Implementation of a Four-Class Motor Imagery Brain Computer Interface Using Long Short-Term Memory Neural Network
International Journal of Clinical Medicine and Bioengineering(2022)
摘要
We have developed multi-channel dry-electrode electroencephalography (EEG) system to implement a brain computer interface (BCI) and discriminate four-class motor imagery signals. The EEG channels recorded from Fz, F3, F4, C3, Cz, C4, P3, and P4 positions, according to the international 10‒20 EEG system, were acquired and wirelessly transmitted to a personal computer. Five subjects were recruited to perform right hand, left hand, right foot, and left foot imagery movements with 60 trials in each movement type. EEG data were segmented into epochs from 0 to 8 s and anchored to time points of imagery movement cues. The segmented EEG epochs were pre-processed using the Morlet wavelet. A long short-term memory (LSTM) neural network with 64 LSTM cells was constructed to discriminate EEG signals recorded from different imagery movements. Among total EEG epochs, 80% were randomly chosen as training data and the rest 20% were used as validation data. The detection accuracy reached 89%.
更多查看译文
AI 理解论文
溯源树
样例
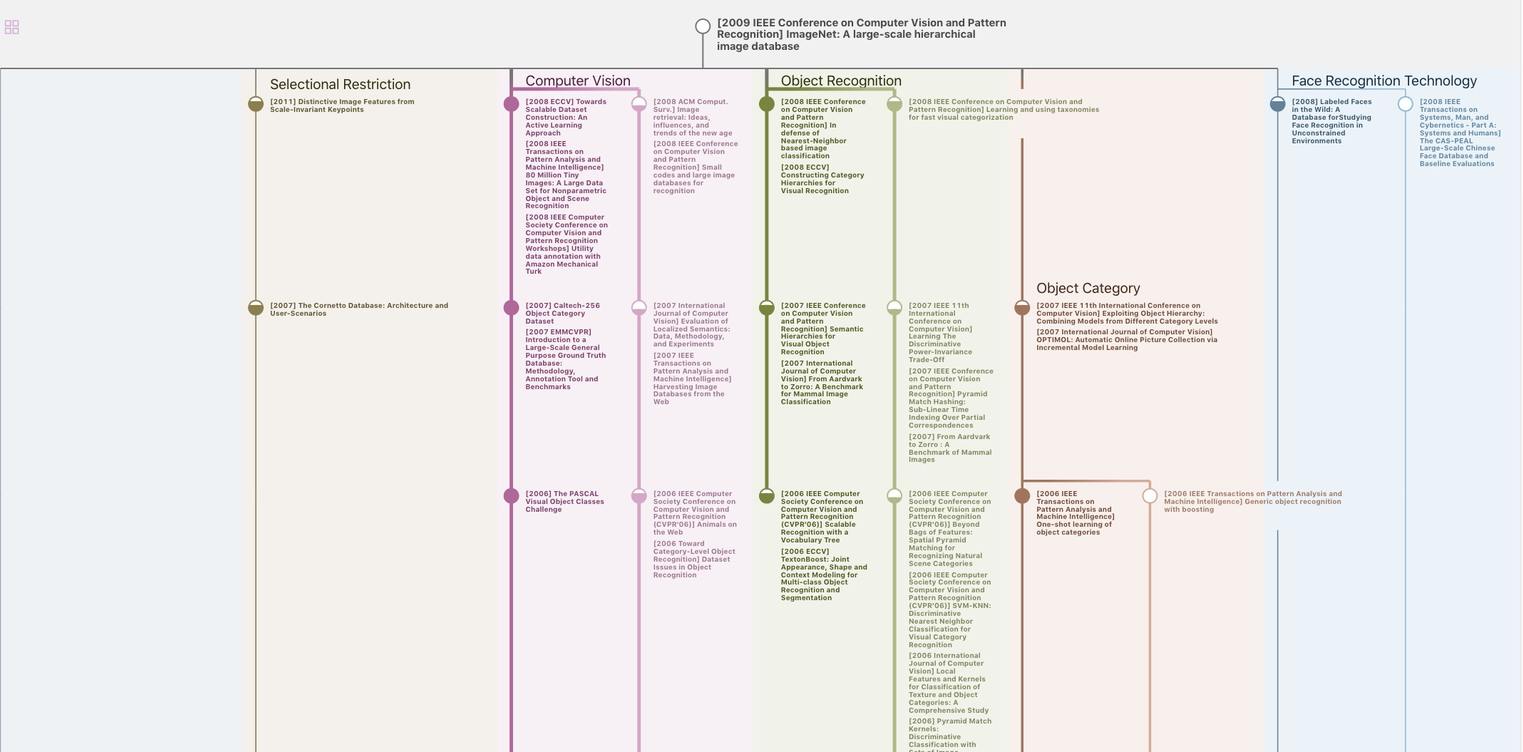
生成溯源树,研究论文发展脉络
Chat Paper
正在生成论文摘要