A kernel transfer learning based multi-sensor surface reconstruction framework for reverse engineering
Procedia CIRP(2022)
摘要
Reverse engineering (RE) has become increasingly important in the product development cycle and product design process, and it is used wildly for several years in industry. However, there is still room for increasing in the performance of RE. Indeed, this is the case in particular with the improvement of the quality of the initially measured data to increase the scope of applications in areas where the accuracy of the reconstructed model is a key element. The increasing requirements for high efficiency and accuracy in RE can be reached by the development of multi-sensor reconstruction techniques. Unlike existing data fusion solutions, this research redefines multi-sensor surface reconstruction as a transfer learning problem. A kernel transfer learning based multi-sensor reconstructed framework is proposed to transfer the common knowledge from the source data to the target data to take advantage of different sensors. A special combination kernel composed of a task kernel and a transfer kernel is designed to extract the transferable information and task-dependent information of two metrology data. The transferable common features are learned by training the task kernel using both datasets. And the conditional difference is captured by the transfer kernel learned on the probe points. Experiments are conducted on both simulated data and real surfaces to verify the effectiveness of the proposed framework. The merits and demerits are also studied comprehensively by statistical analysis and convergence analysis.
更多查看译文
关键词
Reverse engineering,measurement,transfer learning
AI 理解论文
溯源树
样例
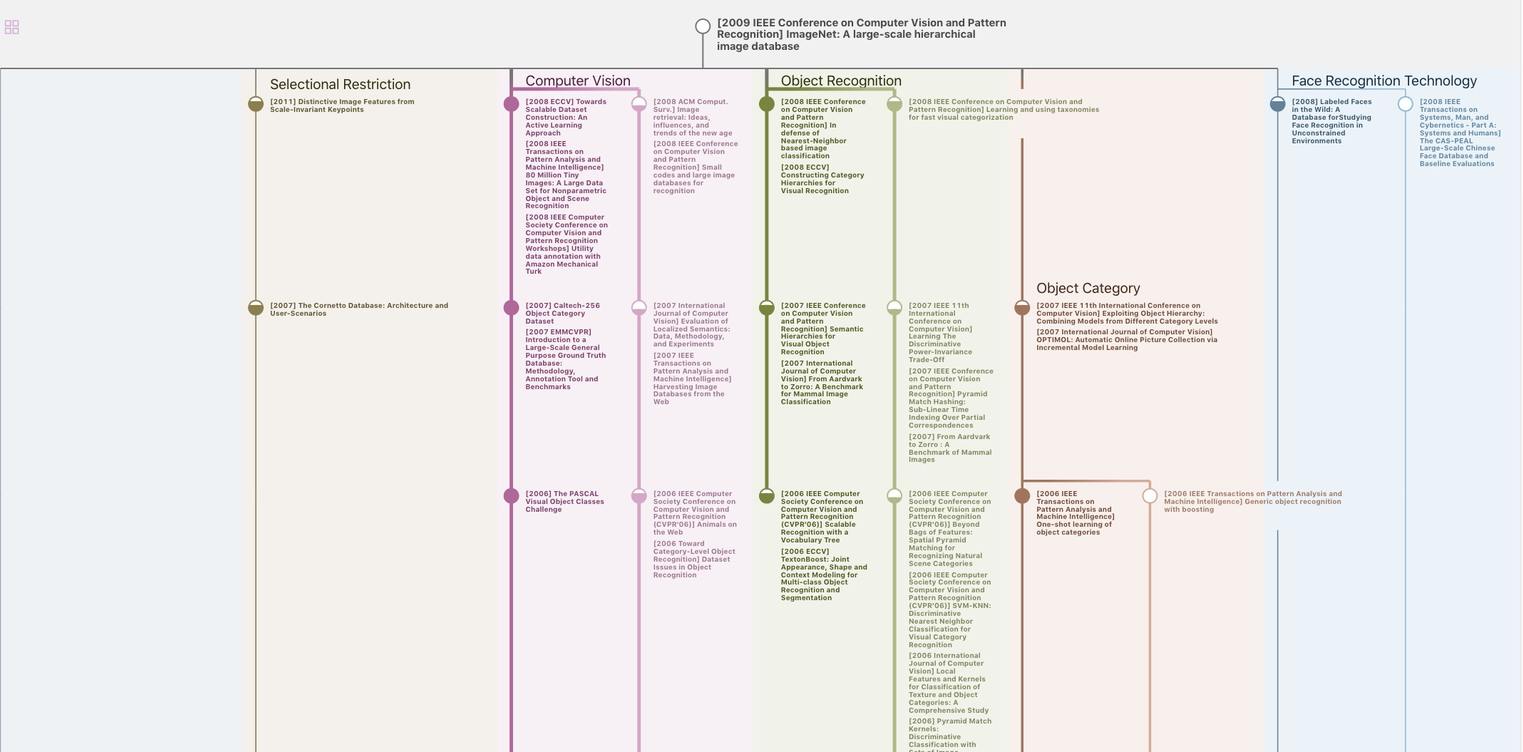
生成溯源树,研究论文发展脉络
Chat Paper
正在生成论文摘要