Speakers prioritize whole object semantics in scene descriptions
crossref(2022)
Abstract
This work investigates the linearization strategies used by speakers when describing real-world scenes to better understand production plans for multi-utterance sequences. Scene meaning predicts visual attention across tasks, but does not predict the order in which objects in scenes are described, contrary to previous work suggesting a tight coupling between the visual attention and language production systems in simple description tasks. In this study, 30 participants described real-world scenes aloud. To investigate which semantic features of scenes predict order of mention, we quantified three features (meaning, graspability, and interactability) using two techniques (whole-object ratings and feature map values). We found that object-level semantic features, broadly defined and affordance-based, predicted order of mention in a scene description task. Our findings provide the first evidence for an object-related semantic feature that guides linguistic ordering decisions and offer theoretical support for the role of object semantics in scene viewing and description.
MoreTranslated text
AI Read Science
Must-Reading Tree
Example
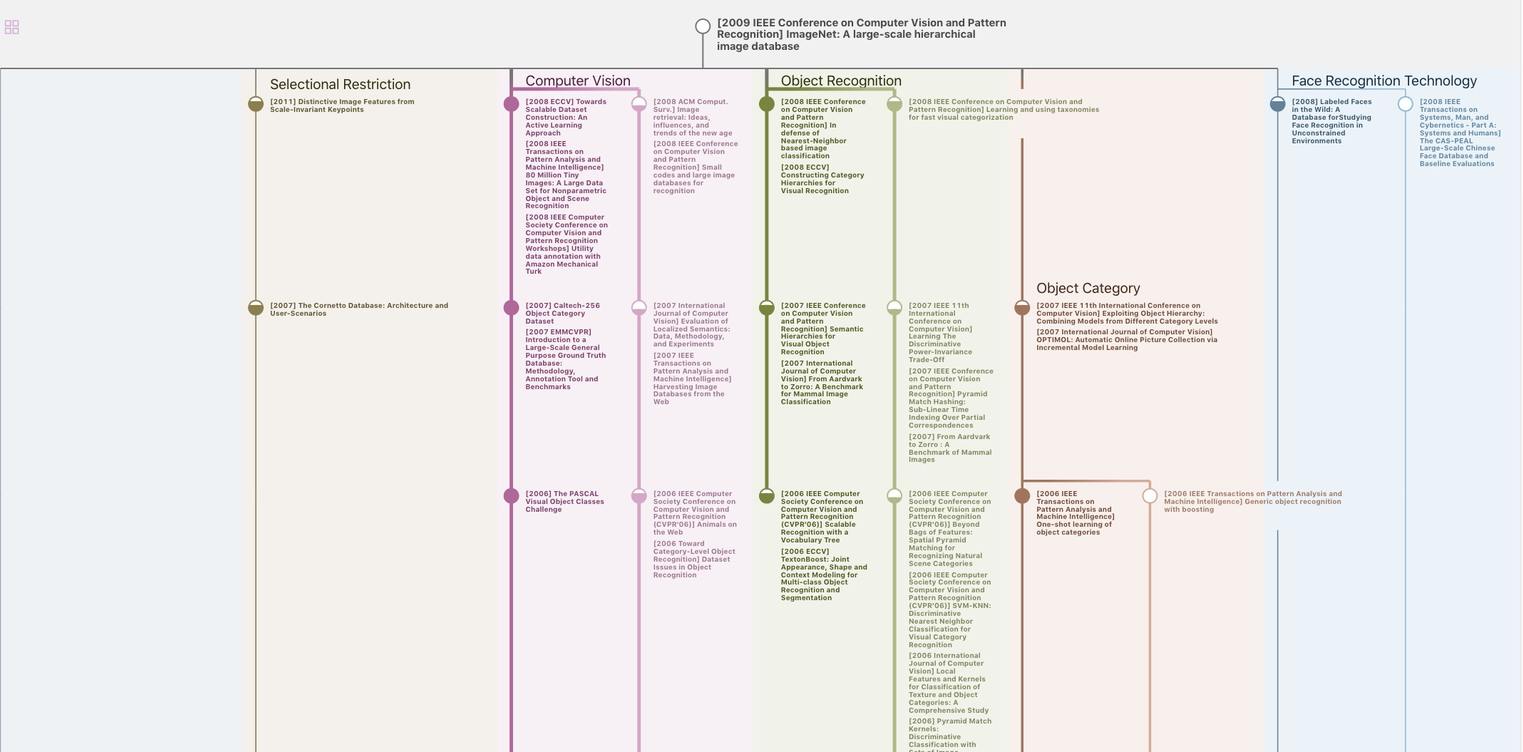
Generate MRT to find the research sequence of this paper
Chat Paper
Summary is being generated by the instructions you defined