Expression of hub genes of endothelial cells in glioblastoma-A prognostic model for GBM patients integrating single cell RNA sequencing and bulk RNA sequencing
crossref(2022)
摘要
Abstract Background:Glioblastoma multiforme (GBM) is one of the most common malignancies in the world and many studies have used traditional high-throughput RNA sequencing (bulk RNA-seq) data to explore its potential prognostic markers. However, it is unable to detect specific cellular and molecular changes in tumour cells. The aim of this study was to use single cell RNA-seq (scRNA-seq) to discover marker genes in endothelial cells and to construct a prognostic model for GBM patients in combination with traditional RNA-seq data.Methods:Bulk RNA-seq data were downloaded from The Cancer Genome Atlas (TCGA) and The China Glioma Genome Atlas (CGGA) databases. 10x scRNA-seq data for GBM were obtained from the Gene Expression Omnibus (GEO) database. The uniform manifold approximation and projection (UMAP) was used for downscaling and cluster identification. Key modules and differentially expressed genes (DEGs) were identified by weighted gene correlation network analysis (WGCNA). Non-negative matrix decomposition (NMF) algorithm was used to identify different subtypes based on DEGS. And Cox regression analysis was used to build prognostic models. Finally, differences in mutational landscape, immune cell abundance, immune checkpoint inhibitors (ICIs)-associated genes, immunotherapy effects and enriched pathways were also investigated between different risk groups.RESULT:The analysis of scRNA-seq data from eight samples revealed 13 clusters and four cell types. After applying differential analysis, endothelial cells were identified as the most important significant cell type. In addition, we explored potential neoangiogenic pathways in GBM after controlling for phenotypic differences between GBM and normal brain tissue ECs at the single cell level. Overall, through differential analysis, WGCNA and screening of endothelial cell marker genes, we identified 157 DEGs for the construction of prognostic models. In addition, based on DEGs,the NMF algorithm identified two clusters with different prognostic and immunological features observed. We finally built a prognostic model based on the expression levels of four of these key genes. Higher risk scores were significantly associated with poorer survival outcomes and were associated with low mutation rates in IDH genes and upregulation of immune checkpoints such as PD-L1 and CD276. The CGGA cohort served as an external validation cohort for our findings.Conclusion:We built and validated a 4-gene signature for GBM using 10 scRNA-seq and bulk RNA-seq data in this work. We believe our findings will provide greater insight into the characteristics of ECs in GBM and provide potential prognostic biomarkers to design rational treatment plans.
更多查看译文
AI 理解论文
溯源树
样例
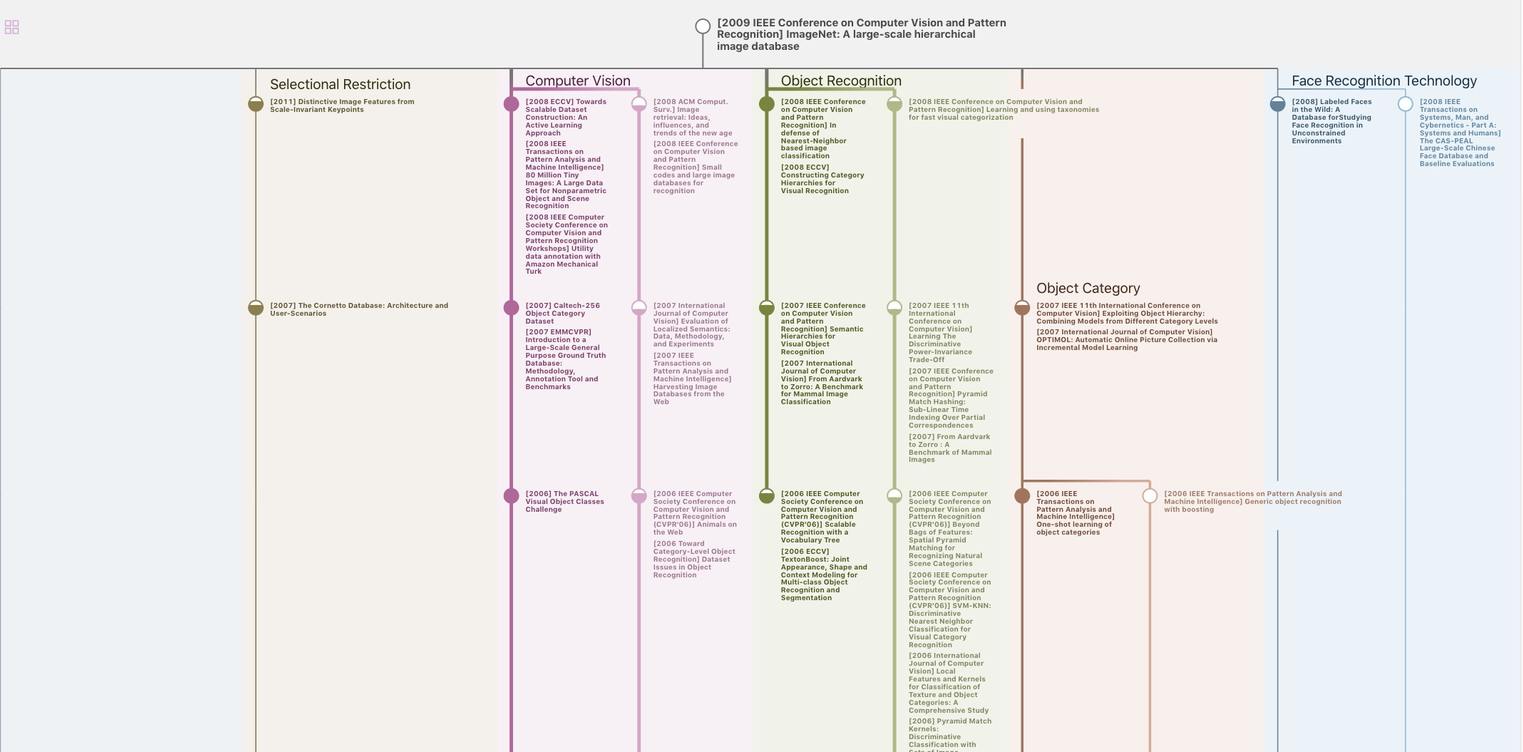
生成溯源树,研究论文发展脉络
Chat Paper
正在生成论文摘要