Bayesian Imaging with Data-Driven Priors Encoded by Neural Networks
SIAM Journal on Imaging Sciences(2022)
摘要
This paper proposes a new methodology for performing Bayesian inference in imaging inverse problems where the prior knowledge is available in the form of training data. Following the manifold hypothesis, we adopt a data-driven prior that is supported on a submanifold of the ambient space, which we can learn from the training data using a generative model, such as a variational autoencoder or generative adversarial network. We establish the existence and well-posedness of the associated posterior distribution and posterior moments under easily verifiable conditions, providing a rigorous underpinning for Bayesian estimators and uncertainty quantification analyses. Bayesian computation is performed using a parallel tempered version of the pCN algorithm on the manifold, which is shown to be ergodic and robust to the nonconvex nature of these data-driven models. In addition to point estimators and uncertainty quantification analyses, we derive a model misspecification test to automatically detect situations where the data-driven prior is unreliable, and we explain how to identify the dimension of the latent space directly from the training data. The proposed approach is illustrated with a range of experiments with the MNIST dataset and is compared with some variational and message passing image reconstruction approaches from the state of the art that also use data-driven regularization. A model accuracy analysis suggests that the Bayesian probabilities reported by the proposed data-driven models are also accurate under a frequentist definition of probability, suggesting that the learnt prior is close to the true marginal distribution of the unknown image.
更多查看译文
关键词
priors,imaging,neural networks,data-driven
AI 理解论文
溯源树
样例
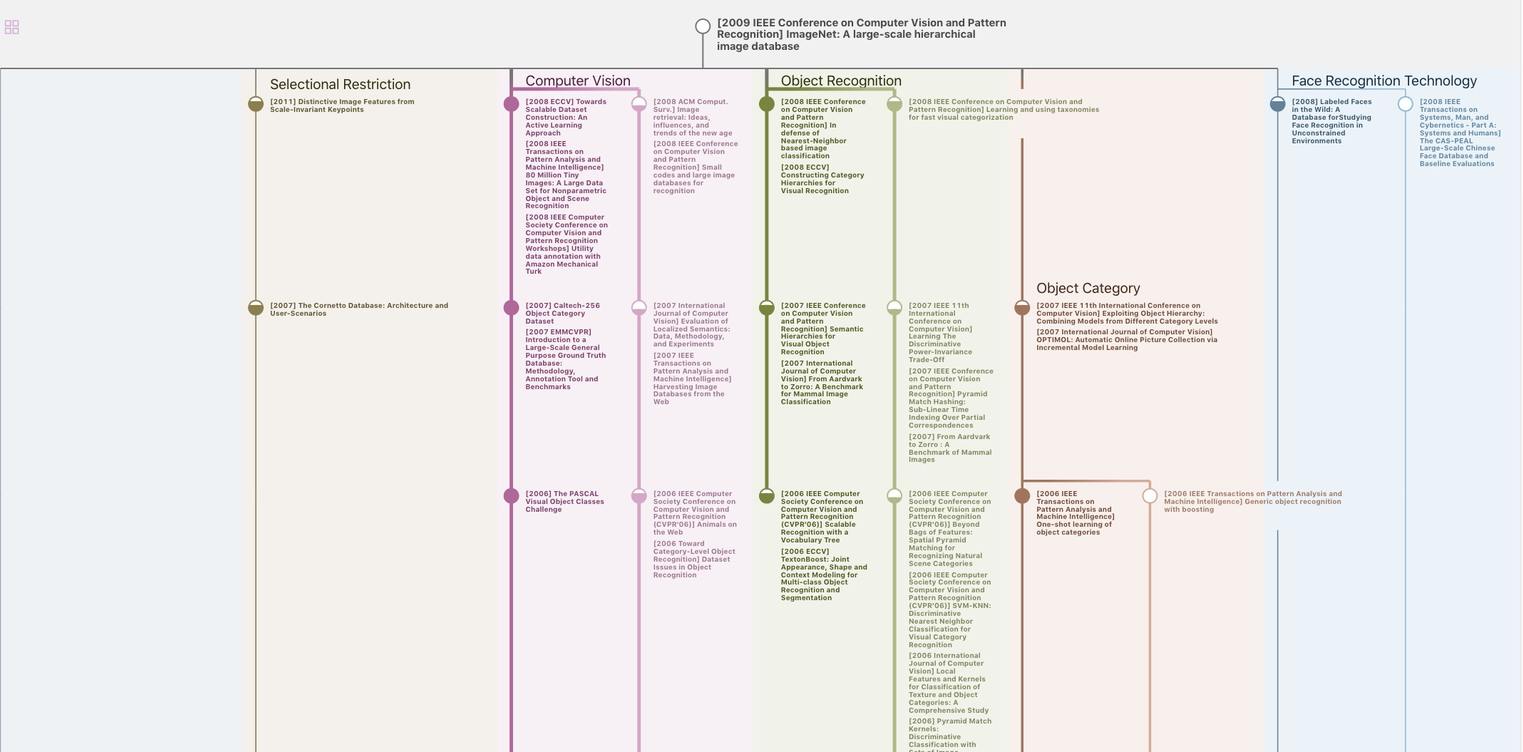
生成溯源树,研究论文发展脉络
Chat Paper
正在生成论文摘要