A Deep Convolutional Spiking Neural Network for embedded applications
Progress in Artificial Intelligence(2024)
摘要
Deep neural networks (DNNs) have received a great deal of interest in solving everyday tasks in recent years. However, their computational and energy costs limit their use on mobile and edge devices. The neuromorphic computing approach called spiking neural networks (SNNs) represents a potential solution for bridging the gap between performance and computational expense. Despite the potential benefits of energy efficiency, the current SNNs are being used with datasets such as MNIST, Fashion-MNIST, and CIFAR10, limiting their applications compared to DNNs. Therefore, the applicability of SNNs to real-world applications, such as scene classification and forecasting epileptic seizures, must be demonstrated yet. This paper develops a deep convolutional spiking neural network (DCSNN) for embedded applications. We explore a convolutional architecture, Visual Geometry Group (VGG16), to implement deeper SNNs. To train a spiking model, we convert the pre-trained VGG16 into corresponding spiking equivalents with nearly comparable performance to the original one. The trained weights of VGG16 were then transferred to the equivalent SNN architecture while performing a proper weight–threshold balancing. The model is evaluated in two case studies: land use and land cover classification, and epileptic seizure detection. Experimental results show a classification accuracy of 94.88
更多查看译文
关键词
Spiking neural network,Satellite image classification,Epileptic seizure detection
AI 理解论文
溯源树
样例
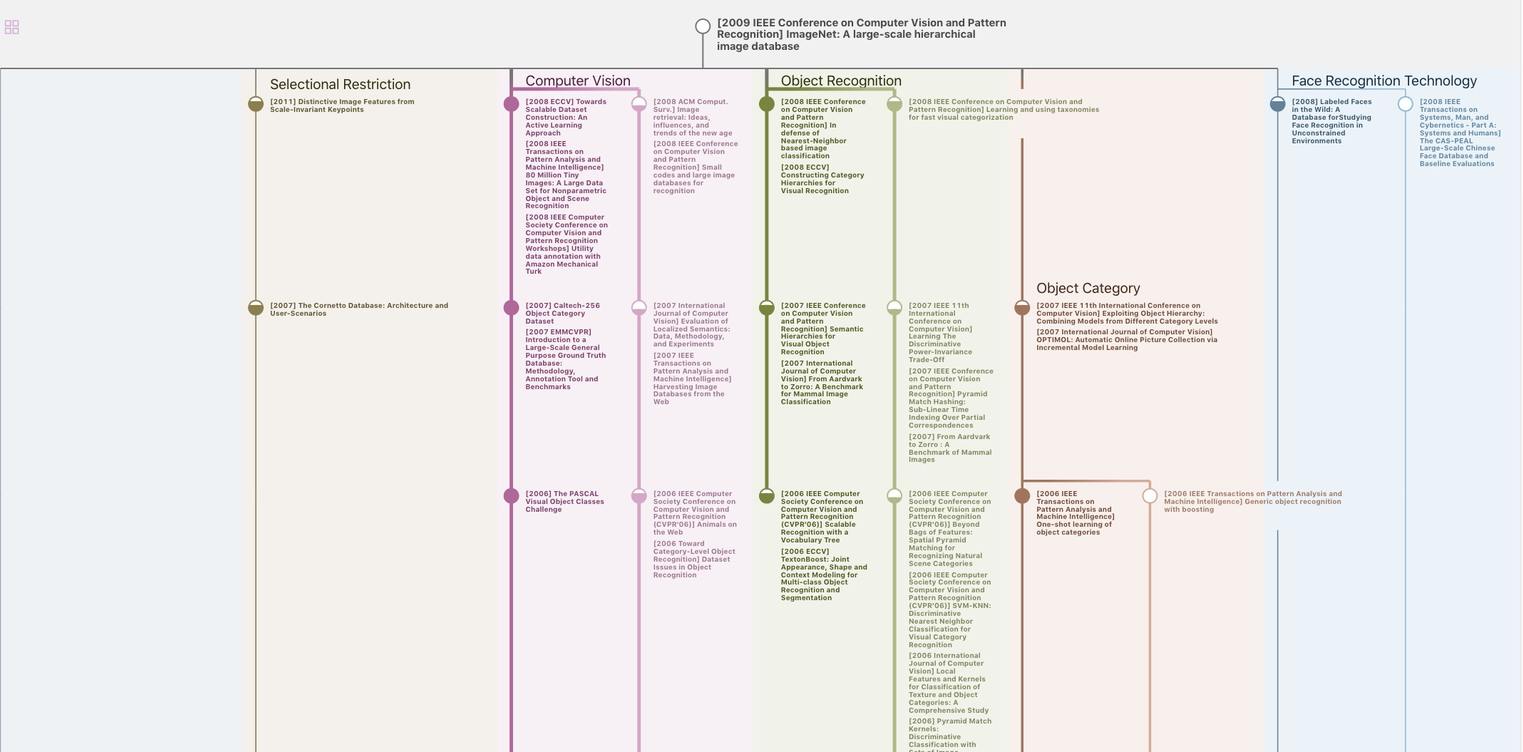
生成溯源树,研究论文发展脉络
Chat Paper
正在生成论文摘要