A Bayesian Belief Network model assessing the multi-scale effects of riparian vegetation on stream invertebrates
crossref(2022)
摘要
<p>Despite the benefits of riparian vegetation, they are limitedly implemented in water management – which is partly due to the lack of information on their effectiveness. In this context, social learning is valuable to inform stakeholders of the efficacy of riparian vegetation in mitigating stream degradation. Tools used in social learning activities are of paramount importance in the learning process. We developed a Bayesian belief network (BBN) model as a learning tool to simulate and assess the reach- and segment-scale effects of riparian vegetation properties and subcatchment-scale land use on instream invertebrates. We surveyed reach-scale riparian conditions, extracted segment-scale riparian and land use information from geographic information system (GIS) data and collected macroinvertebrate samples from four catchments in Europe (Belgium, Norway, Romania and Sweden). We modelled the ecological water quality, expressed as Average Score Per Taxon, as a function of different riparian variables using the BBN modelling approach. The collected data were used to populate the conditional probability table of the BBN model. The model simulations provided insights into the usefulness of both reach- and segment-scale riparian vegetation attributes in enhancing ecological water quality. We assessed the strengths and limitations of the BBN model for application as a learning tool. Despite some weaknesses, the BBN model has great potential in workshop activities to stimulate key learning processes that help inform the management of riparian zones.</p>
更多查看译文
AI 理解论文
溯源树
样例
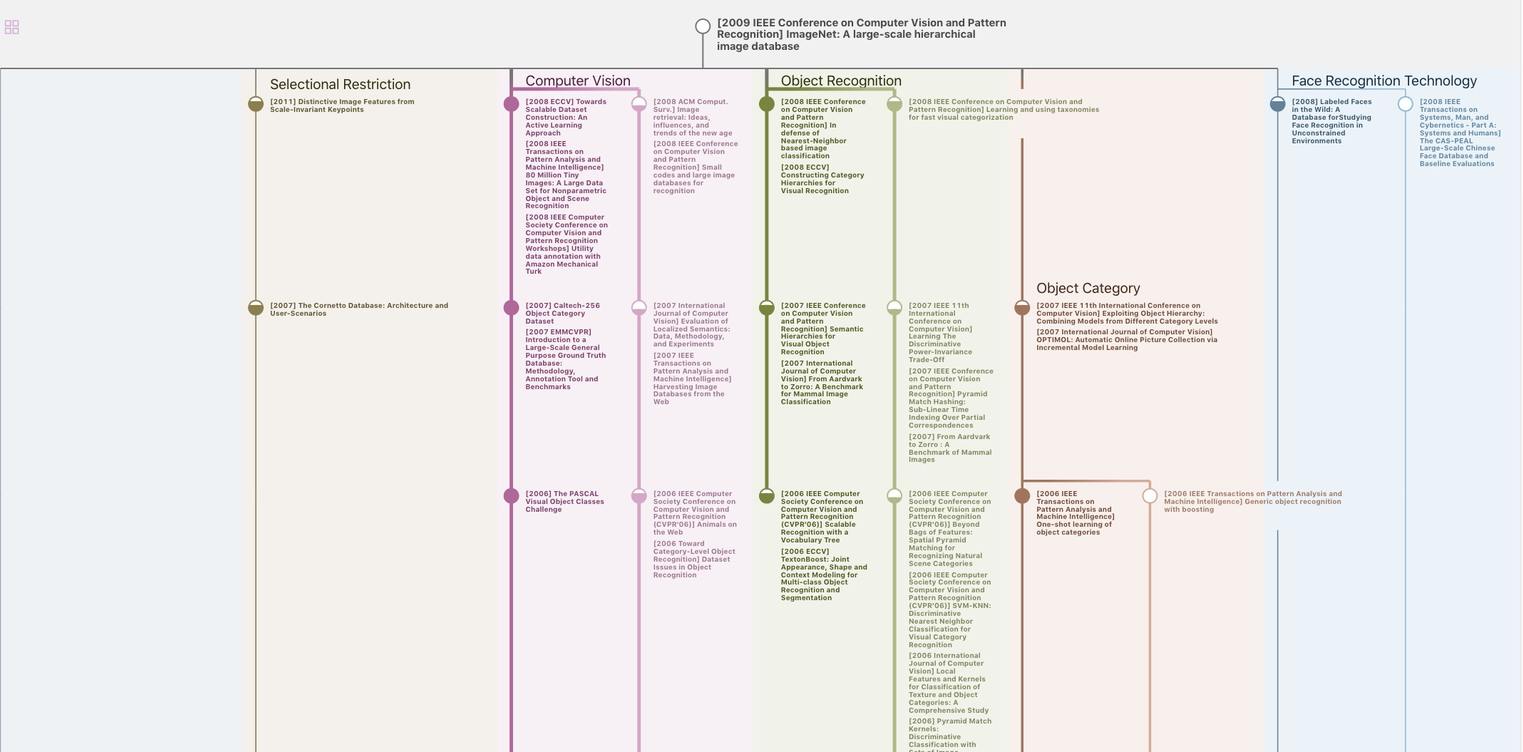
生成溯源树,研究论文发展脉络
Chat Paper
正在生成论文摘要