Primary caregiver considerations for developing and implementing a machine learning-based model for detecting child abuse and neglect: A qualitative study (Preprint)
crossref(2022)
摘要
BACKGROUND Child abuse and neglect, once viewed as a social problem, is now an epidemic. The broad adoption of electronic health records (EHR) in clinical settings offers a new avenue for addressing this epidemic. To improve the development, implementation, and outcomes of machine learning-based models that utilize EHR data, it is crucial to involve members of the community in the process. OBJECTIVE This study elicited primary caregivers' viewpoints regarding child abuse and neglect to highlight implications for designing a machine learning (ML)-based model for detecting child abuse and neglect in emergency departments (ED). METHODS We conducted a qualitative study using in-depth interviews with 20 primary caregivers whose children were cared for at a single pediatric tertiary-care ED to gain insights about child abuse and neglect and experiences with health providers. RESULTS Three central themes emerged from the interviews: (1) Primary caregivers perspectives on the definition of child abuse and neglect, (2) Primary caregivers experiences with health providers and medical documentation, and (3) Primary caregivers perceptions of child protective services. CONCLUSIONS Our findings highlight essential considerations from primary caregivers for developing an ML-based model for detecting child abuse and neglect in ED settings. This includes how to define child abuse and neglect from a primary caregiver lens. Miscommunication between patient and health provider can potentially lead to a misdiagnosis and therefore, have a negative impact on medical documentation. Additionally, the outcome and application of the machine learning-based models for detecting abuse and neglect may cause additional harm than expected to the community. Further research is needed to validate these findings and integrate them into creating an ML-based model.
更多查看译文
AI 理解论文
溯源树
样例
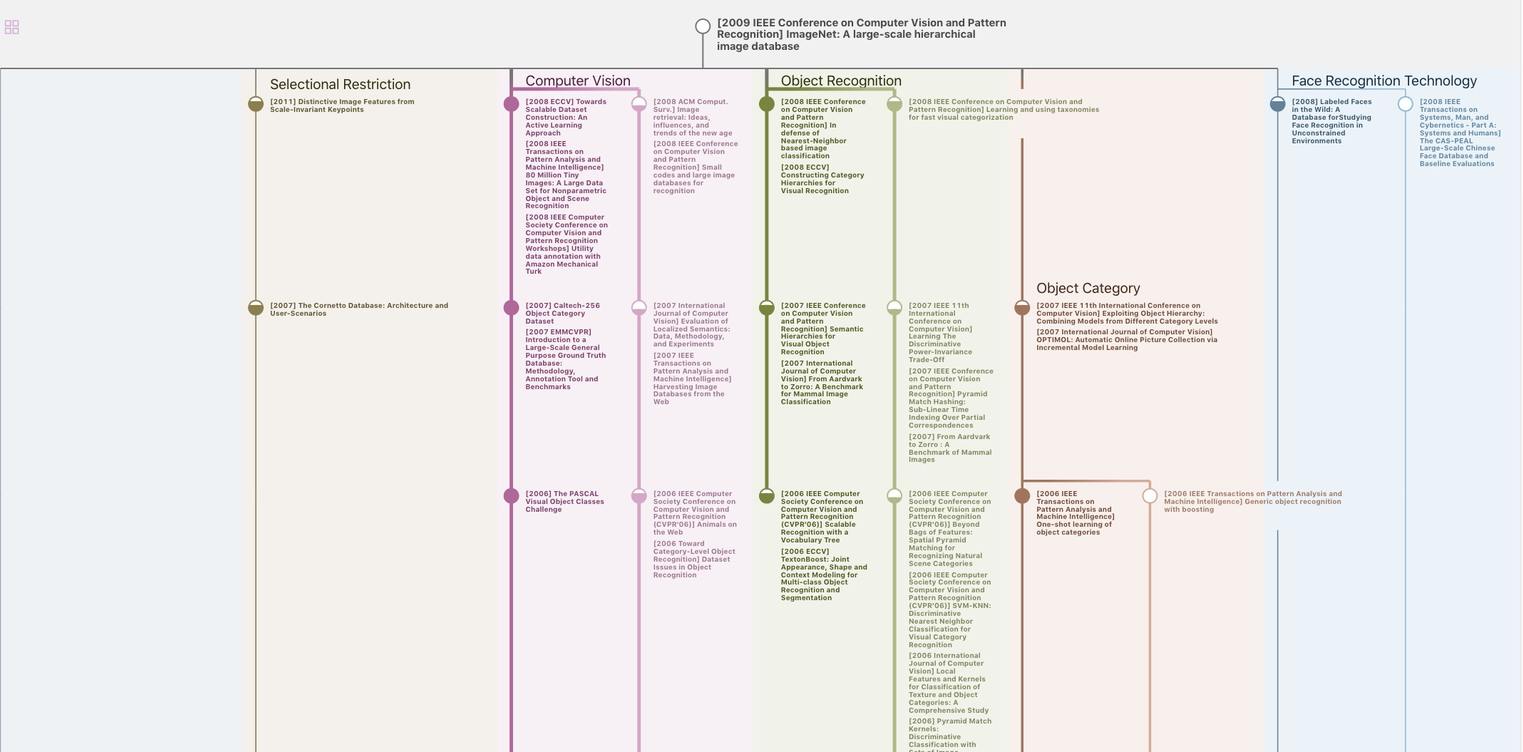
生成溯源树,研究论文发展脉络
Chat Paper
正在生成论文摘要