Abstract 461: Mixed supervision to improve the classification and localization: Coherence of tumors in histological slides
Cancer Research(2022)
摘要
Abstract With the growing standardization of Whole Slide Images (WSIs), deep learning algorithms have shown promising results for the automated classification and localization of tumors. Yet, it is often difficult to train such algorithms, as they usually require careful detailed annotations from expert pathologists, which are tedious to produce. This is why in general only slide-level labels are accessible while annotations of small regions (or tiles) are limited. With only slide-level information, it is difficult to obtain accurate predictions of the localization of pathological tissues inside a slide, despite reaching good slide-level classification. Besides, existing algorithms show limited consistency between slide- and tile-level predictions, leading to difficult interpretation in case of healthy tissue. Using the attention-based multiple instance learning framework, we propose to combine slide-level labels on all slides with tile-level labels on a small fraction (e.g. 20%) of slides within a histology dataset to improve both classification and localization performances. With this mixed supervision of slides, we aim to enforce a better consistency between slide- and tile-level labels. To this end, we introduce an attention-based loss function to further guide the model’s attention on discriminative regions inside tumorous slides, and display an equal attention among all tiles of normal slides. On the Camelyon16 dataset, we reached precision and recall scores as high as 0.99 and 0.85 respectively with an AUC of 0.93 on the competition test set, using only 50% of the slides with tile-level annotations in the training set. Experiments using various proportions of fully annotated slides in the training set show promising results for an improved localization of tumors and classification of slides. In this work, we showed that using a limited amount of fully annotated slides we can improve both the classification and localization performances of an attention-based deep learning model. This increased consistency and performances should help pathologists to better interpret the algorithm output and to focus on suspicious regions in probable tumorous slides. Citation Format: Paul Tourniaire, Marius Ilie, Paul Hofman, Nicholas Ayache, Hervé Delingette. Mixed supervision to improve the classification and localization: Coherence of tumors in histological slides [abstract]. In: Proceedings of the American Association for Cancer Research Annual Meeting 2022; 2022 Apr 8-13. Philadelphia (PA): AACR; Cancer Res 2022;82(12_Suppl):Abstract nr 461.
更多查看译文
关键词
tumors,mixed supervision,histological slides,coherence
AI 理解论文
溯源树
样例
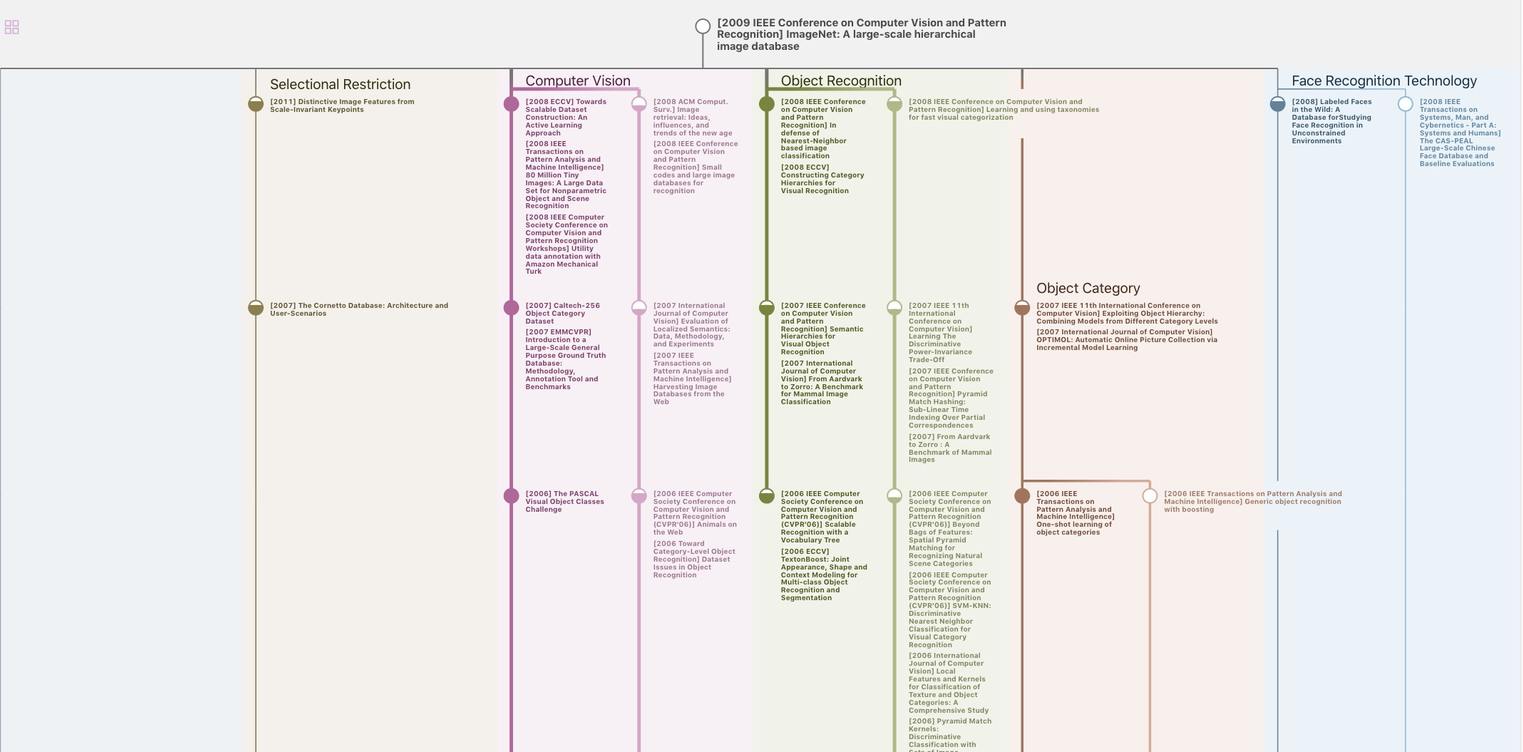
生成溯源树,研究论文发展脉络
Chat Paper
正在生成论文摘要