Applying machine learning methods to river topography prediction under different data abundances
crossref(2022)
摘要
Fast and accurate prediction of river topography is very important for river management and channel regulation. In river simulation, river topography prediction is usually performed using either physical or numerical models, but the former is labor-intensive and the latter suffers from computational efficiency problems. In recent years, machine learning has introduced new insights into many fields, and with the upgrading and development of emerging topographic measurement technologies, high-frequency river topographic data acquisition has become possible, as has the introduction of machine learning algorithms in river topographic prediction problems. In this study, three machine learning models are developed for river topography prediction under four different data abundance conditions, and the prediction results of different models are evaluated. The results show that the effect of data abundance on prediction accuracy is much greater than that of machine learning models in the river topography prediction problem, and all three machine learning models can achieve satisfactory accuracy when the data abundance is sufficient. The prediction accuracy and robustness increase with the complexity of machine learning models. As the data abundance decreases, the prediction accuracy of the machine learning models gradually decreases, and the prediction accuracy of the more complex machine learning models gradually approaches or is even lower than that of the less complex machine learning models. The model prediction error contours also show that the errors are spatially correlated and distributed in strips along the river flow direction. This work improves understanding of the effect of data abundance and model selection on river topography prediction problems. It also contributes to the introduction of machine learning models into river simulation as well as the advancement of river bathymetry equipment and techniques.
更多查看译文
AI 理解论文
溯源树
样例
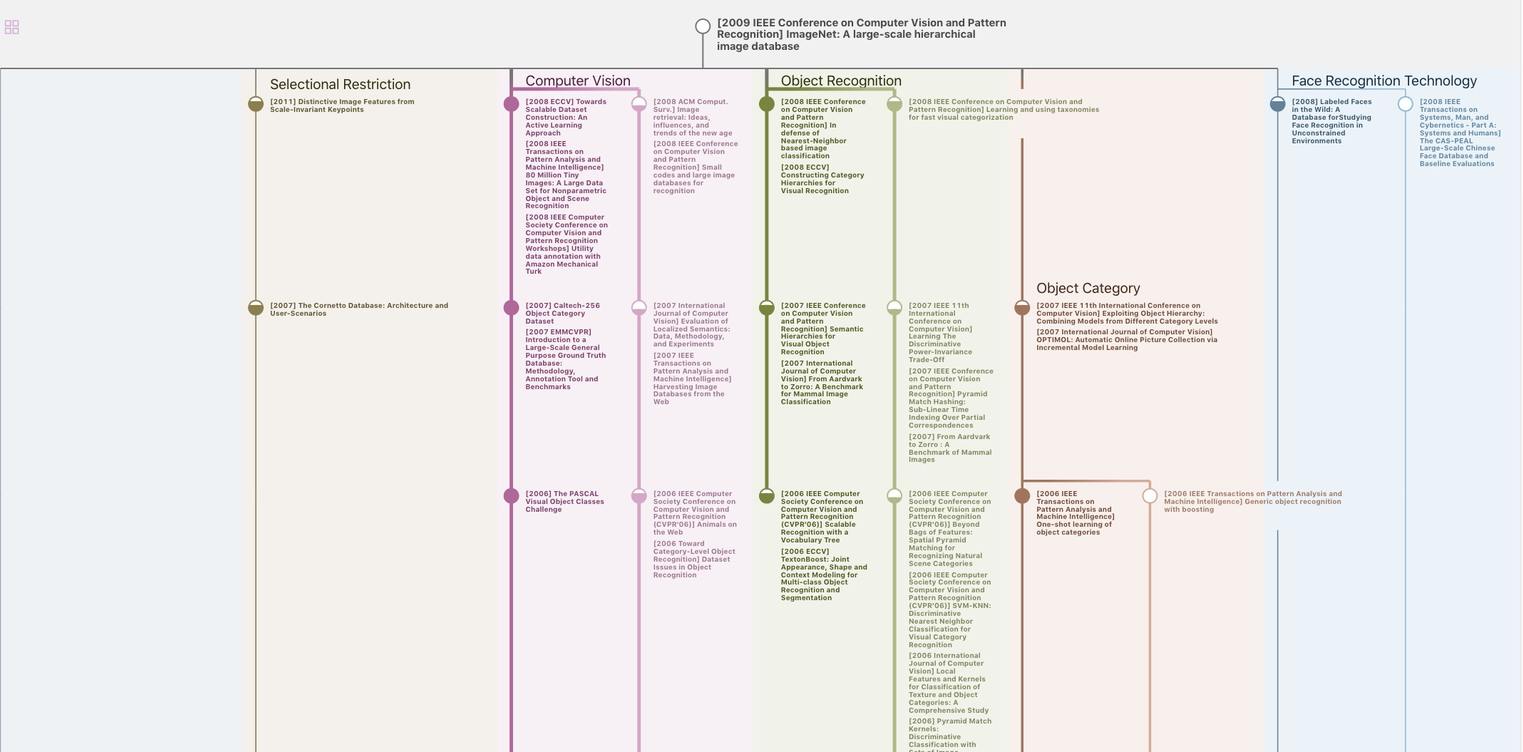
生成溯源树,研究论文发展脉络
Chat Paper
正在生成论文摘要