Abstract 462: Using attention-based deep multiple instance learning to identify key genetic alterations in prostate cancer from whole slide images
Cancer Research(2022)
摘要
Abstract Prostate cancer (PCa) is associated with several genetic alterations which play an important role in the disease heterogeneity and clinical outcome. These alterations involve gene fusion between TMPRSS2 and members of the ETS family of transcription factors like ERG, ETV1, and ETV4 together with mutations or deletions in tumor suppressors like TP53 and PTEN. The expanding wealth of digital whole slide images (WSIs) and the increasing adoption of deep learning approaches offer a unique opportunity for pathologists to streamline the detection of these alterations. Here, we used 736 haematoxylin and eosin-stained WSIs from 494 primary PCa patients to identify several key genetic alterations including ERG, ETV1, and ETV4 fusion, PTEN loss, and TP53 and SPOP mutations. Using a custom segmentation pipeline, we identified tissue regions and tiled them into high-resolution (10X magnification) patches (256X256 pixels) which were passed to our deep multiple instance learning framework. Using a pre-trained ResNet50 model, we extracted informative features which were subsequently used for training to predict slide-level labels and to detect slide regions with high diagnostic relevance. Using a 10-folds cross validation approach, we divided the data into training (80%), validation (10%) and testing (10%) sets. The training and validation data were used for training the model and hyperparameters tuning, respectively while the testing data was used to provide an unbiased evaluation of the models’ performance using the mean Area Under the Receiver Operating Characteristic (AUROC) across the ten testing folds as evaluation metric. We managed to accurately detect key molecular alterations including ERG fusion, ETV1 fusion, ETV4 fusion, and PTEN loss. Additionally, we were able to detect mutations in TP53 and SPOP together with the presence of androgen-receptor splice variant 7 (ARv7). In addition to slide-level classification, we also identified subregions with high attention score which can help pathologists identify the distinct morphological features associated with each genetic alteration. Finally, in order to examine the cellular structure associated with each genetic alteration, we used Hover-Net model to segment and classify the nuclei in the high-attention tiles. Our work highlights the utility of using WSIs to accurately identify key molecular alteration in cancer and their associated morphological and cellular features on the slide which would streamline the diagnostic process. To the best of our knowledge, this is the first study that uses routine WSIs to predict and characterize key genetic alterations in PCa. Citation Format: Mohamed Omar, Zhuoran Xu, Ryan Carelli, Jacob Rosenthal, David Brundage, Daniela C. Salles, Eddie L. Imada, Renato Umeton, Edward M. Schaeffer, Brian D. Robinson, Tamara L. Lotan, Massimo Loda, Luigi Marchionni. Using attention-based deep multiple instance learning to identify key genetic alterations in prostate cancer from whole slide images [abstract]. In: Proceedings of the American Association for Cancer Research Annual Meeting 2022; 2022 Apr 8-13. Philadelphia (PA): AACR; Cancer Res 2022;82(12_Suppl):Abstract nr 462.
更多查看译文
关键词
deep multiple instance,prostate cancer,key genetic alterations,attention-based
AI 理解论文
溯源树
样例
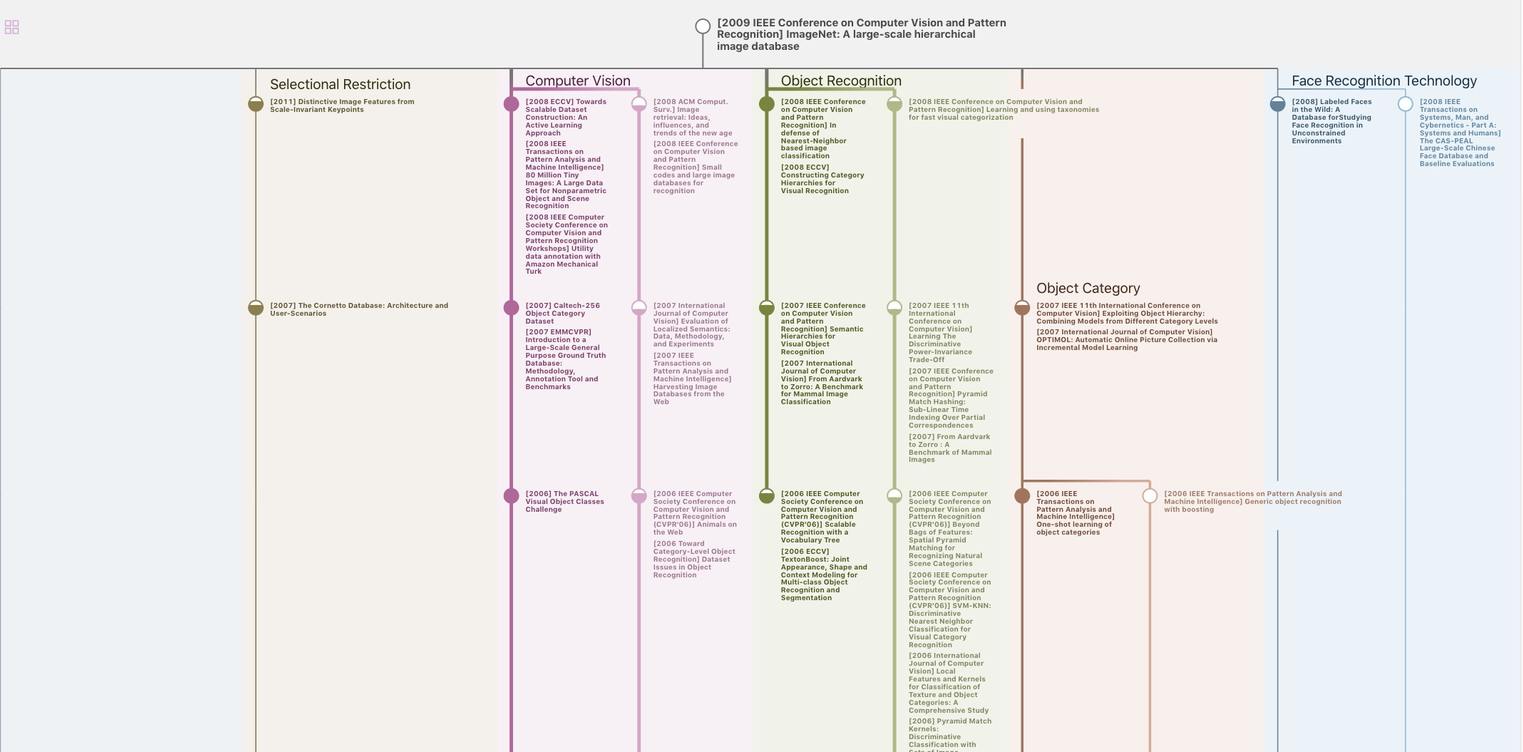
生成溯源树,研究论文发展脉络
Chat Paper
正在生成论文摘要