Abstract 1924: PULS-AI: A multimodal artificial intelligence model to predict survival of solid tumor patients treated with antiangiogenics
Cancer Research(2022)
摘要
Abstract The need for developing new biomarkers is increasing with the emergence of many targeted therapies. In this study, we used artificial intelligence (AI) to develop a multimodal model (PULS-AI) predicting the survival of solid tumor patients treated with antiangiogenic treatments. Our retrospective, multicentric study included 616 patients with 7 different cancer types: renal cell carcinoma, colorectal carcinoma, hepatocellular carcinoma, gastrointestinal carcinoma, melanoma, breast cancer, and sarcoma. A set of 196 patients was left out of the study for validation. Clinical data including patient, treatment, and cancer metadata were collected at baseline for all patients, as well as computed tomography (CT) and ultrasound (US) images. Radiologists annotated all metastases on the CT images and the visible tumor lesion on the US images. AI models were used to extract relevant features from the regions of interest on CT and US images. In addition, handcrafted features related to the tumor burden were extracted from the annotations of all lesions on CT such as the number of lesions and the tumor burden volume per organ (lungs, liver, skull, bone, other). Finally, a Cox regression model was fitted to the set of imaging features and clinical features. The annotation process led to 1147 annotated US images with lesions delineation and 4564 reviewed CTs, of which 989 were selected and fully annotated with a total of 9516 annotated lesions.The developed model reaches an average concordance index of 0.71 (0.67-0.75, 95% CI). Using a risk threshold of 50%, PULS-AI model is able to significantly isolate (log-rank test P-value < 0.001) high-risk patients from low-risk patients (respective median OS of 12 and 32 months) with a hazard ratio of 3.52 (2.35-5.28, 95% CI). The results of this study show that AI algorithms are able to extract relevant information from radiology images and to aggregate data from multiple modalities to build powerful prognostic tools. Such tools may provide assistance to oncology clinicians in therapeutic decision-making. Citation Format: Kathryn Schutte, Fabien Brulport, Sana Harguem-Zayani, Jean-Baptiste Schiratti, Ridouane Ghermi, Paul Jehanno, Alexandre Jaeger, Talal Alamri, Raphael Naccache, Leila Haddag-Miliani, Teresa Orsi, Jean-Philippe Lamarque, Isaline Hoferer, Littisha Lawrance, Baya Benatsou, Imad Bousaid, Mickael Azoulay, Antoine Verdon, François Bidault, Corinne Balleyguier, Victor Aubert, Etienne Bendjebbar, Charles Maussion, Nicolas Loiseau, Benoit Schmauch, Meriem Sefta, Gilles Wainrib, Thomas Clozel, Samy Ammari, Nathalie Lassau. PULS-AI: A multimodal artificial intelligence model to predict survival of solid tumor patients treated with antiangiogenics [abstract]. In: Proceedings of the American Association for Cancer Research Annual Meeting 2022; 2022 Apr 8-13. Philadelphia (PA): AACR; Cancer Res 2022;82(12_Suppl):Abstract nr 1924.
更多查看译文
关键词
antiangiogenics,multimodal artificial intelligence model,solid tumor patients,artificial intelligence
AI 理解论文
溯源树
样例
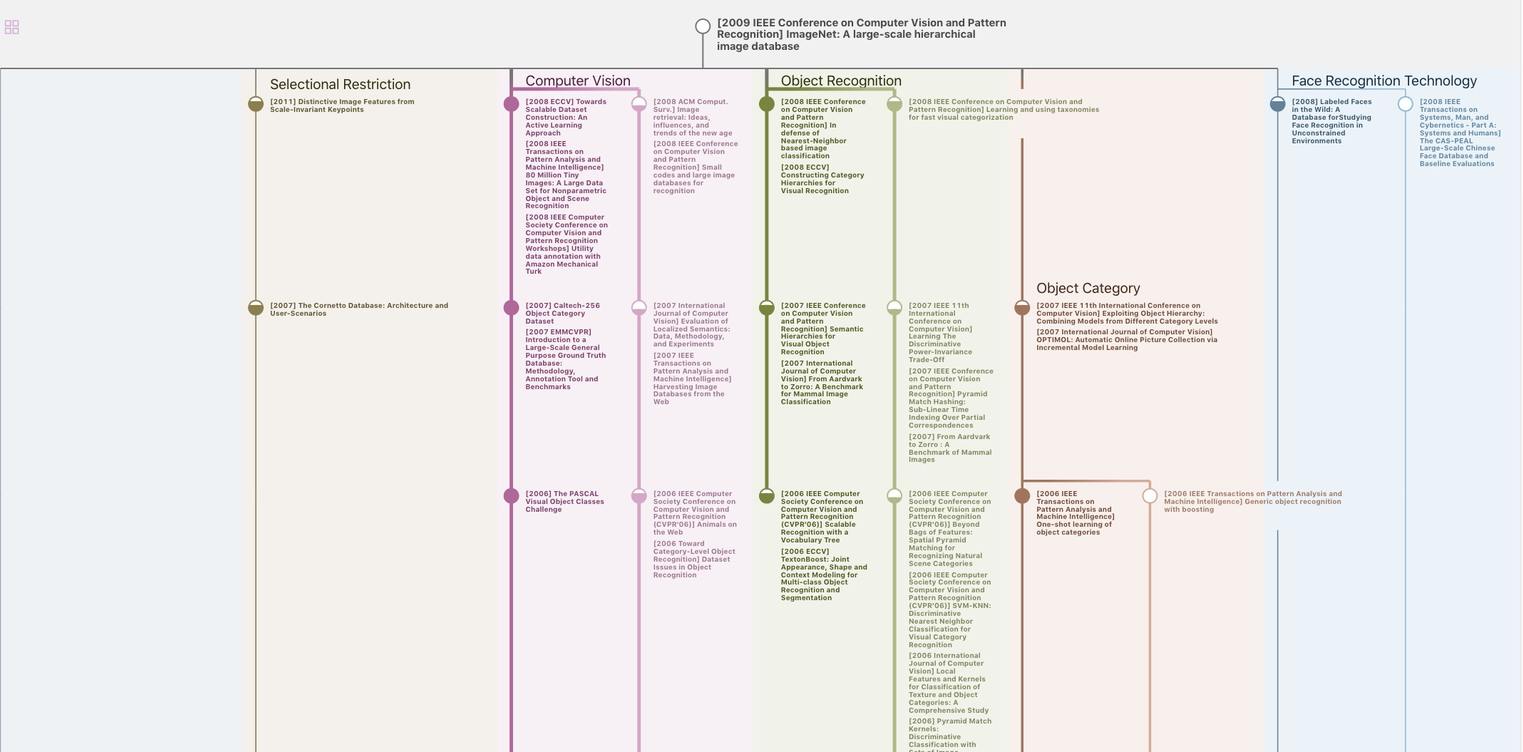
生成溯源树,研究论文发展脉络
Chat Paper
正在生成论文摘要