A Multivariate Approach for Spatiotemporal Mobile Data Traffic Prediction
Engineering Proceedings(2022)
摘要
Widespread deployment of spectrally efficient mobile networks, advancements in mobile devices, and proliferation of attractive applications has led to an exponential increase in mobile data traffic. Mobile Network Operators (MNOs) benefit from the associated revenue generation while putting efforts to meet customers’ expectations of delivered services. Having a clear knowledge of the traffic demand is critical for network dimensioning, optimization, resource allocation, market planning, and the like. As the traffic demand, among others, is a function of customers’ behavior and settlement patterns, land use, and time of the day, capturing traffic characteristics in both temporal and spatial dimensions is needed. Moreover, other parameters, such as the number of users and data throughput, inherently contain traffic-related information, necessitating a multivariate approach for understanding the traffic demand. Realizing the multidimensional and multivariate nature of the mobile data traffic, in this paper, we propose a multivariate and hybrid Convolutional Neural Network and Long Short-Term Memory network (CNN-LSTM) data traffic prediction model. The model is built on mobile traffic data collected from a Network Operator for Long-Term Evolution (LTE) network. The results confirm that the proposed model outperforms its univariate counterparts in Root Mean Square Error (RMSE) and Mean Absolute Percentage Error (MAPE) by 58% and 50%, respectively. Moreover, the model is further compared with CNN-only univariate and multivariate models, which it also outperforms. The comparisons substantiate the achievable improvements because of the hybrid and multivariate nature of the prediction algorithm.
更多查看译文
关键词
mobile data traffic,multivariate prediction,temporal,spatial,CNN,LSTM
AI 理解论文
溯源树
样例
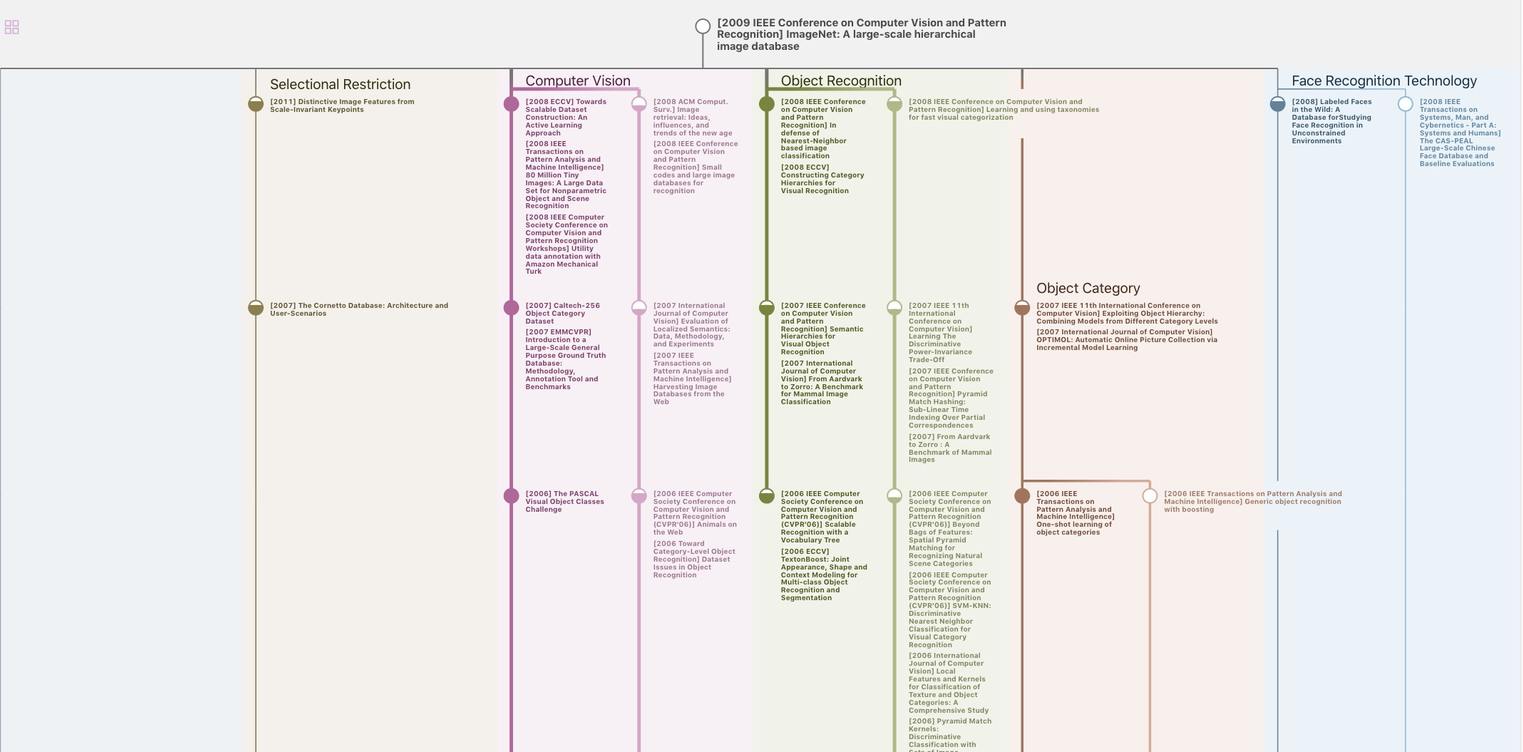
生成溯源树,研究论文发展脉络
Chat Paper
正在生成论文摘要