What predicts people’s adherence to COVID-19 misinformation? A retrospective study using a nationwide online survey among adults residing in the United States
Research Square (Research Square)(2022)
摘要
Abstract Background: Tackling infodemics with flooding misinformation is key to managing the COVID-19 pandemic. Yet only a few studies have attempted to understand the characteristics of the people who believe in and adhere to misinformation. Methods: Data was used from an online survey that was administered in April 2020 to 6,518 English-speaking adult participants in the United States. We created binary variables to represent four misinformation categories related to COVID-19: general COVID-19-related, vaccine/anti-vaccine, COVID-19 as an act of bioterrorism, and mode of transmission. Using binary logistic regression and the LASSO regularization, we then identified the important predictors of adherence to each type of misinformation. Nested vector bootstrapping approach was used to estimate the standard error of the LASSO coefficients. Results: About 30% of our sample reported adhering to at least one type of COVID-19-related misinformation. Adherence to one type of misinformation was not strongly associated with adherence to other types. We also identified 58 demographic and socioeconomic factors that predicted people’s susceptibility to at least one type of COVID-19 misinformation. Different groups, characterized by distinct sets of predictors, were susceptible to different types of misinformation. There were 25 predictors for general COVID-19 misinformation, 42 for COVID-19 vaccine, 36 for COVID-19 as an act of bioterrorism, and 27 for mode of COVID-transmission. Conclusion: Our findings confirm the existence of groups with unique characteristics that adheres to different types of COVID-19 misinformation. Findings are readily applicable by policymakers to inform careful targeting of misinformation mitigation strategies.
更多查看译文
关键词
adherence,nationwide online survey
AI 理解论文
溯源树
样例
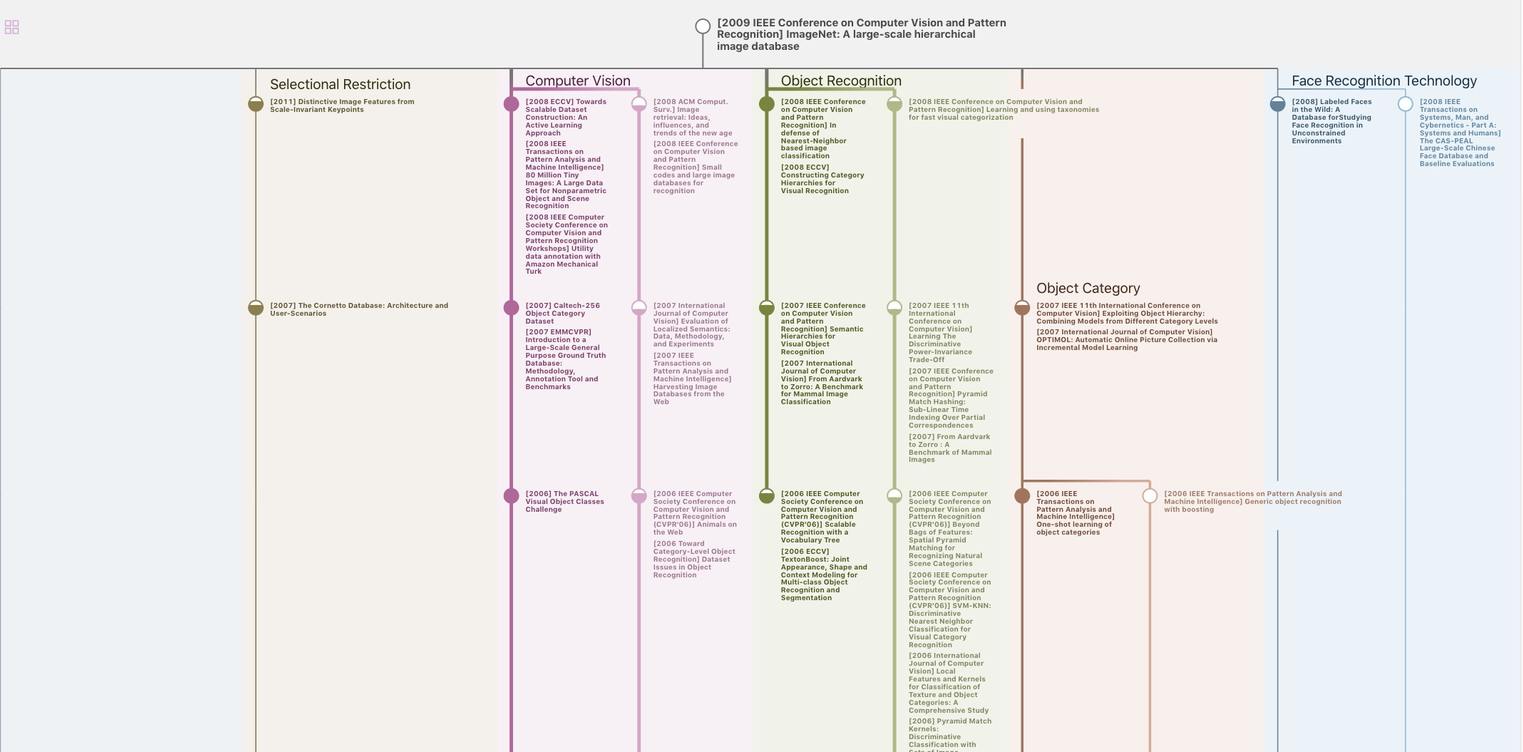
生成溯源树,研究论文发展脉络
Chat Paper
正在生成论文摘要