Generalized Empirical Depth-based Classification
Research Square (Research Square)(2022)
摘要
Abstract The empirical likelihood is a fully data-dependent method which inherits many advantages of the parametric likelihood approach and requires comparatively less assumptions. In this paper, we consider empirical likelihood-based depth notion the so-called generalized empirical depths to introduce a nonparametric classification procedure. The existing classifiers based on data depths such as the halfspace and the zonoid depth suffer from the “outsiders”. The proposed method suitably considers those points to include in the convex hull of each class observations and completely gets rid of the “outsider problem”. Moreover, unlike other empirical likelihood-based procedures, it has advantage of having non-degenerate population version. The performance of the proposed approach is examined using simulated as well as real datasets, and the results are compared with those based on the zonoid and halfspace depths. In most of the cases, the proposed classifier performs comparably with aforementioned procedures across a range of settings and outperforms in many cases with encouraging results.
更多查看译文
关键词
classification,generalized,depth-based
AI 理解论文
溯源树
样例
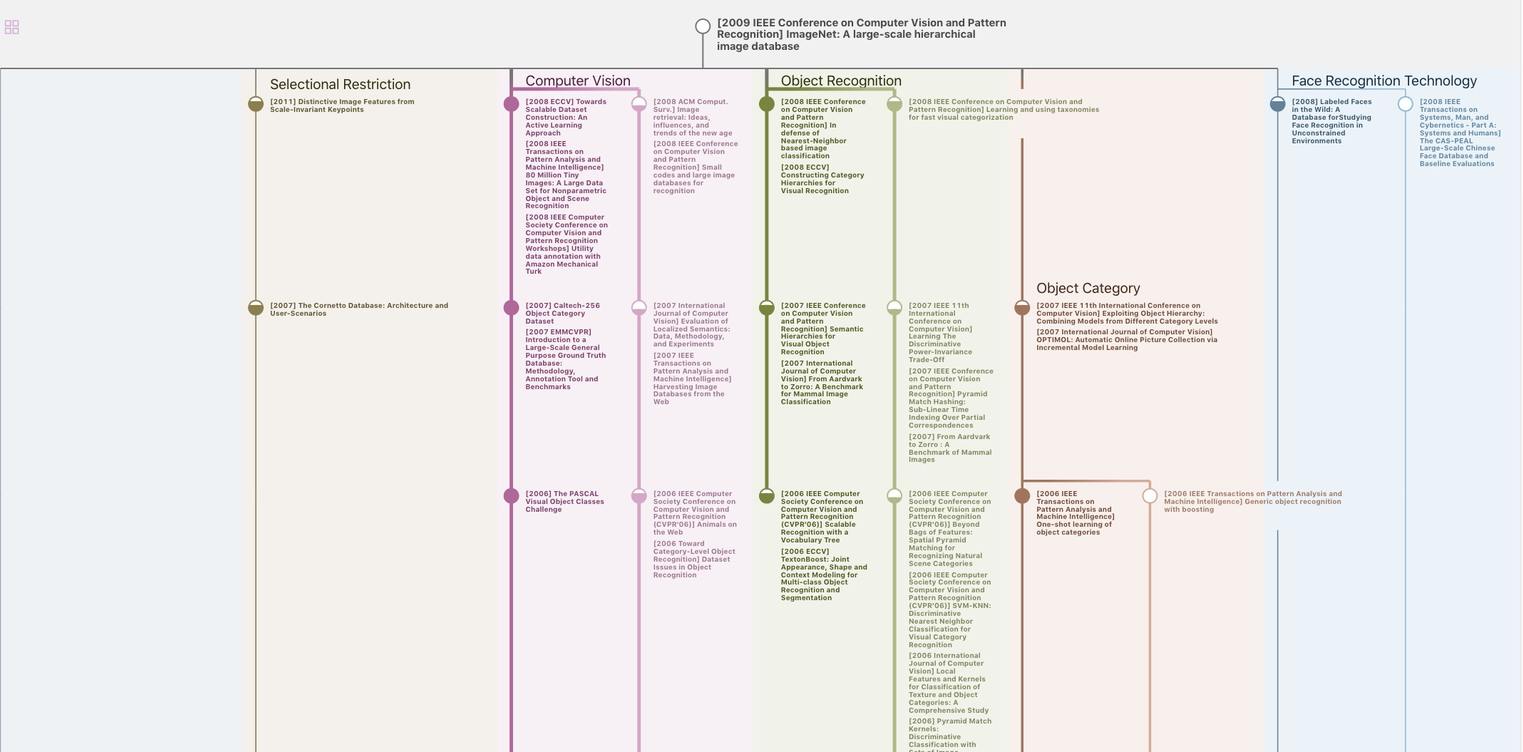
生成溯源树,研究论文发展脉络
Chat Paper
正在生成论文摘要