Heterogeneous graph neural network for lncRNA-disease association prediction
Research Square (Research Square)(2022)
摘要
Abstract The computational methods of lncRNA-disease association prediction are effective ways to solve the problem of expensive and time-consuming traditional biological experiments. However, existing methods still have challenges to make full use of network topology information to identify potential associations between lncRNA and disease in multi-source data. In this study, we propose a novel method called HGNNLDA for lncRNA-disease association prediction. First, HGNNLDA constructs a heterogeneous network composed of lncRNA similarity network, lncRNA-disease association network and lncRNA-miRNA association network; Then, on this heterogeneous network, various types of strong correlation neighbors with fixed size are sampled for each node by restart random walk; Next, the embedding information of lncRNA and disease in each lncRNA-disease association pair is obtained by the method of type-based neighbor aggregation and all types combination though heterogeneous graph neural network, in which attention mechanism is introduced considering that different types of neighbors will make different contributions to the prediction of lncRNA-disease association. As a result, the area under the receiver operating characteristic curve(AUC) and the area under the precision-recall curve(AUPR) under 5-fold cross-validation (5FCV) are 0.9786 and 0.8891, respectively. Compared with five state-of-art prediction models, HGNNLDA has better prediction performance. In addition, in two types of case studies, it is further verified that our method can effectively to predict the potential lncRNA-disease associations, and have ability to predict new diseases without any known lncRNAs.
更多查看译文
关键词
heterogeneous graph,neural network,lncrna-disease
AI 理解论文
溯源树
样例
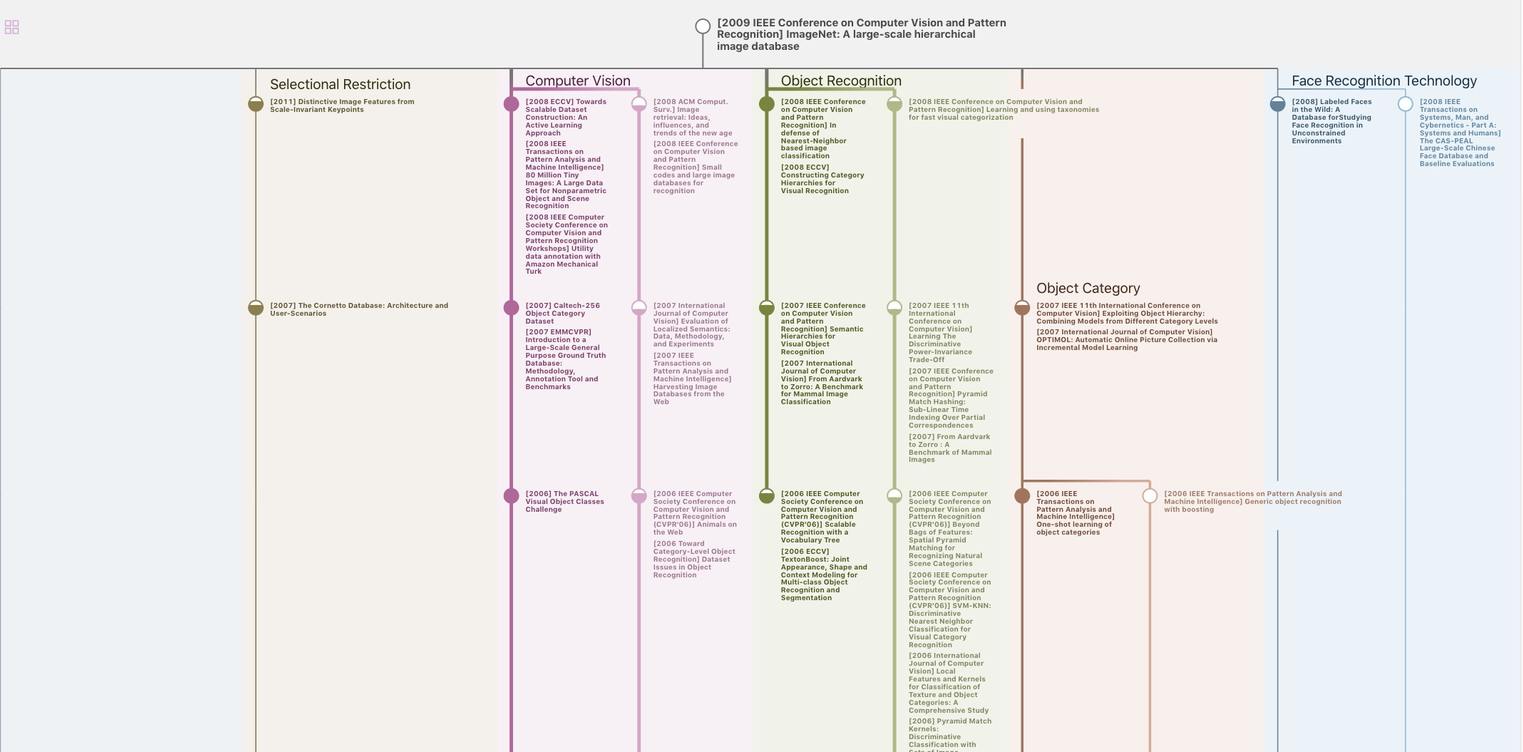
生成溯源树,研究论文发展脉络
Chat Paper
正在生成论文摘要