Perioperative risk assessment of patients using the ‘MyRISK’ digital score completed before the preanesthetic consultation: a prospective, observational trial. (Preprint)
crossref(2022)
摘要
BACKGROUND The pandemic highlighted the potential of digital health solutions to adapt the organization of care in a crisis context. OBJECTIVE Our aim was to demonstrate the prognostic value of the perioperative ‘MyRISK score’ derived from data collected on a digital conversational agent (chatbot) before the preanesthetic consultation (PAC). METHODS Single-center, prospective, observational study. The 16 items composing the MyRISK score were selected by Delphi method. An algorithm was used to stratify low (‘green’), intermediate (‘orange’) and high (‘red’) risk patients. Postoperative complications occurring in the first 6 months (composite criterion) were numerically collected and verified by phone and consultation of the electronic medical database. A logistic regression was carried out to identify their explanatory variables. A machine learning model was trained to predict the MyRISK score using a dataset of 1823 ‘green’ and ‘red’ patients to re-classify ‘orange’ individuals. User satisfaction and usability were assessed. RESULTS Four hundered and one patients were included. Sixteen of the 389 patients (4.1%) analyzed for the primary endpoint experienced a postoperative complication. An ASA score ≥ 3 and a ‘red’ score were independent predictors of postoperative complications (Odds Ratios of 5.8 [CI95%: 1.7 - 20.2; p=0.006] and 5.9 [CI95%: 1.5 - 22.3; p=0.009] respectively). Once ‘orange’ patients re-classified according to the prediction of the trained model, a ‘red’ score was identified as a strong predictor of postoperative complications with an Odds Ratio of 21.8 [CI95%: 2.8 - 171.5; p=0.003]. Patient satisfaction Numeric Rating Scale and System Usability Scale were 8 [7-9]/10 and 90 [82.5-95]/100 respectively. CONCLUSIONS We demonstrate the good prognostic predictive value of the MyRISK digital perioperative risk score established before the PAC. Its predictive strength was increase using a machine learning model reclassifying intermediate-risk patient. This numerical categorization could be used to guide patients between teleconsultation and face-to-face PAC, or to provide a perioperative personalized care pathway for high-risk patients.
更多查看译文
AI 理解论文
溯源树
样例
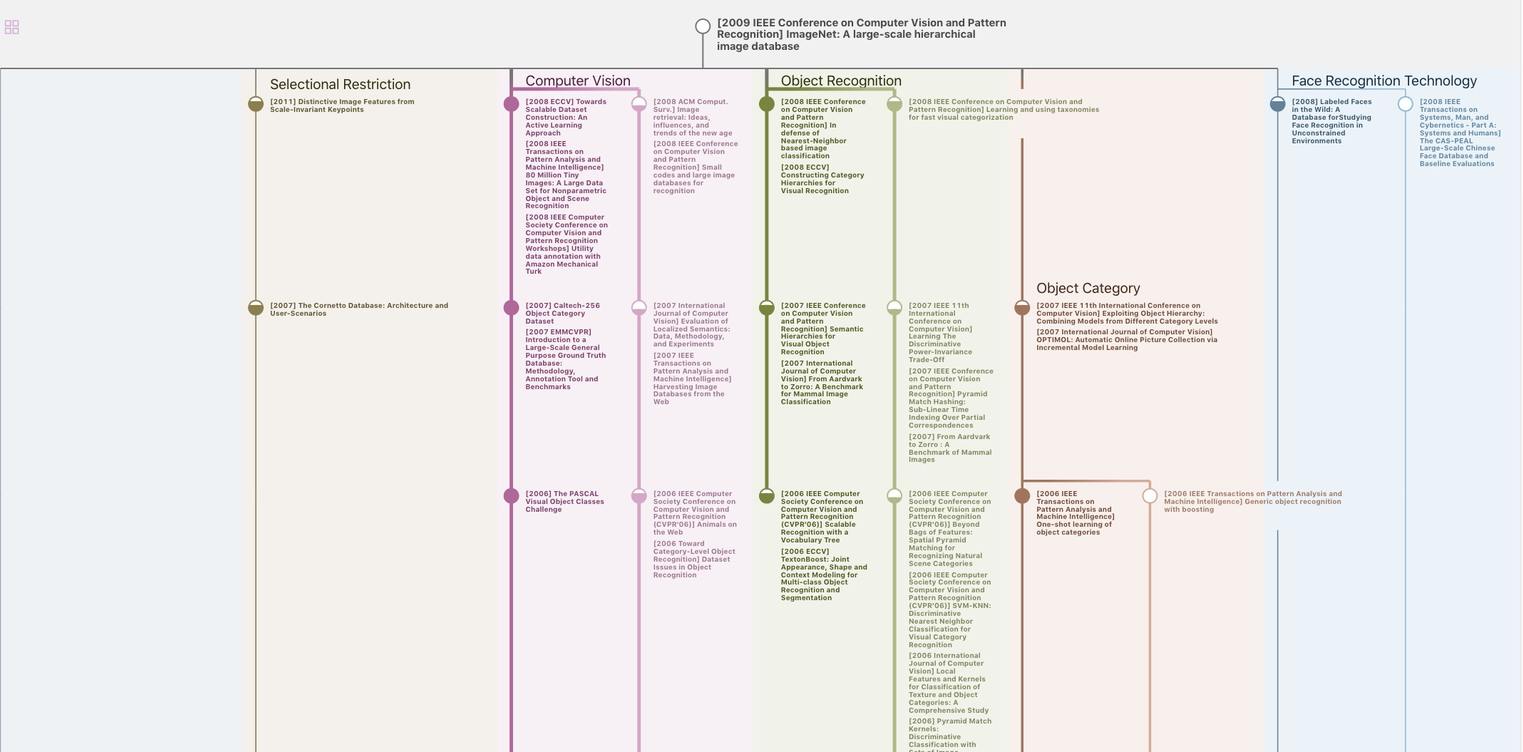
生成溯源树,研究论文发展脉络
Chat Paper
正在生成论文摘要