Identifying the geographic distribution of seawater desalination plants globally using species distribution models
crossref(2022)
摘要
<p>Desalination water is a vital source of freshwater for regions with coastal water scarcity. Identifying the geographic distribution of global seawater desalination plants enables a spatially detailed water sources assessment. In this study, which is the first of its kind, we investigated the potential application of species distribution models (SDMs), which are widely used in ecology, to predict the global spatial distribution of seawater desalination plants. Two regression SDMs, a generalized linear model (GLM) and a generalized additive model (GAM), along with two machine learning SDMs, a random forest (RF) model and a generalized boosted regression model (GBM), were trained and tested using the cross-validation method at 0.5 degrees. For each SDM, we considered four explanatory variables: aridity, distance to seashore, gross domestic product (GDP) per capita, and the sum of annual domestic and industrial water withdrawal. Our results showed that the four SDMs have good accuracy according to three different evaluation metrics. An ensemble presence map was then created from the four individual SDM predictions. Finally, we mapped the future distribution of seawater desalination plants. Due to the increases in aridity, GDP per capita, and domestic and industrial water withdrawal, the total number of presence grid cells is predicted to increase from 2014 figures by 37%, 47%, 35%, and 30% in 2030, 2050, 2070, and 2090, respectively. Using future predictions such as these, our study can contribute to integrated global water resources assessments. Our findings also provide insight into how SDMs can be used for predicting the geographic locations of water management facilities.</p>
更多查看译文
AI 理解论文
溯源树
样例
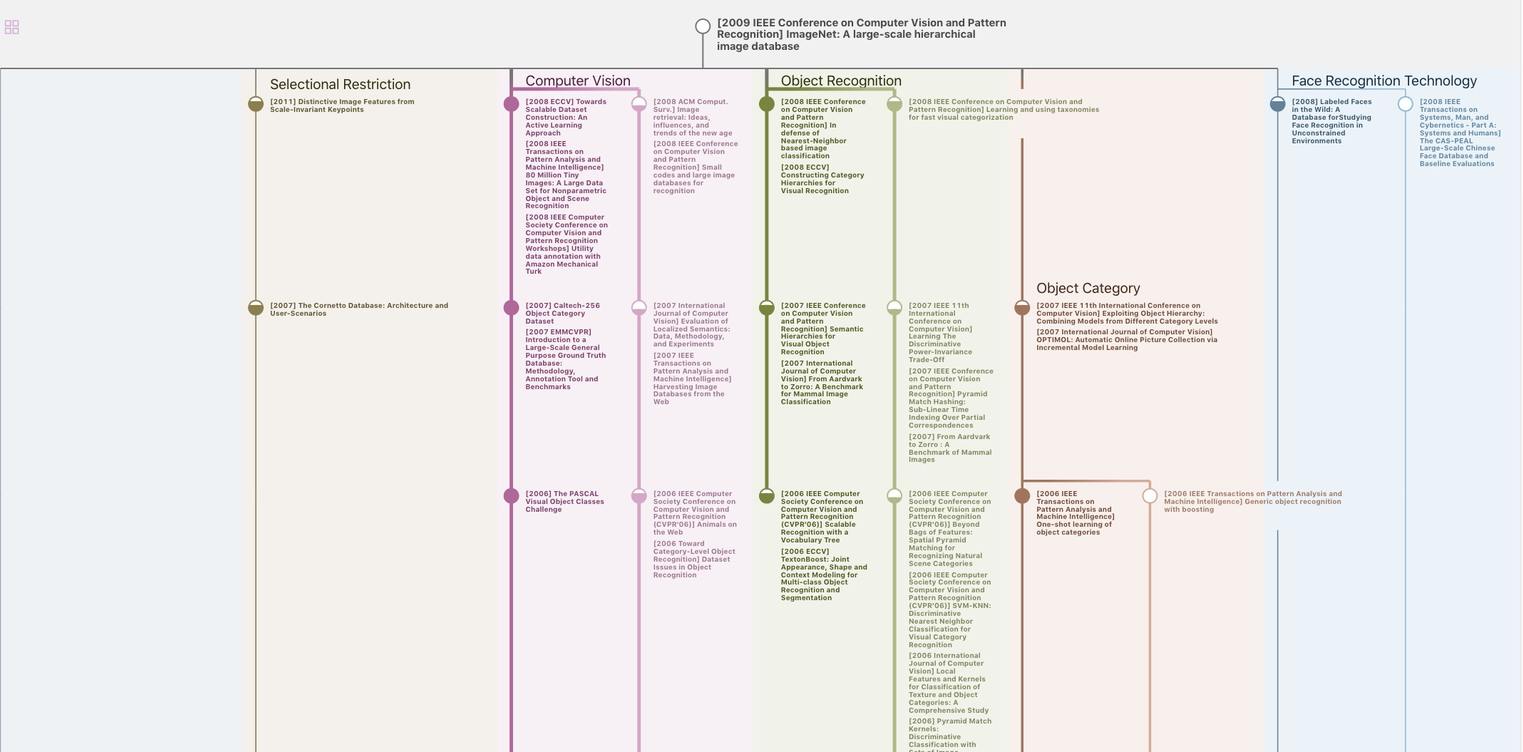
生成溯源树,研究论文发展脉络
Chat Paper
正在生成论文摘要