Anomaly Prediction of CT Equipment Based on IoMT Data
Research Square (Research Square)(2022)
摘要
Abstract Large-scale medical equipment, extensively implemented in medical services, is of vital importance for diagnosis but vulnerable to various failures. Most of hospitals conduct regular maintenance for their medical equipment and have been suffering from medical-equipment-related incidents for years. Currently, the Internet of Medical Things (IoMT) has emerged as a crucial technology to monitor the real-time status of medical equipment. In this paper, we develop a IoMT system of Computed Tomography (CT) equipment in the West China Hospital of Sichuan University and meanwhile, select machine-learning algorithms to effectively store, preprocess and analyze the data. Specifically, a data-driven framework is proposed to predict the anomalies of CT equipment successfully. In this framework, the first step is data preprocessing, where we average the original non-uniform data and use linear interpolation to handle missing data. The second step is feature construction, where sliding time window is applied to fully reflect historical information. The third step is features selection, where the seven features that perform best are selected. Finally, we use two methods to split the training and test set, and apply random oversampling to deal with those imbalanced data before putting them into models. The results show that the prediction precision and recall of our method are 70% and 81%, respectively. The proposed method could distinguish the state of CT equipment and be used as a reference for practical maintenance by early prediction, where unexpected anomalies of medical equipment could be reduced. It also brings new insights about how to handle non-uniform and unbalanced time series data in the practical cases.
更多查看译文
关键词
anomaly prediction,prediction equipment
AI 理解论文
溯源树
样例
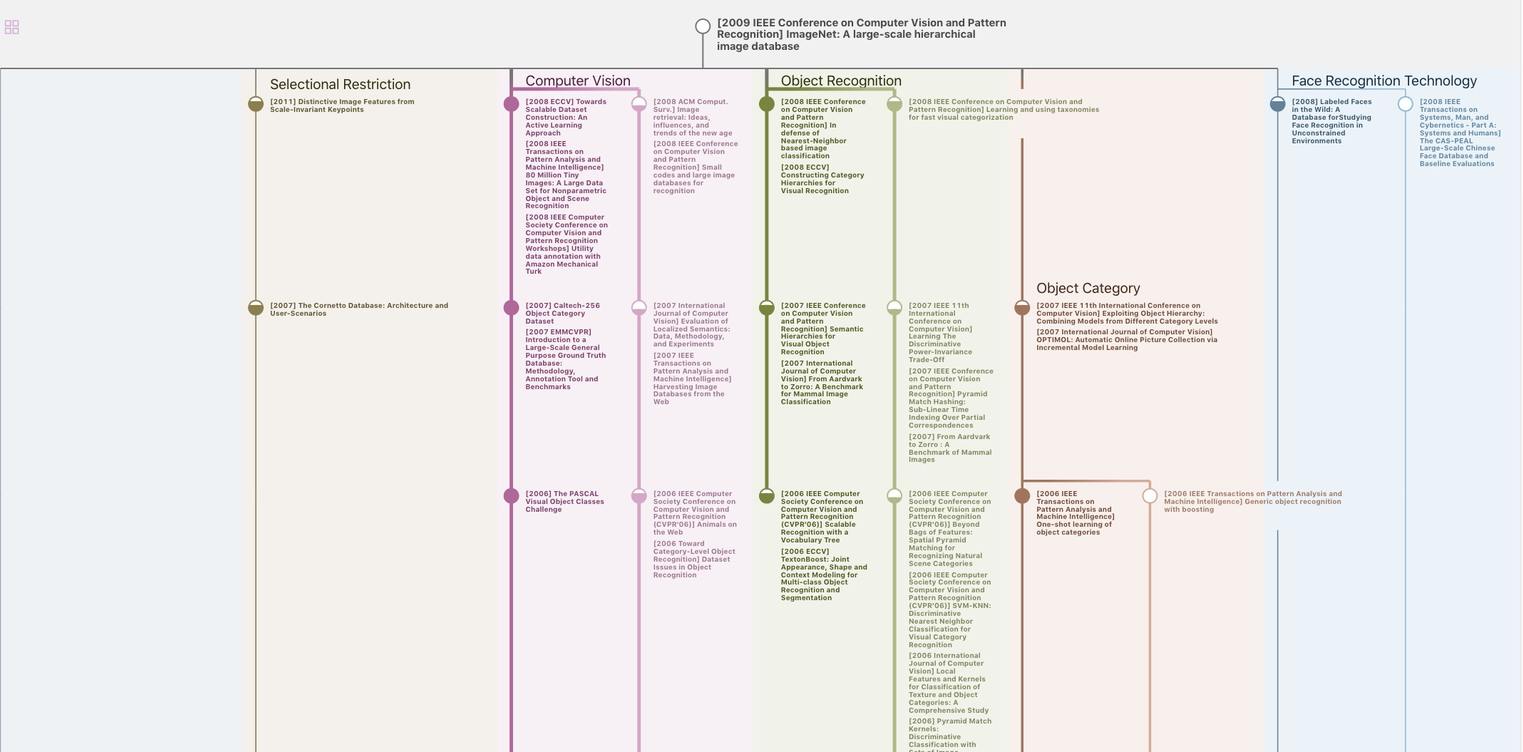
生成溯源树,研究论文发展脉络
Chat Paper
正在生成论文摘要