GAP method: a gap-based technique for outlier detection and its application to wildlife GPS data
Research Square (Research Square)(2022)
摘要
Abstract Outliers in datasets are a concern for analysts as noise or signals. To detect them, we must quantify how much each data point lies away from others and then set a threshold to separate outliers from the others. However, there is scant research on the latter. We developed a method to address this issue. The method uses value gaps between adjacent data pairs in a univariate dataset, where data are in ascending order of values. Its core process is to find the largest gap in the upper range of the dataset and remove the data above the gap as outliers, and its supplementary process is to repeat the core process with the same dataset after removal. Analysts must decide when to stop this repetition; however, this technique lessens subjectivity by leaving only a few logical options. We can apply this method to any dataset, such as a time series or multivariate dataset, by quantifying data to expose anomalies as outliers in a univariate dataset. We demonstrate how to implement the technique using wildlife GPS data. We expect that this method has a much broader application because, to our knowledge, there are no other techniques to play the role.
更多查看译文
关键词
outlier detection,wildlife,gps,gap-based
AI 理解论文
溯源树
样例
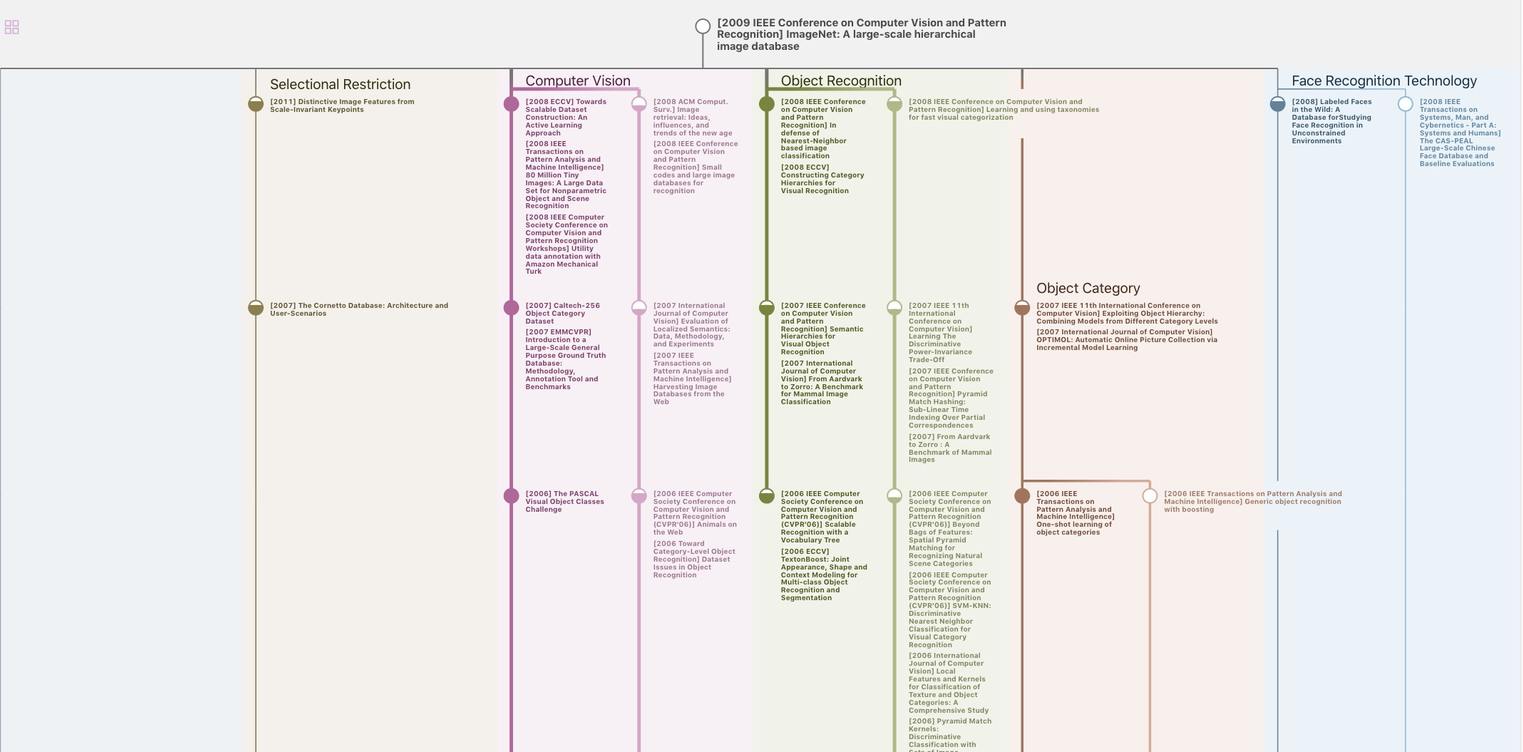
生成溯源树,研究论文发展脉络
Chat Paper
正在生成论文摘要