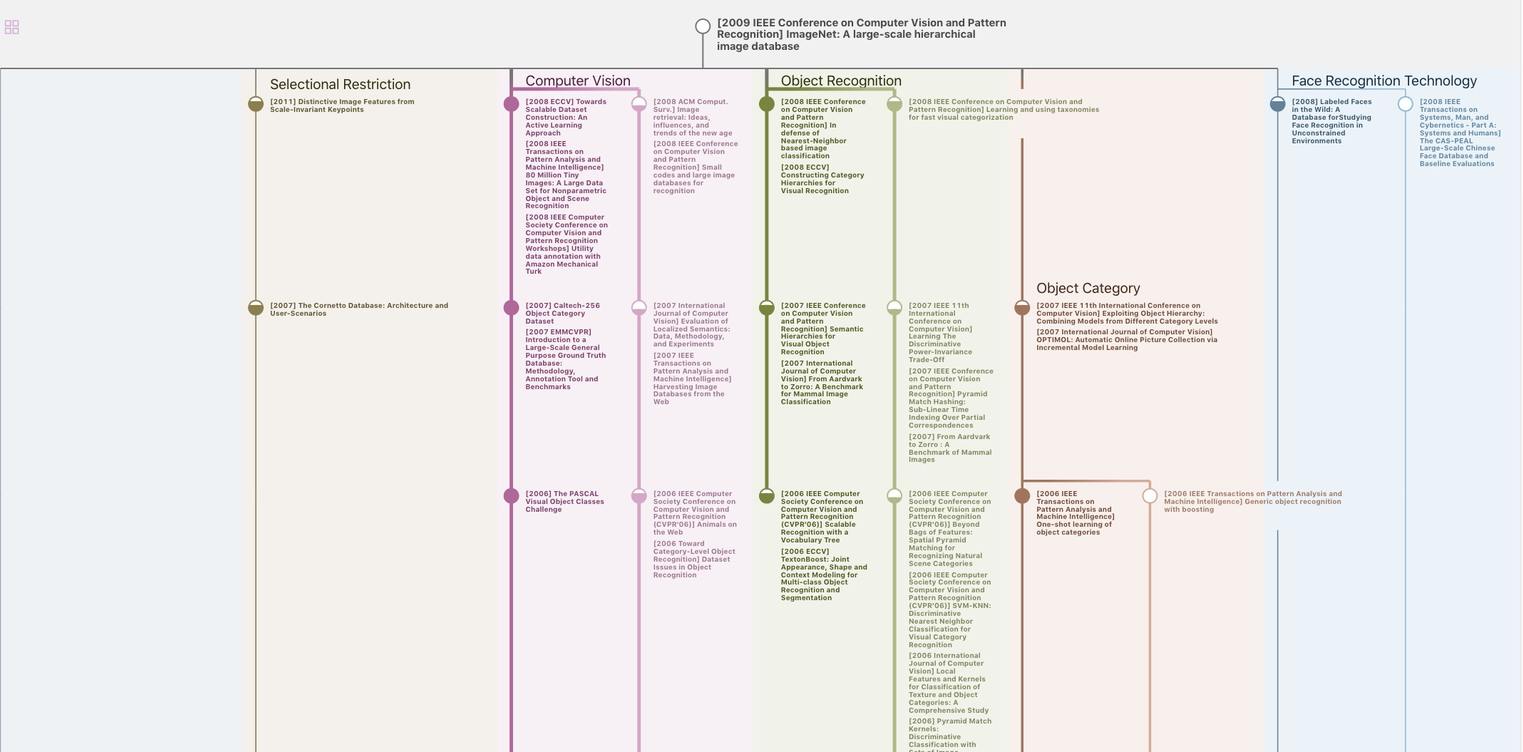
生成溯源树,研究论文发展脉络
Chat Paper
正在生成论文摘要