DICDP: Deep Incomplete Clustering with Distribution Preserving
Communications in computer and information science(2022)
摘要
Clustering is a fundamental task in the computer vision and machine learning community. Although various methods have been proposed, the performance of existing approaches drops dramatically when handling incomplete high-dimensional data (which is common in real world applications). To solve the problem, we propose a novel deep incomplete clustering method, named Deep Incomplete Clustering with Distribution Preserving (DICDP). To avoid insufficient sample utilization in existing methods limited by few fully-observed samples, we propose to measure distribution distance with the optimal transport for reconstruction evaluation instead of traditional pixel-wise loss function. Moreover, the clustering loss of the latent feature is introduced to regularize the embedding with more discrimination capability. As a consequence, the network becomes more robust against missing features and the unified framework which combines clustering and sample imputation enables the two procedures to negotiate to better serve for each other. Extensive experiments demonstrate that the proposed network achieves superior and stable clustering performance improvement against existing state-of-the-art incomplete clustering methods over different missing ratios.
更多查看译文
关键词
distribution preserving,clustering,deep incomplete
AI 理解论文
溯源树
样例
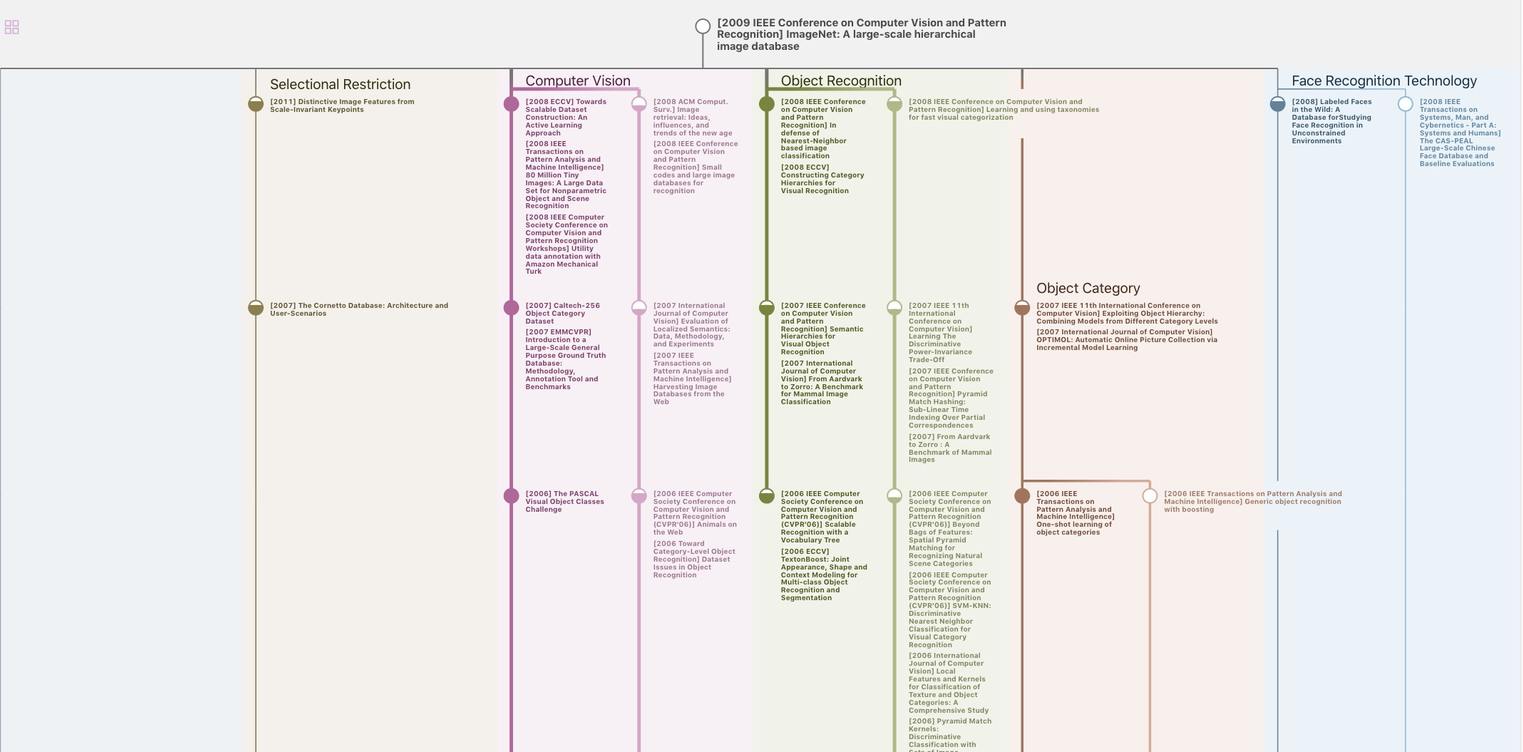
生成溯源树,研究论文发展脉络
Chat Paper
正在生成论文摘要