Two-Stage Self-Supervised Cycle-Consistency Transformer Network for Reducing Slice Gap in MR Images
IEEE Journal of Biomedical and Health Informatics(2023)
摘要
Magnetic resonance (MR) images are usually acquired with large slice gap in clinical practice,
i.e.
, low resolution (LR) along the through-plane direction. It is feasible to reduce the slice gap and reconstruct high-resolution (HR) images with the deep learning (DL) methods. To this end, the paired LR and HR images are generally required to train a DL model in a popular fully supervised manner. However, since the HR images are hardly acquired in clinical routine, it is difficult to get sufficient paired samples to train a robust model. Moreover, the widely used convolutional Neural Network (CNN) still cannot capture long-range image dependencies to combine useful information of similar contents, which are often spatially far away from each other across neighboring slices. To this end, a Two-stage Self-supervised Cycle-consistency Transformer Network (TSCTNet) is proposed to reduce the slice gap for MR images in this work. A novel self-supervised learning (SSL) strategy is designed with two stages respectively for robust network pre-training and specialized network refinement based on a cycle-consistency constraint. A hybrid Transformer and CNN structure is utilized to build an interpolation model, which explores both local and global slice representations. The experimental results on two public MR image datasets indicate that TSCTNet achieves superior performance over other compared SSL-based algorithms.
更多查看译文
关键词
mr images,reducing slice gap,transformer,two-stage,self-supervised,cycle-consistency
AI 理解论文
溯源树
样例
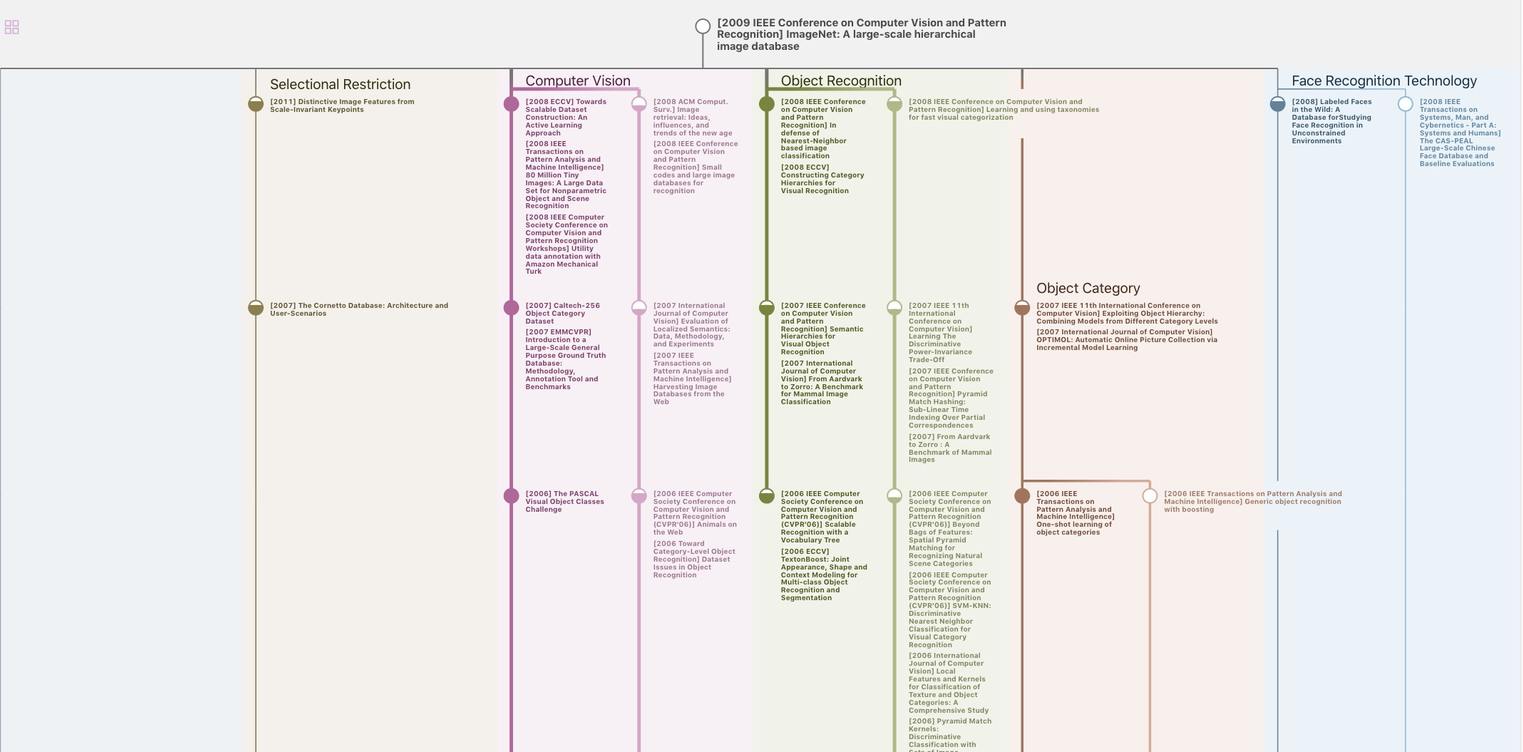
生成溯源树,研究论文发展脉络
Chat Paper
正在生成论文摘要