Calibrating the Performance Assessment Mechanism in Virtual Laboratories with Machine Learning: Using a Non-Hierarchical and a Hierarchical Variation
crossref(2022)
摘要
Abstract Science universities are constantly confronted with the challenge of offering efficient lab training to their students while securing their safety and protecting the lab equipment from damages; hence the needs for the development of distance learnings tool for students to be trained virtually and safely in using the various lab instruments and performing experiments. Furthermore, as the students are evaluated for their laboratory performance at the on-site labs, the need for their assessment at the virtual labs is also present; hence the need for an incorporated assessment mechanism in the virtual lab in charge of the evaluation of the user’s performance. For the assessment mechanism to be reliable though and devoid of the designer’s bias, it may need calibration with machine learning. Hellenic Open University has developed its own virtual biology laboratory, Onlabs, which simulates its on-site one for its students to be trained and evaluated at. Considering the evaluation of the user’s performance in Onlabs, it is made with respect to particular experiments and is based on an embedded scoring algorithm. The latter has also been optimized with the use of two separate machine learning methods, particularly a genetic algorithm and an artificial neural network. Moreover, within the context of the experimental procedure of microscoping, the scoring algorithm was redesigned in a hierarchical fashion in order to incorporate background knowledge about the procedure. In this paper, we present the two machine learning methods used for the optimization of the assessment mechanism in a non-hierarchical and a hierarchical variation and compare the results within a particular variation as well as within a particular method.
更多查看译文
AI 理解论文
溯源树
样例
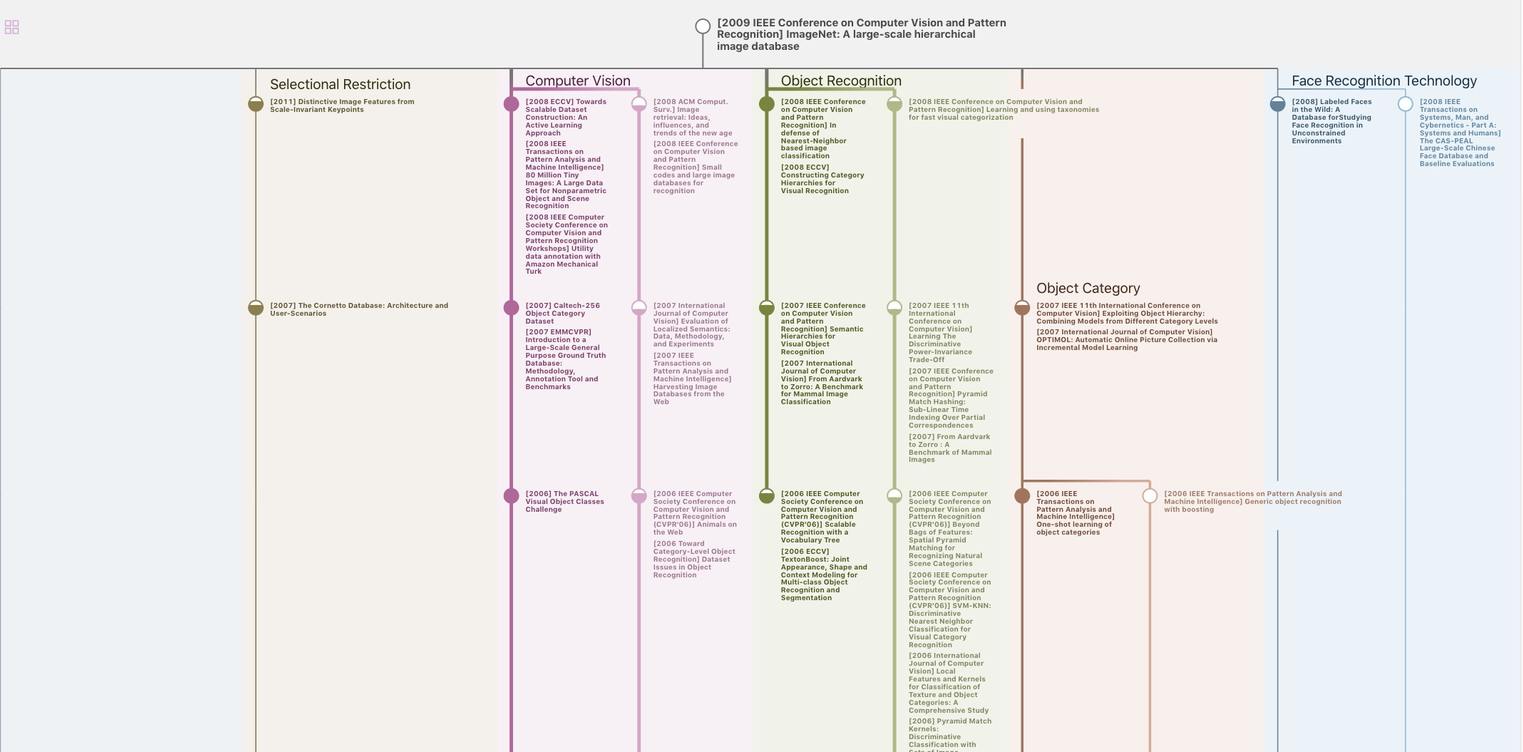
生成溯源树,研究论文发展脉络
Chat Paper
正在生成论文摘要