Early detection of tongue cancer using a convolutional neural network and evaluation of the effectiveness of EfficientNet
Research Square (Research Square)(2022)
摘要
Abstract ObjectivesEarly detection of oral cancer is critical because the survival rate is reported to be 90%, whereas 45.5% for advanced oral cancer occur with neck metastasis. This study assessed the detection potential of oral tongue cancer and precancerous lesions using a convolutional neural network (CNN) and evaluated the effectiveness of EfficientNet in a situation with limited datasets.Materials and Methods1,810 tongue images organized into four categories (malignant tumors, precancerous lesions, benign or inflammatory lesions, and normal) were used for model training. Data augmentation, transfer learning, and fine-tuning have been used to overcome the problems associated with limited datasets. Also, the weight balancing method was introduced to mitigate class imbalance by assigning different weights to each class. VGG16, Inception-ResNet-V2, and EfficientNet models were used and compared to each other.ResultsThis study evaluated the possibility of detecting tongue cancer and precancerous lesions using CNN. The final model achieved an accuracy of 0.9167, a precision of 0.9212, a recall of 0.9167, and an F2 score of 0.9176 with the test dataset.ConclusionsOur proposed model can detect potential oral cancer and precancerous lesions. EfficientNet was effective through data augmentation and weight balancing in detecting the tongue lesions with a limited dataset. The results of this study may help patients and general practitioners in tongue lesion diagnosis.Clinical relevanceIn many cases, tongue cancer is initially confused with glossitis, even by general practitioners. Thus, screening of tongue cancer/precancerous lesions through an artificial neural network will help detect tongue cancer early.
更多查看译文
关键词
tongue cancer,convolutional neural network,efficientnet,neural network,early detection
AI 理解论文
溯源树
样例
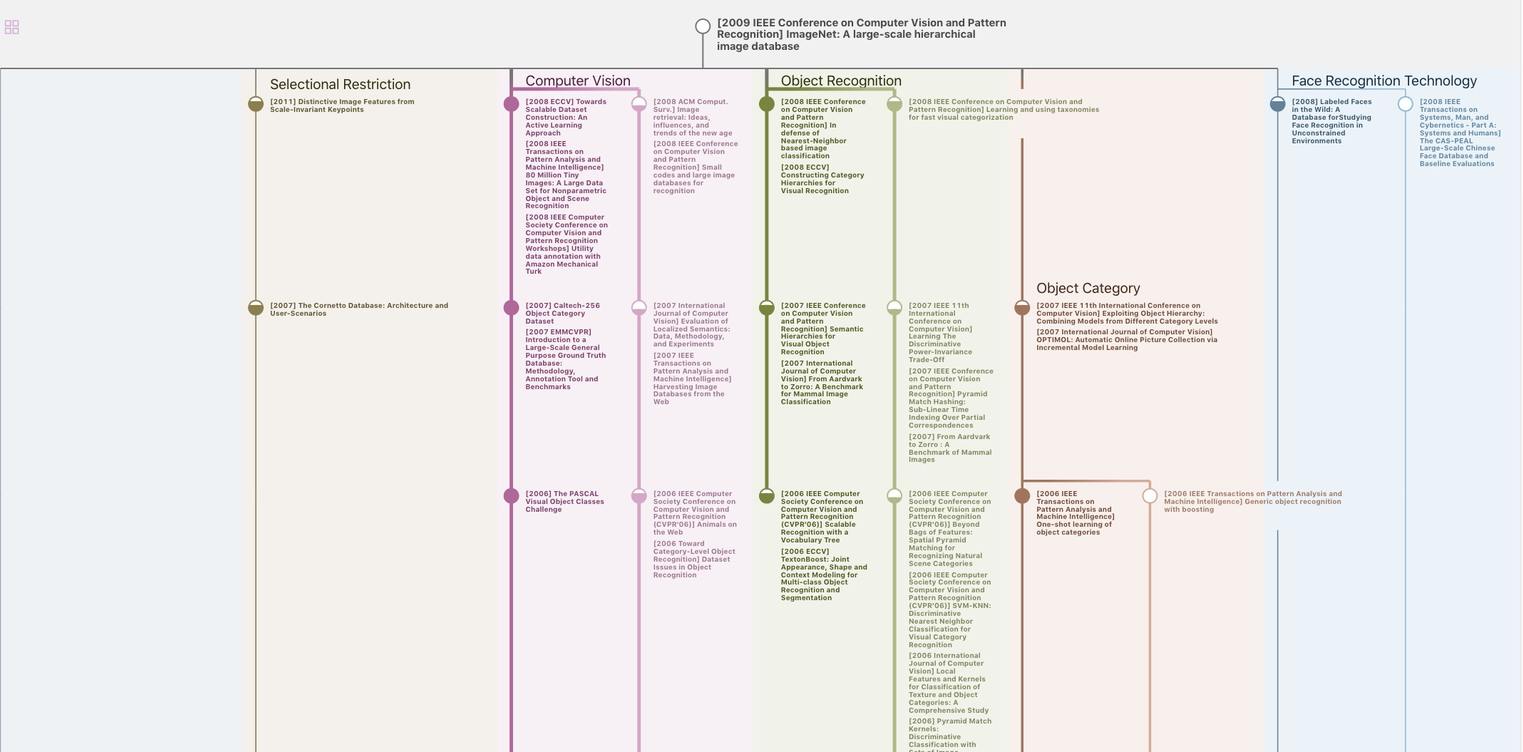
生成溯源树,研究论文发展脉络
Chat Paper
正在生成论文摘要