Optimization of Neoadjuvant Chemotherapy for Rectal Cancer Using the Deep Learning Approach
Research Square (Research Square)(2022)
摘要
Abstract To optimize the use of neoadjuvant chemotherapy (NAC), this study aimed to develop a therapeutic response prediction model with NAC for rectal cancer (RC). Three courses of S-1 and oxaliplatin (SOX) NAC were administered before rectal surgery. We prospectively collected the dataset for the prechemotherapy arterial phase of enhanced computed tomography (CT) images from 57 RC patients undergoing rectal surgery after NAC. The therapeutic response to NAC had been pathologically confirmed. We established three prediction labels: poor response, marked response, and complete response (CR). We built a CT patch-based predictive model using a residual convolutional neural network and used 3-fold cross-validation. Of the 57 patients, pathological CR was observed in 9 (15.8%). A total of 4,607 patches were extracted from the segmented tumor area of each patient. The accuracy of the model for predicting pathological CR was 99.9% for the training dataset. In the validation dataset, the accuracy was 94.9%. Furthermore, the area under the curve (AUC) was 0.949. Likewise, in the prediction of marked and poor response, the models demonstrated high accuracy (93.6% and 93.3%, respectively) and high AUC (0.966 and 0.976, respectively). Our CT-based deep learning model exhibited high predictive performance in projecting therapeutic response to SOX NAC.
更多查看译文
关键词
rectal cancer,neoadjuvant chemotherapy,deep learning
AI 理解论文
溯源树
样例
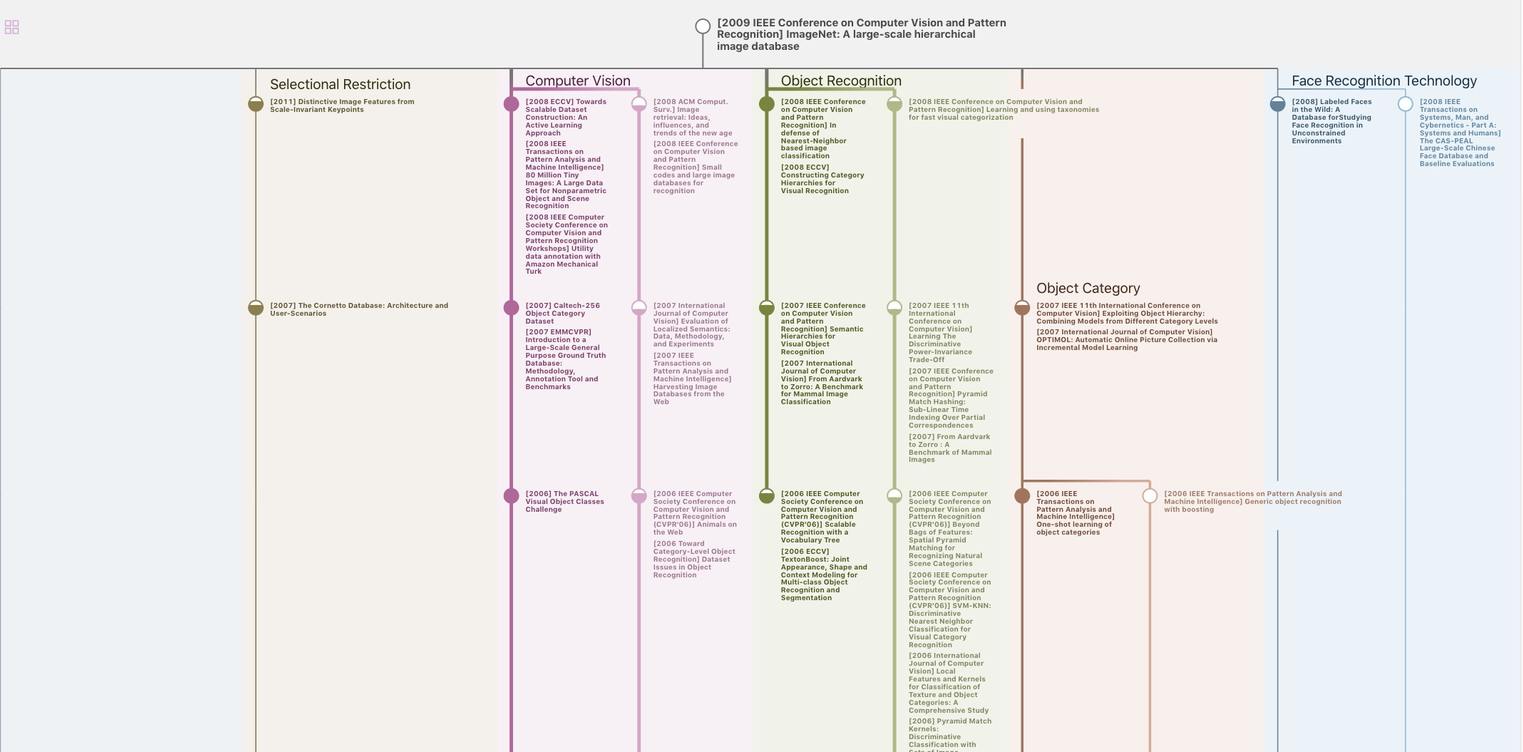
生成溯源树,研究论文发展脉络
Chat Paper
正在生成论文摘要