Robust epileptic seizure prediction with missing values using an improved denoising adversarial autoencoder
2022 4th International Conference on Image, Video and Signal Processing(2022)
摘要
Epileptic seizure prediction is one of the most used therapeutic adjuvant strategies for drug-resistant epilepsy. Conventional methods commonly perform the forecast using high-quality EEG signals with complete channels. However, in real life, missing values are often observed in EEG recordings due to the electrode falling off. In this work, a robust model is proposed to tackle this issue. By developing a denoising layer, this model attempts to learn the invariant features that are adaptive to corrupted data. To optimize the feature extraction procedure, this work improved the denoising adversarial autoencoder (DAAE) using convolutional layers. Besides, to measure the feasibility of its application, this work mimics the corrupted signals under the channel-missing situation and tests the model under this condition. Experimental results on the public CHB-MIT scalp EEG database demonstrate that this approach is better than other methods in robustness, meanwhile maintaining high accuracy.
更多查看译文
AI 理解论文
溯源树
样例
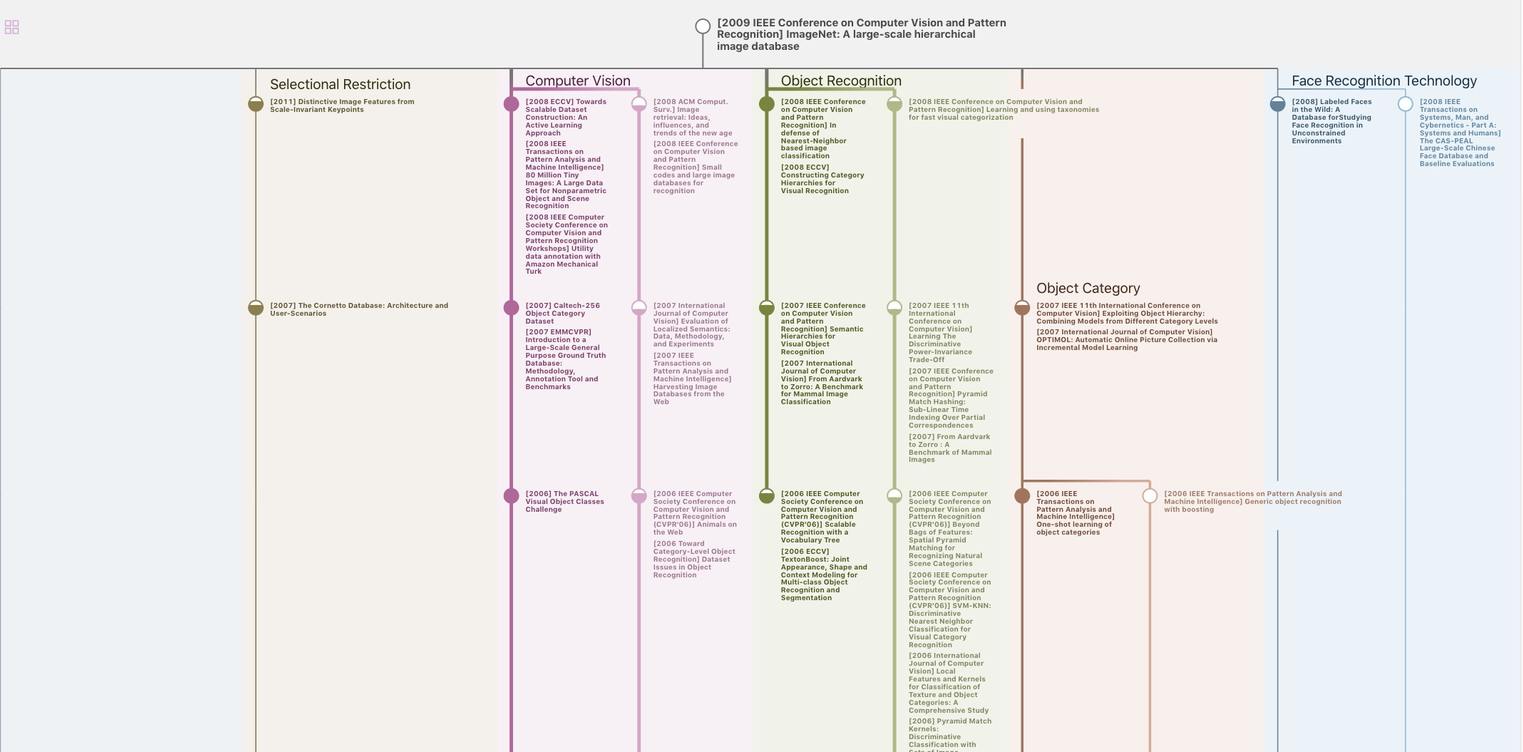
生成溯源树,研究论文发展脉络
Chat Paper
正在生成论文摘要