Deep Learning for Automatic Differential Diagnosis of Primary Central Nervous System Lymphoma and Glioblastoma: Multi-Parametric Magnetic Resonance Imaging Based Convolutional Neural Network Model.
Journal of magnetic resonance imaging(2021)
摘要
BackgroundDifferential diagnosis of primary central nervous system lymphoma (PCNSL) and glioblastoma (GBM) is useful to guide treatment strategies.PurposeTo investigate the use of a convolutional neural network (CNN) model for differentiation of PCNSL and GBM without tumor delineation.Study TypeRetrospective.PopulationA total of 289 patients with PCNSL (136) or GBM (153) were included, the average age of the cohort was 54 years, and there were 173 men and 116 women.Field Strength/Sequence3.0 T Axial contrast‐enhanced T1‐weighted spin‐echo inversion recovery sequence (CE‐T1WI), T2‐weighted fluid‐attenuation inversion recovery sequence (FLAIR), and diffusion weighted imaging (DWI, b = 0 second/mm2, 1000 seconds/mm2).AssessmentA single‐parametric CNN model was built using CE‐T1WI, FLAIR, and the apparent diffusion coefficient (ADC) map derived from DWI, respectively. A decision‐level fusion based multi‐parametric CNN model (DF‐CNN) was built by combining the predictions of single‐parametric CNN models through logistic regression. An image‐level fusion based multi‐parametric CNN model (IF‐CNN) was built using the integrated multi‐parametric MR images. The radiomics models were developed. The diagnoses by three radiologists with 6 years (junior radiologist Y.Y.), 11 years (intermediate‐level radiologist Y.T.), and 21 years (senior radiologist Y.L.) of experience were obtained.Statistical AnalysisThe 5‐fold cross validation was used for model evaluation. The Pearson's chi‐squared test was used to compare the accuracies. U‐test and Fisher's exact test were used to compare clinical characteristics.ResultsThe CE‐T1WI, FLAIR, and ADC based single‐parametric CNN model had accuracy of 0.884, 0.782, and 0.700, respectively. The DF‐CNN model had an accuracy of 0.899 which was higher than the IF‐CNN model (0.830, P = 0.021), but had no significant difference in accuracy compared to the radiomics model (0.865, P = 0.255), and the senior radiologist (0.906, P = 0.886).Data ConclusionA CNN model can differentiate PCNSL from GBM without tumor delineation, and comparable to the radiomics models and radiologists.Level of Evidence4Technical EfficacyStage 2
更多查看译文
关键词
central nervous system neoplasms,glioblastoma,magnetic resonance imaging,deep learning,neural networks,computer
AI 理解论文
溯源树
样例
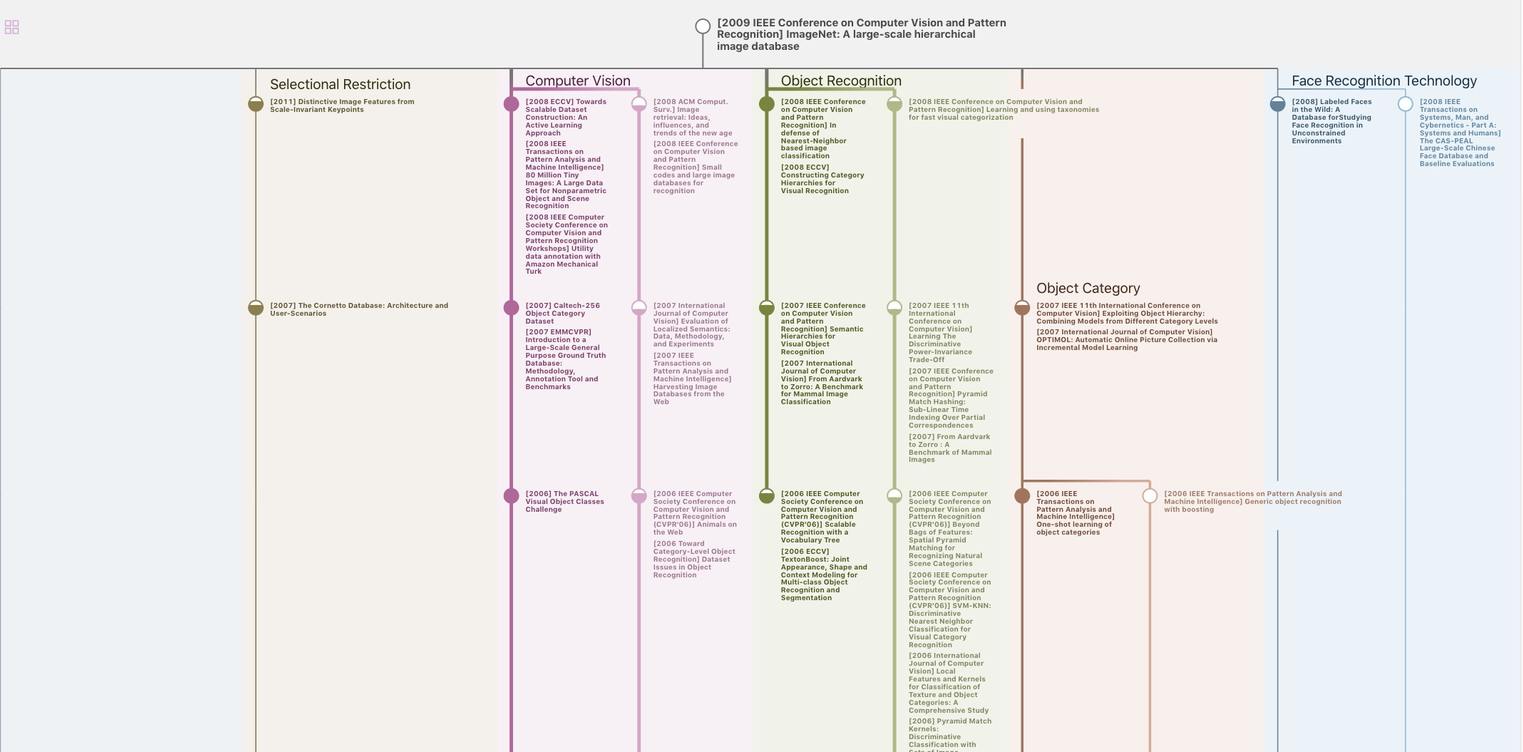
生成溯源树,研究论文发展脉络
Chat Paper
正在生成论文摘要