Identifying groundwater contaminant sources based on the hybrid gradient grey wolf algorithm and deep belief neural network
Research Square (Research Square)(2022)
摘要
Abstract Optimization models used to identify groundwater contamination sources (GCSs) often have many variables to determine and a high degree of nonlinearity. When the grey wolf optimization algorithm (GWO) is used to solve this kind of optimization model, due to the relatively weak local search ability, it has the disadvantage of premature convergence. To improve the GWO, the gradient descent algorithm (GDA) and the GWO were integrated to construct a hybrid gradient GWO (HGWO) with little dependence on the initial value and a strong local search ability. The HGWO was then applied to solve the optimization model without falling into premature convergence, which improved the accuracy of the GCS identification results. In addition, the calculation load generated was reduced by calling the simulation model, and computing time was saved in the process of solving the optimization model. A surrogate model of the numerical simulation model was established by applying a deep belief neural network (DBNN) to participate in the iterative calculation. The results show that compared with the simulated annealing algorithm (SA) and GWO, the HGWO had higher calculation accuracy and could improve the identification accuracy of GCSs. Although the calculation efficiency of the HGWO was insufficient, the improvement in solution accuracy was sufficient to overcome this shortcoming. The DBNN surrogate model had high accuracy and could save 99% of the computing time by participating in the iterative calculation instead of the simulation model.
更多查看译文
关键词
groundwater contaminant sources,neural network,deep belief
AI 理解论文
溯源树
样例
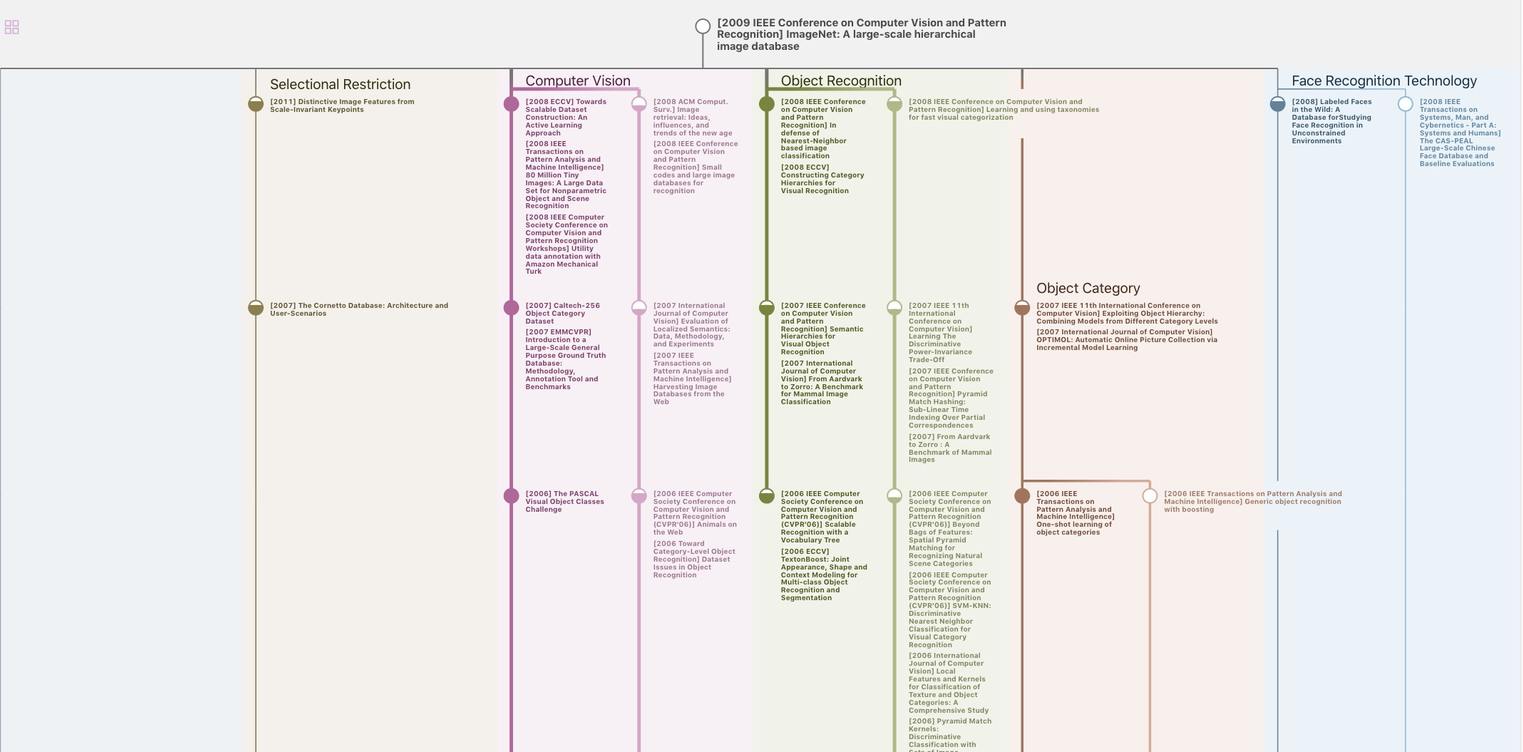
生成溯源树,研究论文发展脉络
Chat Paper
正在生成论文摘要