Comparing Contact Tracing Through Bluetooth and GPS Surveillance Data: Method (Preprint)
crossref(2022)
摘要
BACKGROUND The COVID-19 pandemic has highlighted the need for accurate and responsive epidemiological simulations of epidemic outbreaks. These simulations must be grounded in quantities derived from the measurements, for example of the period over which a person is infectious or the mortality rate of the disease. Particularly difficult parameters to estimate are those associated with contacts between individuals. Digital contact tracing data, such as that provided by Bluetooth beaconing or GPS co-locating, can provide more precise measures of contact than traditional methods based on direct observation or self-reporting. Both measurement modalities have shortcomings and are prone to false positives or negatives as unmeasured environmental influences bias the data. OBJECTIVE In this paper, we compare GPS co-located versus Bluetooth beacon derived proximity contact data for their impacts on transmission models’ results, under community and types of diseases. METHODS We examine the contact patterns derived from three previously collected datasets employing both Bluetooth beaconing and GPS localization on smartphones running the Ethica Data app. We compared the structure of contact networks inferred from proximity contact data collected with modalities of GPS co-locating and Bluetooth beaconing. We assessed the impact of sensing modalities on simulation results of transmission models informed by proximate contacts derived from sensing data. Specifically, we compared the incidence number, attack rate, and individual infection risks across simulation results of agent-based Susceptible, Exposed, Infectious, Recovered (SEIR) transmission models of four different contagious diseases. We demonstrate their differences with violin plots, t-tests, and Kullback-Leibler (KL) divergence. RESULTS Both the network structure analyses show visually salient differences between GPS co-locating and Bluetooth beaconing collected proximity contact data, regardless of the underlying population. The violin plots of attack rate and KL-divergence of individual infection risks demonstrate discernible differences for different sensing modalities regardless of the underlying population and diseases. The results of t-tests on attack rate between different sensing modalities are mostly significant, with P-value less than 0.001. CONCLUSIONS We show that the contact networks generated from these two measurement modalities are different and generate significantly different attack rates across multiple datasets and pathogens. While both modalities offer higher resolution portraits of contact behavior than is possible with most traditional contact measures, the differential impact of measurement modality on simulation outcome cannot be ignored.
更多查看译文
AI 理解论文
溯源树
样例
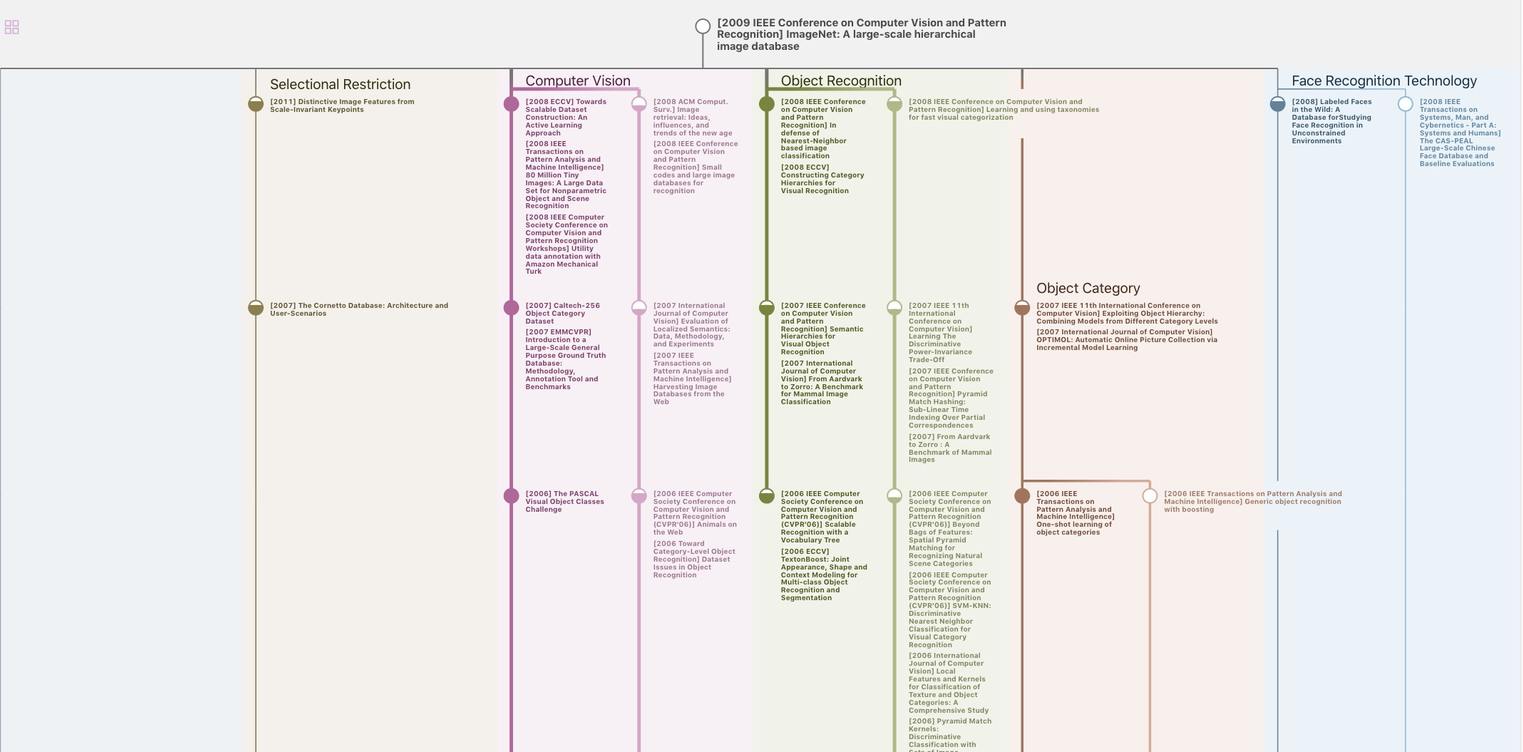
生成溯源树,研究论文发展脉络
Chat Paper
正在生成论文摘要