Abstract 4100: Developmental deconvolution for classification of cancer origin
Cancer Research(2022)
摘要
Abstract Cancer is a disease manifesting in abrogation of developmental programs, and malignancies are namedbased on their cell or tissue of origin. However, a systematic atlas of tumor origins is lacking. Here we map the single cell organogenesis of 56 developmental trajectories to the transcriptomes of over 10,000 tumors across 33 cancer types. We use this map to deconvolute individual tumors into their constituent developmental components. Based on these deconvoluted developmental programs, we construct a Developmental Machine Learning Perceptron (D-MLP) classifier that outputs cancer origin. The D-MLP classifier (ROC-AUC: 0.974 for top prediction) outperforms classification based on expression of either oncogenes or highly variable genes. We analyze tumors from patients with cancer of unknown primary (CUP), selecting the most difficult cases where extensive multimodal workup yielded no definitive tumor type. D-MLP revealed insights into developmental origins and diagnosis for most patient tumors. Our results provide a map of tumor developmental origins, provide a tool for diagnostic pathology, and suggest developmental classification may be a useful approach for otherwise unclassified patient tumors. Citation Format: Enrico Moiso, Alexander Farahani, Hetal Marble, Austin Hendricks, Samuel Mildrum, Stuart Levine, Jochen Lennerz, Salil Garg. Developmental deconvolution for classification of cancer origin [abstract]. In: Proceedings of the American Association for Cancer Research Annual Meeting 2022; 2022 Apr 8-13. Philadelphia (PA): AACR; Cancer Res 2022;82(12_Suppl):Abstract nr 4100.
更多查看译文
关键词
developmental deconvolution,cancer origin,classification
AI 理解论文
溯源树
样例
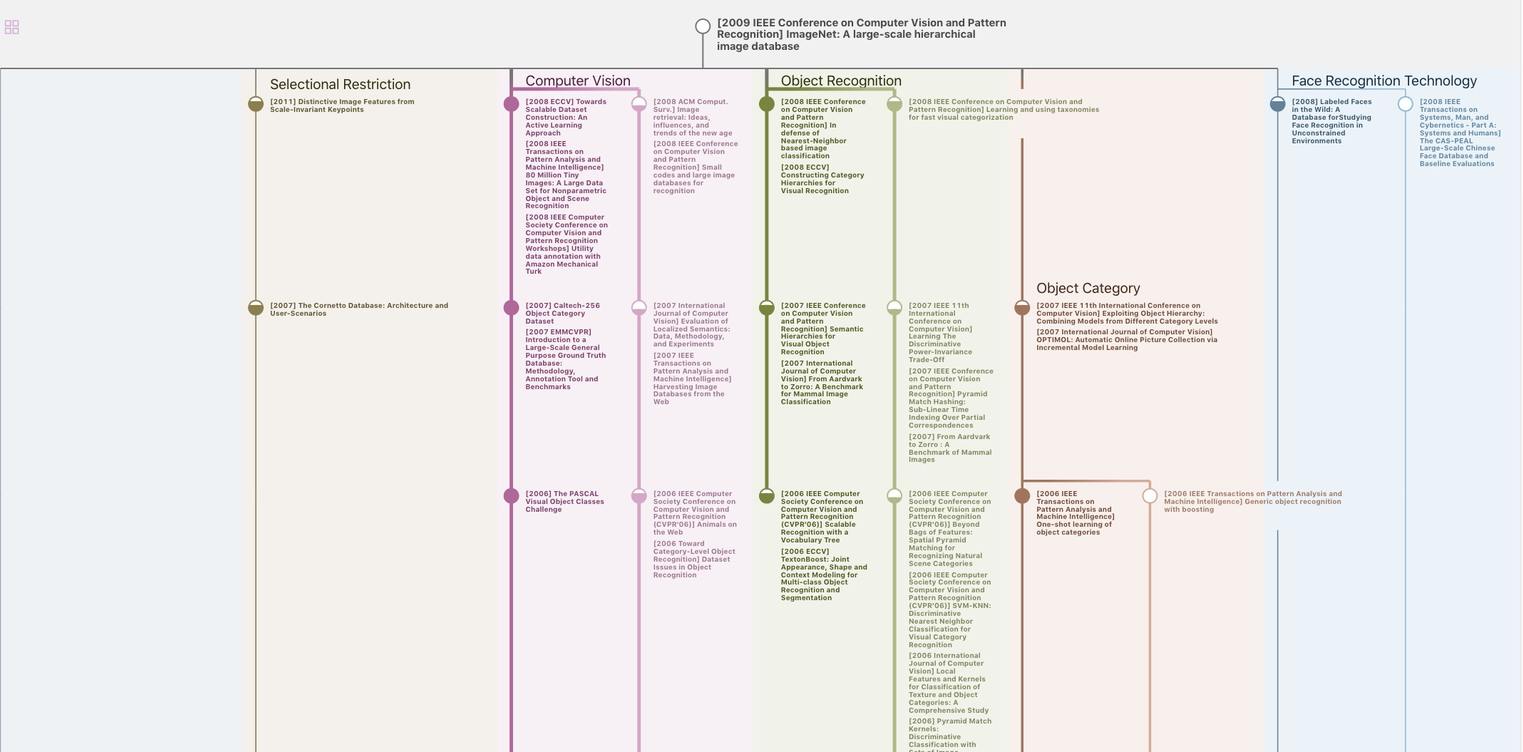
生成溯源树,研究论文发展脉络
Chat Paper
正在生成论文摘要