Validation of a nested cancer-subtype radiomic model to improve diagnostic accuracy for pulmonary nodule risk stratification
Cancer Research(2022)
摘要
Abstract Introduction: Building quantitative radiomic prediction models to improve the accuracy of non-invasive diagnosis of indeterminate pulmonary nodules (IPNs) is challenging, as these models are trained with a heterogeneous cancer population. The different subtypes of lung cancer have distinct radiologic appearances, and the most predictive radiological features for one subtype may not be useful in others. However, the management strategies of these IPNs are based upon the probability of the nodule being a cancer, regardless of subtype. We recently developed a predictive nested model which incorporates a series of subtype-models, each trained on a specific cancer histology, with each subtype model probability score secondarily used as predictive variables in a larger nested model. This work serves as a validation of the model in an independent cohort derived from patients who underwent bronchoscopic biopsy for their IPN deemed suspicious enough to warrant biopsy, which therefore represents a diagnostically challenging cohort of IPNs. Methods: Computed tomography (CT) images from 263 patients with IPNs measuring 6-30 mm were included from the Vanderbilt University Medical Center navigation bronchoscopy cohort. 180 patients (68%) were ultimately diagnosed with lung cancer: 91 adenocarcinoma (ADC), 27 squamous cell carcinoma (SCC), 7 small cell lung cancer (SCLC) and 55 other non-small cell lung cancer. Nodules were segmented using HealthMyne image analysis platform (Healthmyne, Madison WI). The probability of cancer for each patient was calculated using the Mayo Clinic model (the baseline model), and then using a previously trained nested-generalized linear model (N-GLM). Model performance was assessed using receiver operator characteristic (ROC) area under the curve (AUC) using bootstrap validation. Results: The ROC AUC showed that the N-GLM outperformed the Mayo Clinic model, with an AUC of 0.64 (95% C.I. of 0.54-0.70) for the N-GLM vs 0.55 (0.49-0.65) for the Mayo Clinic model. The N-GLM also outperformed our previously reported model trained using a logistic regression model on all lung cancers vs benign (AUC of 0.61, 95% CI of 0.46-0.63). Conclusions: A set of radiomic predictive models trained against each cancer subtype which were then nested within a larger predictive model was validated and found to outperform the clinical baseline Mayo Clinic Model and other quantitative radiomic models among a cohort comprised of the most diagnostically challenging indeterminate pulmonary nodules. Citation Format: khushbu H. Patel, Michael N. Kammer, Dianna J. Rowe, Brent E. Heideman, Sanja L. antic, Fabien Maldonado. Validation of a nested cancer-subtype radiomic model to improve diagnostic accuracy for pulmonary nodule risk stratification [abstract]. In: Proceedings of the American Association for Cancer Research Annual Meeting 2022; 2022 Apr 8-13. Philadelphia (PA): AACR; Cancer Res 2022;82(12_Suppl):Abstract nr 1426.
更多查看译文
关键词
diagnostic accuracy,radiomic model,cancer-subtype
AI 理解论文
溯源树
样例
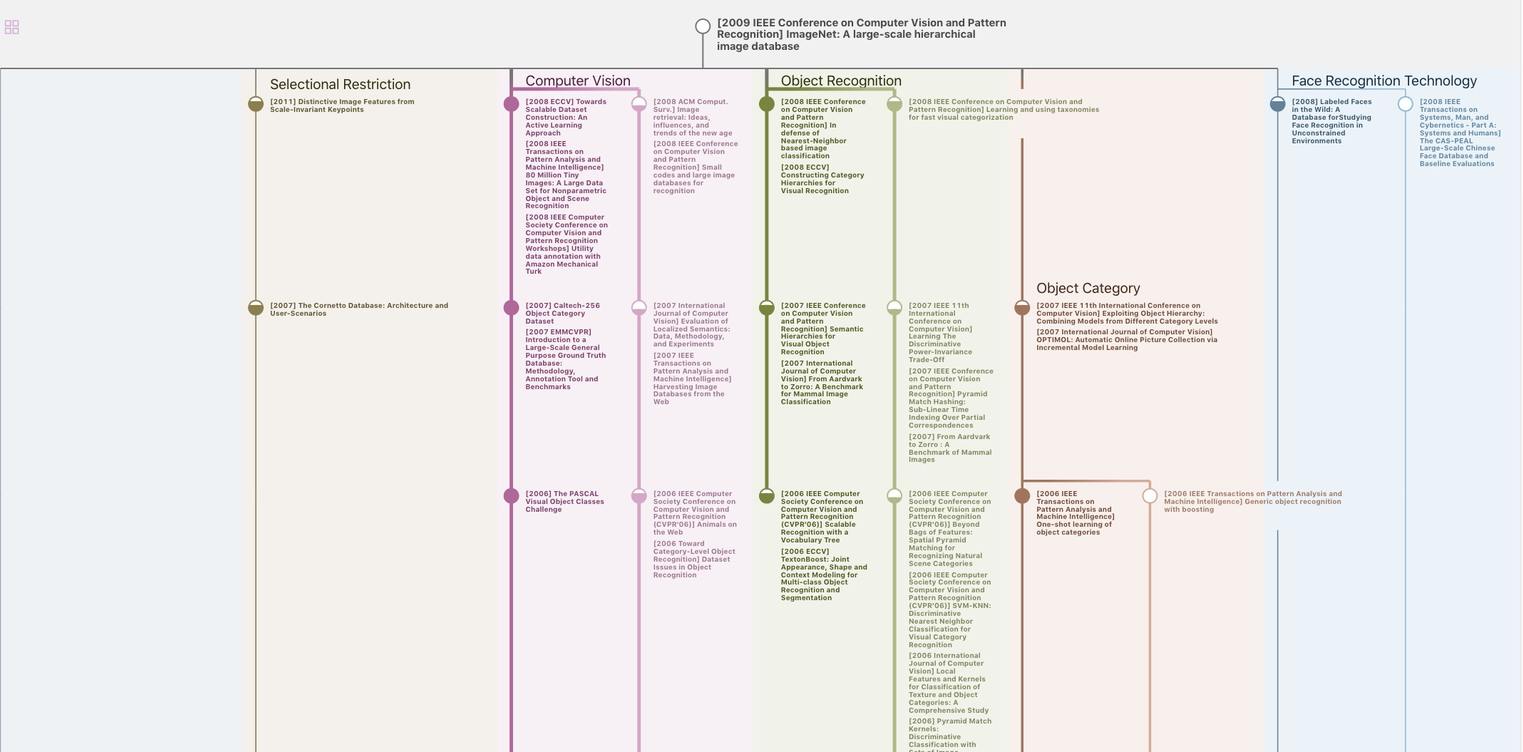
生成溯源树,研究论文发展脉络
Chat Paper
正在生成论文摘要